– Welcome everyone to Wednesday Nite @ the Lab. I’m Tom Zinnen, I work here at the UW-Madison Biotechnology Center. I also work for the division of Extension here at UW-Madison. And on behalf of those folks and our other core organizers, Wisconsin Public Television, Wisconsin Alumni Association, and the UW-Madison Science Alliance, thanks again for coming to Wednesday Nite @ the Lab. We do this every Wednesday night, 50 times a year. Tonight it’s my pleasure to introduce to you Jason Fletcher of the La Follette School of Public Policy here at UW-Madison. He was born in Athens, Tennessee and went to high school at Athens. And then he went to the University of Tennessee where he studied ECON. He came here for a Master’s and PhD in Agricultural and Applied Economics, which is the building right through that wall, and then another wall, and then you’re in Taylor Hall.
Then he went to be on the faculty at Yale University. Then he was on the faculty at Columbia University. And in 2013 he returned to UW-Madison to be part of the La Follette school. Tonight he’s talking about something that’s pretty important to a lot of us, especially in the DNA field. He’s going to be talking about “Molecular Me, “Exploring the Social Implications “of the Genomics Revolution.” Would you please join me in welcoming Jason Fletcher to Wednesday Nite @ the Lab.
(audience applauds)
– Thanks Tom. Thanks, and thanks to Tom, and thank you all for coming. So tonight I wanted to outline partly some of the advances coming from the genomic revolution, and then really focus in on questions of law, policy, and ethics that are then implicated through these advances. Especially honing in on questions of privacy, discrimination, and overall human flourishing that are going to come up.
I’ll start with a picture of very recent accumulated knowledge. These are our chromosomes with all the, almost 90,000 relationships that have been found in the last 15 years between points in our DNA and outcomes of interest, mostly health. So for example, if you zoom in on this and picked a color you would find over 1000 individual locations in the human genome that have been linked to digestive system disease. Over 700 to cardiovascular outcomes. And this is all accumulated, this as of last month. It totals almost 4000 publications in the last 15 years that are on this one figure. So that’s a lot of knowledge there, and what I’m going to do is pivot from a book that a sociologist, Dalton Conley, and economist, me, wrