– Welcome everyone to Wednesday Nite at the Lab. I am Tom Zinnen. I work here at the UW-Madison Biotechnology Center. I also work for UW-Extension Cooperative Extension, and on behalf of those folks and our other core organizers, Wisconsin Public Television, the Wisconsin Alumni Association, and the UW-Madison Science Alliance, thanks again for coming to Wednesday Nite at the Lab. We do this every Wednesday night, 50 times a year. Tonight, it’s my pleasure to introduce to you Laura Albert. She’s a professor in the Industrial and Systems Engineering department here in the College of Engineering. She was born in Park Ridge, Illinois, and she went to Conant High School in Hoffman Estates, Illinois, and then she went to the University of Illinois to get an engineering degree, and she got her Master’s and PhD in Industrial Engineering at the University of Illinois. And then she went to Virginia Commonwealth University as a professor, in Richmond, Virginia, but in 2013, she saw the light and came here to UW-Madison. She has a really interesting range of topics tonight. She’s gonna talk about Advanced Analytics, for emergency response, bracketology, and beyond. I just wish we had better news about our basketball team being in the brackets this year, but maybe next year. Please join me in welcoming Laura Albert to Wednesday Nite at the Lab. [applause]
– Well, good evening, it’s a pleasure to be here. So, as Tom mentioned, I’m a professor of Industrial and Systems Engineering, and I think today, you’ll learn a little bit more about what systems are and what systems engineers do. As Tom mentioned, I’m a professor by day. I’m also an assistant dean in the College of Engineering, and I wear several other hats in addition to these two. I also write a blog. My blog is Punk Rock Operations Research, and I’m also a vice president at the Institute for Operations Research and the Management Sciences, INFORMS. I’m the VP of Marketing, Communication and Outreach, so very active in my community and keeps me busy. There’s a lot of fun things going on. Today I was asked to talk about bracketology, and also some applications of optimization and systems engineering. And so, what I’m going to do in my talk is first start with a roadmap. What’s a system? What do systems engineers do? What kind of things do we study? And then, the middle part of my talk will be about my research. Most of my real research is in public sector, operations research, or public sector problems. I’ll talk about emergency response today, and I’ll also talk about bracketology. And at the end, I’ll offer some concluding remarks about analytics and operations research. So I mentioned a couple of things, industrial engineering, systems, and operations research, and analytics. I’ll start with a system, all right, so I study systems, and a system is a set of things. It can be people, vehicles, basketball teams, and even cells, and they’re connected in such a way that they produce their own behavior over time, So, I like to say that one car is just a single vehicle, but a collection of cars can be a traffic jam, and that’s the type of behavior that we often study.
My discipline is called operations research, and it’s the science of making decisions using advanced analytical methods, so we’re focusing on designing systems, focusing on these interconnections between components, and really engineering a system that works better. Admiral Grace Hopper, who’s a Navy Admiral, as well as a computing pioneer, said life was simple before World War II. After that, we had systems, and this is a nod to how complex it is to design and manage systems, and it’s also a nod to World War II. What happened in World War II was really the birth of my discipline of operations research. The name actually refers to military operations. That’s why it doesn’t refer to systems or optimization even though that’s a lot of what we do. And World War II was a major military campaign. We had to move troops around. We had to move around a lot of supplies to support the troops. That’s a lot of what happens in a military campaign, and it’s a huge problem.
It’s on a network, it’s on a transportation network. There’s a lot of moving pieces that are interconnected, and it’s very expensive, and so small improvements that can be made systematically that would reduce cost, improve performance, those things really added up just because of the immense costs involved. That’s kind of the birthplace of my discipline, and also, a reason why we need systems engineering, so we didn’t stop with all those systems in World War II. We see systems around us all the time, and they also need us to manage them. In my discipline of operations research, we studied a lot of different types of discipline. Transportation is the first one I mentioned in addition to the military, so moving goods around, freight travel, train travel, the airlines, scheduling all those flights, getting the crew on the flight, dealing with delays. These are all things that we can do. Manufacturing is another one of our classic application areas, so we don’t make the stuff, we just make the system run very efficiently. Currently, healthcare is probably the number one employer of my students. There’s a lot going on in healthcare.
It’s a big system. You have surgeries, you have doctor visits, there’s a lot of data and information that goes into those decisions, and there’s a lot of delays, so treating a patient takes time, usually repeated visits, and coordinating those is an opportunity to really improve health. We’ve also studied service systems, so optimizing networks of people working together, and this is a picture of a call center. We’re routing the calls to the right people, and matching people with resources, and using them in an efficient and effective manner. I mentioned public sector problems before. I’ve studied security systems, and in particular, aviation security quite a bit. We need a lot of efficiency to get through some of the security checkpoints. And emerging application areas, so a hot area right now is the shared economy, so when you go to a bike dock, you want a bike to be there. No matter where we return it, we always want one to be available, and there’s a lot of interesting challenges from a mathematical and applied perspective in the shared economy. Our world is increasingly complex and it’s very connected, and systems are really important for not only navigating the world we live in, but designing a better future.
So as an engineer, a systems engineer, I really want to design a more efficient system, and I really want to maximize performance, and a lot of this, a lot of what we do focuses on improving performance, and we have a couple of main criteria. One is just making a system more effective. How well does this system work? So typically, we’re looking at throughput, getting things through the system in the quickest way possible or pushing the most supply through. We can also maximize revenue, or in public sector problems, sometimes this is minimizing risk. We also look at cost, so how much does the system cost to operate, to design the system. We can look at actual dollar costs, or we could look at cost in terms of human capital, such as waiting times. In general, we don’t always get to entirely control the cost so we look at efficiency. You’ll hear me mention this in the talk. I think I’ve already mentioned it, and we want to design a system that’s cost effective, so how do we get the most performance out of a given set of inputs? How do we get the most bang for our buck? This is something I think about all the time. So you might be wondering, what’s stopping us from getting the most performance possible, the best possible performance? Well, the reason is we have limited resources all around us, and when I look at systems and operation, I’m constantly noticing the limited resources we have and where bottlenecks occur.
We can see evidence of the limited resources, in terms of, for example, queues or congestion. Where lines form, there’s usually a limitation there. Limited capacities or supplies, so we may only be able to make so many widgets per hour, and that might be a limitation in the system. And another limited resource is time, so we quite often have time delays due to travel, I mentioned transportation problems, processing, or information, so if you’ve been in the healthcare system, you know you’re waiting for lab results before you can make your next decision, and these all inform the design and operation of systems. When we think about getting the most we can out of a system, we want to think about a dial. This is how we think about it. How do I kind of maximize performance and increase the dial, but usually with systems, it’s a little more complicated. What we’re actually doing under the hood is using our limited resources wisely, and usually this comes back to that system, and these interconnections between the components in the system. If we can use our limited resources to manage those, we can improve the overall performance of the system, but that means managing a lot of these moving parts. I’ll talk about applications in a little bit, but first, I want to talk about data analytics and where they fit in, so we have a lot of data available to us, and engineering is, of course, becoming increasingly data driven, and I have a picture here of a big data.
It’s the Niagara Falls of data because there’s so much data that’s out there, and I would like to say, the data are just data. The point of having all that data is that we want to turn it into information for it to be useful. All right, so there’s a number of mathematical and statistical tools that can help us get from data to information. As an engineer, I want to go one step further and use that data and information, apply some advanced analytics to it, and actually make better decisions, and inform the design of a system. So I have this on a continuum moving from data to information or insight into decision, and we start with the data part. There’s actually a suite of advanced analytical methods that help us answer all different types of questions, and so usually when we have a lot of data, we might ask questions like what happened and why. So this is very much looking backward at the data that was collected in the past, and trying to piece together what happened. A number of statistical methods, artificial intelligence, machine learning, data mining, data visualization, the typical data science tools that you may have heard about are used to answer this first question. It’s kind of looking into the past a bit. If we want to look into the more immediate future, we can ask, what will happen, all right, so we can talk about forecasting.
I use forecasting and simulation methods here. We haven’t quite got to a system yet, but if we ask the next question, what should we do, this is where a decision comes into play. We can use optimization, and more advanced simulation tools. In operations research, we call this prescriptive analytics because we’re prescribing decisions. We’re trying to understand what a good decision will look like, and generally, that’s a good decision made in a system, so I’ve got my picture of my Slinky here, and the Slinky is to represent a system, because if you move one part of a Slinky, you’re going to affect the rest of the Slinky, and you see this in systems. If you change one part of the system, there’s ripple effects that affect many other parts of the system. And that can be difficult to manage. This is why we have optimization. So this is my pitch for what we do as systems engineers, and why optimization could be useful. In the next part of my talk, I actually want to talk about some of my work in emergency medical services, and so, this is actually ambulances and fire engines often responding to 911 calls.
I’ve been researching in this area for more than a decade. It’s really fun. Some of my research has been put into practice and can make a difference and that’s a good feeling at the end of the day. The research questions I will talk about today are how do we send ambulances to patients in real time, what’s the best mix of vehicles for responding to calls for service, and what’s the impact of severe weather and congestion on response policies. I won’t really lift up the hood to show you all the details, but I’ll try to explain some of the insights we get, and some of the value of using optimization, as well as some of the trade-offs that we have to evaluate when designing systems. I first want to talk about what happens when there’s a call for service. So, when an ambulance responds to a call, first, the call must be placed. Move that over here, and when that occurs, there’s some triage that’s done on the phone, so a call-taker gets some, collects some data. They try to figure out what’s going on, and then, they send the resource. Okay, so this is when they make their decisions way up front, and then they send an ambulance to the call based on this triage information, so it may not be perfect.
There’s a little uncertainty at this point. Then the ambulance is sent to the call and it arrives at the scene, just this next one here, and this is actually the goal of the system, so I talked about maximizing performance. In many applications, it’s not really clear what performance is, especially in public sector problems. They’re so messy with so many stakeholders involved. Emergency medical services are the exception to the rule. The goal really is to have really quick response time, so typically, the goal is nine minutes, and they want to get to as many calls in less than nine minutes as possible, and this is true for every emergency medical service department in the country. The only difference is that it might not be nine minutes. It might be a different threshold, but they all have the same flavor of goal. After the ambulance arrives at the scene, care is provided, and typically, the patient goes to the hospital, but not always, and sometimes, that taking the patient to the hospital and transferring them to the hospital is most of the work that needs to be done, in terms of the time that it takes. So what’s the challenge here? It seems pretty straightforward in this nice graph that I’ve showed you.
Well what’s going on here is that when the ambulance is sent, we make this decision up front with maybe slightly imperfect information. The ambulance is tied up for a while, right, so sometimes, it can be 40 minutes, sometimes it could be two hours. It’s a long time, and they can’t respond to somebody else if they’re taking a patient to the hospital. So when they’re servicing patients, they’re actually called out of service because they’re out of service for new calls, and this is, we have a couple of limited resources here. Our first limited resource is our service providers. We have only so many ambulances, and paramedics, and EMTs that can respond to calls. The second issue is time. So when an ambulance is unavailable for other patients when it’s serving patients, we have to make a decision when a new call arrives between this patient at hand that’s just called into the system, and future patients that could arrive, because our decision now might have ripple effects into the future. We always have to make these trade-offs, so it’s pretty straightforward if you have a very serious emergency that arrives to the system. You do everything you can, but what if it’s a patient that’s lower priority and can potentially wait? I don’t want to delay service to patients, but we have a lot of data, and I have a good sense of what could happen in the future that can inform some of these decisions.
I took out my dial again, so our goal here is pretty straightforward with our dial. We want to increase the number of calls we can reach in nine minutes, and typically, we want to get to the most serious calls within nine minutes, so some agencies or some departments really do triage their calls and they’ll have priority one calls, or they might use a different scheme to triage and categorize patients. The point is to actually provide good healthcare and save lives. What goes on under the hood, of course, is optimization. Optimization to the rescue, so we want to design response districts, and possibly locate our vehicles, and staff them, and schedule the service providers in a way that we can reduce the response times for most patients in the system. All right, so what this actually means is that, as I pointed out, some of the decisions are pretty obvious. The optimization tells us what we think we should do. If there’s a very serious call, we send the closest ambulance, lights and sirens. We try to get there as fast as we can, and other times, it’s less obvious, and I’ll show you some of the results on the map. This is all about managing these trade-offs between patients so this is a picture of four response districts around four ambulances in a county, and actually most of the calls are sort of in that green region on the bottom right, and if you have a high priority call, you always just send the ambulance in the district.
What’s less obvious is if the ambulance in your district is not available because it’s serving somebody else, and this is where optimization actually has some value. It helps inform us about what a good decision could be for a backup service when our first choice isn’t available, or maybe low priority patients, right. It might be reasonable to have a slightly longer response time to a low priority patient if we can save a life, and that’s what we find. So we find that with so many calls in this green district, we really need to ration that ambulance for the high priority calls. What will happen if we don’t is that there’s so many calls there, it will always be responding to calls, and all those patients in the busiest part of the region will never have a very nearby ambulance, and that’s not good. We find that there are so few calls in the red district that that could be rationed for low priority calls a little bit more than average. And if we actually look at our second choice for our high priority calls, we’ve got four colors here, and our second choice only uses the two ambulances, the blue one and the yellow one, so this is not so obvious until we run the optimization, but now we have a plan, because half the time, our first choice ambulance isn’t available and now, we’re making much better decisions more frequently. Some of my other work has built upon this, and has looked at getting the right mix of vehicles to patients, and this is an exciting project because it was put into place, and I started working with this county in Virginia, and they had a lot of, it was a semi-rural county, there was a housing crisis at the time. They get paid through property taxes, and it didn’t look like they were going to increase their budget for a while, and the response times were pretty long, so we looked at some strategies for improving the response to patients without increasing costs, and they came up with this idea which I thought was brilliant. Normally, you have two staff members on an ambulance, so they can be an EMT, which is an Emergency Medical Technician who does very basic care, or a paramedic which is an EMTp, who can do much more advanced care.
So I’ve just told you how to reduce response times, or at least that reducing response times is the goal. We’re on a transportation network, it takes time. Well they had this great idea of replacing ambulances with these quick response vehicles. They could be SUVs, I’ve got a picture of one here. It was a small truck. It only requires one person to staff it, so now, you can have twice as many vehicles. I can just spread them out a lot more. The odds that somebody would be close to the next patient that arrives is now much higher. You’ve probably guessed by looking at this small truck that it can’t take patients to the hospital. So we’ve got some trade-offs here.
Even though our criteria is really clear, we do have some trade-offs to make, so what we do is we have to send both an ambulance and a quick response vehicle, so now, we’re tying up three people instead of two, and we actually have to look at whether or not the paramedic provided some medical treatment that only a paramedic can do, and so, we looked at what actually happened at the scene which becomes very important. That initial triage is not so important for figuring out how we use our resources. We actually have to look at what happened at the scene. So when we look at this, we have to sort of weigh what could happen, and it was not so obvious initially, although I was pretty sure the quick response vehicles were a good idea. The quick response vehicles cannot take patients to the hospital, and even at the scene, before they go to the hospital with this doubling up. Okay, so that’s a bonus in that we can free up those quick response vehicles if they don’t have to take the patient to the hospital, so you know, we send three. Maybe the paramedic itself gets freed up and can do something only a paramedic can do which can help the health of most of the citizens who need to dial 911, but sometimes the paramedic does need to go to the hospital and in this county, it took about an hour, on average, to go take the patient to the hospital, so now we’re tying up three service providers instead of two so we have this potential introduction of a huge inefficiency in the system. This is why we need to use optimization and model the system and consider different scenarios, and we found that optimization models suggested that this was a really good idea. So the county was great. They used the idea.
They put three quick response vehicles out in service. It’s pretty nice. They wouldn’t let me use my optimization to tell them where. They said that was too political for Math, so I took their word for it and, uh, but it was nice to see it happen. And what was great about this is the actual results were a lot better than I predicted because what happened was the service providers actually responded to the system, and the paramedics would no longer give somebody an IV unless they really needed it, because they would much rather be freed up to serve another patient than have to go to the hospital. So it was in their best interest to kind of be available for future patients. I want to show a graph because I’m so proud of this work. As a professor, we don’t always, and especially in public sector problems, we don’t always get to see our work put into practice. We found, by comparison to the previous year, that we improved the number of calls, the fraction of calls responded to in less than nine minutes. The goal was to get to 80%.
The priority one calls in the middle there, are the high priority calls that we are most interested in, right here, and you can see, we went from 75% the year before to 80% after, and in almost any application area, this is a really enormous increase, or improvement in performance if you don’t pump extra costs, or extra resources into the system, so that was really great, and it improved the response to all calls as well. And it also won a national award, which is really neat, so this is pretty exciting work to do, and yeah, so there’s a lot of interesting challenges to work on. I really do enjoy working in emergency medical services, and there’s a number of problems that I’ve worked on, and I’m also a native of the Midwest, as you learned earlier so I’m sort of obsessed with weather all the time, and I lived in Virginia, and it was really interesting to see how they could not respond to snow, and I think they’re getting, they have a snowstorm today. My neighbors saw me once shoveling the driveway, and they just said, you know Laura, we know you’re not from around these parts ’cause you own a shovel. [laughter] We got 14 inches of snow in that Nor’easter and I just needed to shovel my driveway to not feel trapped, as you know how it goes. But I’ve been very interested in responding to emergencies during emergencies, from regular weather events, like snowstorms, but also more severe weather events, and I have a couple of research projects, some are done, some are ongoing where we’re looking at this issue, because what happens is everything is different, so I told you what we like to do for typical, day-to-day emergencies, you want to respond immediately. There’s almost always an ambulance that we can use to respond immediately, and all that goes out the window when we have a weather event, right, so we don’t want it to be a disaster, that would be bad, but things can become disastrous if we don’t manage them well. So after a number of these snowstorms, I started collecting data, and I started looking at the impact of congestion in the system. That’s a nice way of putting it, so I looked at actually what’s gonna happen in terms of the system, so first of all, there’s a surge of patients. From what I’ve seen and from what I’ve studied, no matter what the emergency is, there was a surge of mostly low priority calls into the system, so you have these real serious emergencies that you need to respond to, but they get diluted by all of these other calls that happened, so before Hurricane Sandy, the mayor was telling people to dial 311 instead of 911 to help people self-triage, and help them use their limited resources better.
That’s not the only thing that goes on. Critical infrastructure is impaired or destroyed. Now typically, it’s just impaired for a while if there’s ice or snow, but that’s actually pretty significant because all these ambulances are driving around on the road networks, and if you increase the amount of time it takes, sometimes it’s not the increase in call volume that is the problem, it’s the extra time per call that really keeps the service providers busy, and now, you’re no longer allowed or able to respond immediately to a call. People have to wait. And this is an engineering term, but I say we have cascading failures in the system, and that just means that there’s a weather event and it’s icy in one part of the city, it’s probably gonna be icy in many parts of the city, so we can’t treat these as independent issues. Everything is sort of affected, maybe not equally, but a lot of things are affected in a similar way. And we started looking at what happened and what actually happens in the models, and I didn’t show you what happened under the hood but all of the Math models we use make assumptions, and assumptions have to be reasonable for the application that we’re considering, and I make reasonable assumptions for everyday emergencies, but those assumptions can’t really be applied to other situations, and I have a picture there of an ambulance stuck in the snow for a reason, and quite often, public services are not managed very well during weather events, unless they’re really, really serious, like a hurricane. So this really motivates a need for new models, new system engineering models to help us design data-driven decisions for new situations. One of the things to think about here is just the decisions may be different, so most models typically would say, you can always respond immediately, and you should respond immediately. That may not be possible here if you have a big line that forms due to so many calls due to the surge and slower transportation, so we might have to strategically ask patients to wait for service.
We don’t want anybody that really needs immediate care to wait for service, so we want to be really selective in how we ask patients to wait, but that might be something we have to do differently. So I have some papers that I’ve written in this area, and some that are ongoing, and it basically studies emergency response on congested networks, and we look at systematic ways to ask people to wait, and typically, you want to delay low priority calls when the system is very congested, and if you do this strategically, we don’t have to ask too many patients to wait, or we don’t have to divert too many to other regions, but we can really improve the performance of the system for the patients that need the most care immediately. I also had a student do a really nice work using simulation, and we studied a number of situations, and we wanted to study staffing levels, and what was really interesting is that we have human service providers and I was showing you earlier some of the applications. You might have noticed that there’s a lot of people in those pictures, in those systems that we’re managing, and that’s true, and so sometimes, people behave in somewhat surprising ways, and in the presence of congestion, people actually feel the pressure and the heat a little bit, and they’ll work faster, and it’s measurable, so we have enough data that we can actually measure that, and it’s not a lot faster. It’s just tiny bits, but we have a system of patients and service providers, and it actually does make a difference. We noticed that patients were slightly less likely to go to the hospital in a snowstorm. Maybe they were, just the bad weather, they were a little bit more unwilling to travel unless they really had to, but it was just a small fraction of patients, a very small fraction, but it made a difference, and it was almost like having an extra service provider in the system, and so the system itself was a little bit resilient up to a point of handling the congestion in the system, and that was really interesting. All right, so I want to switch gears. I know some of you might be here to hear about bracketology, and I have no explanation for the historic upset of Virginia. I was looking at the numbers before the day, and sometimes, you know, rare events happen, but there is a little bit more system to the rest of what I do and what I study in bracketology.
I’ll talk about basketball and football, although I got started in football bracketology. I got started, as mentioned earlier, I joined the university here in 2013. It was great. The basketball team went to two Final Fours immediately, but a year after I arrived here was the first college football playoff, and somebody said to me, well, you should study bracketology for football, and I agreed. I thought that was a great problem, and you know, it’s painful to watch college football if you’re an Illinois alum, so the math is little bit more comforting to me, and it keeps me going, and I do post the Big 10 rankings, and it’s sad every week for me in some ways, but I like the methods. So my goal here was really to forecast the first college football team, so there’s no optimization here, but it’s really kind of this data-driven data science problem, and I actually wanted to do some forecasting. How do we recognize which teams might have a chance, and what’s interesting about this problem is the college football season is really short, and I wanted to see if we could do this with math and small data, just a few data points. The teams play about 12 games. And I also wanted to bring this into the classroom a little bit, and use some methods that I have in class to talk about this. Not everybody follows football or basketball, but students understand winning and losing and trying to recognize the best team, and it’s kind of interesting.
So the method that we use is Markov chains, so this is a mathematical model. I will open up the hood a little bit on this one, and we’ll talk about this, and this is a mathematical model that helps us understand how a system evolves over time, and you can wrap an optimization model around it, and I do that in other parts of my research too. Markov chains are used very widely. They model systems that operate probabilistically, so they often are used to model financial systems. They’re used widely in epidemiology to model the spread of disease, or even zombie outbreaks, and they provide the science of queues, the science of waiting in line. There’s a science to it, and so there’s a wide variety of applications to Markov chains. It’s pretty interesting. So those are probability models, but you can also introduce some data into the system, and you know, you can ask questions like how will a system perform over time in an uncertain environment. So this is something that a Markov chain can answer. I also wanted to ask questions with my bracketology about how do we draw conclusions from limited data, and how do we make data-driven decisions in the presence of uncertainty.
So I want to talk a little bit about how we do this with sports, and I’m going to show you a picture of this, and it’s pretty intuitive because in a Markov chain, you move from state to state, and so this is the college football season in 2014. It’s a graph, and you move from team to team, so each team is a state, and you can see here that the teams are connected if they play each other, and you can see these clusters of different colors, and these are the conferences. You can see that there’s a lot of structure here already when we look at the system. Some of you are trying to find Wisconsin, so there you go. You can see the Big 10 up there, and what we do in the Markov chain is we sort of vote, so I’ll introduce a partial voting scheme in a few minutes. We vote for the team that beats you, okay. If several teams beat you, like Illinois, you can just choose one of them randomly, and you move to that team, and Wisconsin, last year would just vote for Ohio State. So what you do is you’ll notice if you’re not a good team, you quickly leave that team and you’re more likely to visit better teams, and if you’re a good team, you’re visited quite often, and if you’re a great team, has a great record, you win a lot, and you beat good teams, you’re visited the most frequently, and so this amount of time we spend visiting the teams, and you can imagine something wandering through the system over an infinite time horizon, we will recognize who the best teams are with a single metric, or the fraction of time we spend on each team, and it actually works pretty well. Some of you may be wondering, does this have other applications, or maybe I’ve thought about this, I’ve heard about this before, and Google’s PageRank algorithm, one of the algorithms that changed the world in which we live is based on the Markov chain. They don’t have games, they don’t have winners or losers, but they look at websites and they see who they link to, so most websites have links to other websites, and if you look at analytics, it would probably just send you to informs.
org, or if you are very interested in the best engineering schools, it would probably take you to UW-Madison’s homepage, right, and this is because all the places that talk about analytics link to informs, and places that talk about great places to study engineering, they might link to Wisconsin among some of our other great schools, and you have these websites here which are always sorted by smiley faces, but the ones with the most incoming links are the biggest, right, so they will show up first in your search results. So I’m looking out at the audience and you look like many of you are old enough to remember Internet searches before Google, and they were pretty terrible. I’ve used most of these, and most of them don’t exist anymore, ’cause once we had Google, the best, the most information, the sites with the best information typically showed up first, and we no longer needed a human to parse through. We could just do it using math. Just math could recognize where the important information was on the internet, and it works with ranking sports teams as well. All right, so how does this all work for ranking sports teams? It’s pretty simple. I use simple data, and part of this is so I can teach with it for students who think, many of my students are international and they think football is a different sport, but we use simple information. I use a partial vote scheme, right, if you win by a lot, you should get more credit than if you win by, in overtime or win by a point. And so, we have to look at game outcomes, so in this case, it’s just score differentials, and I look at home or away status, so home field advantage is a huge thing, especially in college sports and I adjust for that, so you get less credit for winning at home than you do on the road. I’m often asked, well how do you account for strength of schedule.
Well the Markov chain accounts for strength of schedule. It’s just endogenous into the model. It captures these movements from team to team. At the end, I’ll talk about how I actually use this for forecasting, and I simulate the rest of the college football season. I don’t want a human in the loop having to figure out who the top teams are. I want to do it automatically, and I can do this if I can rank the teams. Okay, so here’s a quick example. This is from a couple seasons ago when Wisconsin made the tournament, and they played Rutgers twice, so in college basketball, many of the teams play each other twice if they’re in the same conference, and I can use this as my data for evaluating those partial votes that I’m going to be giving to teams, so the first game, Wisconsin beat Rutgers by 20 points at home, okay, so I have to figure out what this partial vote is. You can see four arrows between these two teams. In this partial vote, you give the rest of your vote to yourself, so it’s an arrow back on, from a team to itself, and I only need to know this one parameter, W, which is my partial vote parameter here, so the question is, how much credit should Wisconsin get for beating Rutgers by 20 at home, so this is the question.
I want to have a data-driven answer for this, so I don’t have the exact answer, but I can say, what’s the probability that Wisconsin will beat Rutgers next time on the road. So I can start with that, and I can look at this, and I can plot all the data, so I took all the data from several of these seasons, and I said, put the home game, the first game score differential on this x axis here, and the next game on the y axis, so you can see where that no games end in a tie, so you can see that this kind of cross where the zeroes are, and this slice here for winning by 20 at home in the first game is kind of the Wisconsin-Rutgers situation, so one thing you’ll notice here is you’ll see sort of this pattern. It’s positively correlated to a large degree, but it’s not perfect, so if you actually look at the amount of data points above the line, which would be a win for Wisconsin the next game versus below, you see that there’s actually a good chance a team would lose in the next game, even though they won by 20 at home, but this is what the data tells us. So we can actually fit a distribution to this, and I used logistic regression. It’s a real workhorse in data science, and it’s fitting a curve to a line, and it’s always between zero and one, so it always gives us something that’s a partial vote. You can see here that there’s about a 62% chance that the team would win the next game on the road, and they will not benefit from home field advantage. Next time, the opponent will. Rutgers will, in this case. But this is kind of a surprising answer, but it gives us a data-driven answer. You also notice that, let’s say you happen to tie the first time, you will actually have less than a 40% chance of winning next time, so home field advantage is pretty huge, and I have to make the adjustment for home field advantage, and account for what a neutral site win probability would be, and I’ll show you how I do some of that.
So I end up getting this line here. This is my S-shaped curve. I used log point differentials. This is especially helpful in football when they run up the score, and mathematically, that makes a huge difference, so I have to do something about that. One of the issues with these curves though is let’s say, you win or lose by a point, so you’re right here on the zero mark. You actually get about the same credit. You have about the same probability of winning the next game, and I don’t want to give those two teams the same amount of credit for winning or losing by a point. You won, you should get some extra credit there, and so, I used the pure vote model which is you get everything if you win, and nothing if you lose, and I sort of averaged them together to get this red line here, and so now, there’s more of a difference between losing by a point and winning by a point, and in football, this seems to be the special sauce that makes it work because there’s only 12 games in the season. The red line gives me my partial votes that I get, so the Wisconsin-Rutgers example, Wisconsin did beat Rutgers by seven on the road next time, and they have a 62% chance of winning on a neutral court, but I would give them 68% of the vote for the win. There’s a lot of basketball games.
There’s thousands of them. So I put it all together for all of the teams, then I look at what the math says. My Selection Sunday rankings this year look like something reasonable, right, for Selection Sunday. I do have Virginia ranked number two. You see Nevada here is 24 and Loyola is just off the charts here. They were actually pretty highly-ranked. So we see some of the teams that actually make it into the Sweet Sixteen that seem like upsets actually are ranked quite high, and you know, some teams blow it in the tournament. The math can’t tell you when that will happen necessarily. You can make an educated guess, but you never really know. At the end of last season– Oh, there’s Wisconsin, sorry, 68th.
I have a composite ranking too that uses Markov chains and some other things and they were a little bit lower than 68th, and that was even sadder. And last year, these were my rankings. I had Wisconsin ranked number 12th after the tournament, and North Carolina was correctly recognized as being the number one team in the nation after winning the national championship. So college football is where I started, and it works in a similar way, except the rankings just tell you who’s highly ranked now. It doesn’t tell you anything about the future. I like to know about the future, so I wanted to look at who the best teams are at the end of the regular season, and so I ended up using the rankings, but then simulating the next week of games, and seeing at the end of all this, the end of my simulated season who would be ranked in the top four. So the rankings are great. They give me the top four teams. And as you know, the top 68 teams do not make it into the basketball tournament. There’s a lot of automatic bids.
In football, we don’t necessarily have that issue. So most of this looks pretty similar to what we saw before. I do actually have to observe a few weeks of games so usually about six games which takes about seven to eight weeks of play to collect some initial data. I do my rankings with Markov chains, and then, this middle part is new. I simulate the next weeks of games, and I use another logistic reduction model here to understand what the outcome may be, and this looks at the difference between the rating and the two teams, helps determine this win probability in this step. I simulate the rest of the season, including the conference championship games, which is really critical for understanding who might make it into the playoffs. This past year was the only year I disagreed with the committee on one team, so in four seasons, I’ve done pretty well with the math. This is what I had last year. I did have Wisconsin ranked fourth after the loss to Ohio State. They lost one game to a really great team.
I thought they were way up there. I don’t give anything special to Wisconsin in my rankings. There was a big cluster around, right below that, and so, Alabama was seventh, but it was almost a tie with some of the teams ahead of them, and they were ranked number one at the end of the college football playoff. This is what my rankings, these are the rankings, so this doesn’t have the forecast in them. One thing to notice here as you look across the top is you only see Wisconsin in this one spot here at number one, but you see them up near the top. You see Alabama a lot in the top two places. You’ll see the impact of a loss right here, so Alabama goes down two places with a loss. This is just the ranking. What’s interesting about this simulation is that it tells us not only about the strength of schedule, it tells us about the strength of our future schedule, and how hard it is to get into the playoffs, and we see that when we look at the simulation results. So here’s at the end of our rankings, is what we have on Selection Day.
Our forecasts look a little bit different. First of all, you see Wisconsin at the top a little bit more, and this is because the Big 10 West really is easy. That’s an easy path to the playoff, to be honest, and I think, Wisconsin this year could have made it in even with a loss, and you see some of the teams are actually ranked differently, and this is because some teams have a more difficult path to the playoff, and here you’ll notice that when Alabama loses, they were pretty unlikely to make it into the playoff, so they really needed Wisconsin to lose, and the drop off was because they lost the opportunity to play in the SEC Championship Game, and that’s an important part of the path to the playoff. That’s not my opinion, that’s what the math tells me. And that’s something that pops out of doing the models, and something we can learn from all the models. So just to prove that my model works pretty well, this is what I had the year before, this past year, and I agreed with the committee. I had Clemson and Washington tied for third, third seed. But it’s pretty amazing. I’ve just been amazed every year that just the math can rank the teams and do pretty well on the forecasting with just a few games. It’s been pretty exciting and fun to do.
So I want to wrap a few things up at the end to bring things back to systems engineering and analytics and some ways we can think about it. The first thing I will say is that, as an engineer, I say that the data very much look into the past. They’re very backward looking, and I tell this to my students all the time. Data reflects something that was collected in the past, right, at some point. As an engineer, I’m really much more focused on the future, and I like to separate out those two ideas to stay focused on system design, making better decisions, designing systems. These are all things that we want to do in the future, and we have to understand the limitations of the data as we’re designing data-driven systems. I’ll repeat again, the data are just data. You want to turn that data into information for it to be useful, and then you want to apply advanced analytics, like optimization and Markov chains, so that information and data to make better decisions. I’m a big fan of a lot of the data science methodologies. We hear about data science so often, but what you may not know is that data science doesn’t tell us a lot about the system, and how to manage a system.
Those methods are just better used to answer other questions and prescriptive analytics and optimization really help us manage these connected systems where we have many interrelated decisions to be made. And also, we don’t always need a lot of data to make a difference and to design a better system. I think about the future a lot. I have some pictures here of some fake robots that are some of my favorites and an actual self-driving car. I think a lot about data-driven engineering and autonomous vehicles, and you know, some people ask me, well are humans going to not be part of the loop. I talked about not having humans in the loop, or having humans in the loop in my talk today, and you know, there will be a lot of automation, but quite often, we will see a human in the loop somewhere in many applications for the foreseeable future. Even automated vehicles need a human to step in and take over, or perform a procedure here and there. That tends to be the case in systems engineering. With systems with a lot of interconnected parts, quite often we have human decision-makers that have to do something in these decisions, and make a decision. So analytical models can also supplement human decisions instead of replacing them, and we’re increasingly seeing this right now.
The example from my talk, as I said, a lot about response districts. We still have call takers. We still have people that have to make the decision. The software might make some recommendations, but a person, at the end of the day, needs to make that final call. Same thing with doctors. They’re increasingly using algorithms, and looking at risk assessments, but the doctor still makes those treatment decisions. Finally, I’m an optimist. I study public sector problems. When advanced analytical methods started to be used for public sector problems in the ’60s and ’70s, somebody wrote something, and they said, “Planning and the emerging policy sciences are among the more optimistic of professions. Their representatives refuse to believe that planning for betterment is impossible,” and I think engineering is very optimistic, and it’s part of why I like being a systems engineer. But who knows, I could be wrong. This is just my opinion. I would like to wrap things up. I am very grateful that you are here tonight with me on a Wednesday night. It was a pleasure, and I’ll be happy to answer any questions.
[applause]
Search University Place Episodes
Related Stories from PBS Wisconsin's Blog

Donate to sign up. Activate and sign in to Passport. It's that easy to help PBS Wisconsin serve your community through media that educates, inspires, and entertains.
Make your membership gift today
Only for new users: Activate Passport using your code or email address
Already a member?
Look up my account
Need some help? Go to FAQ or visit PBS Passport Help
Need help accessing PBS Wisconsin anywhere?
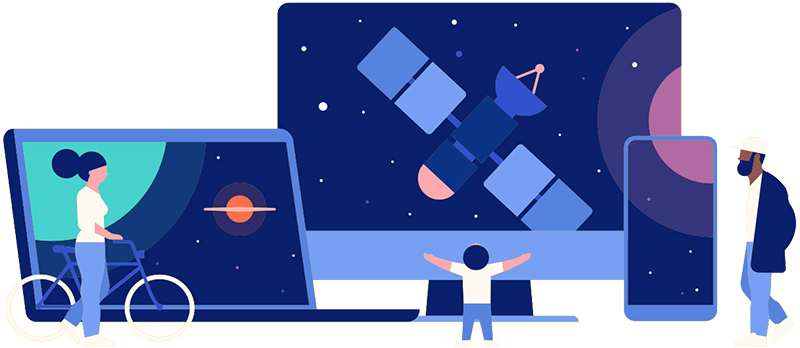
Online Access | Platform & Device Access | Cable or Satellite Access | Over-The-Air Access
Visit Access Guide
Need help accessing PBS Wisconsin anywhere?
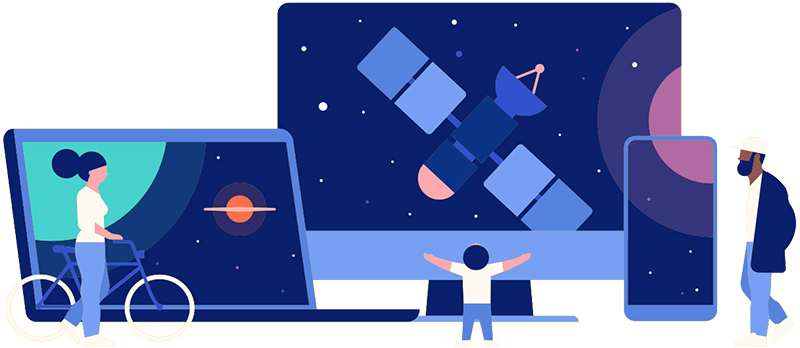
Visit Our
Live TV Access Guide
Online AccessPlatform & Device Access
Cable or Satellite Access
Over-The-Air Access
Visit Access Guide
Follow Us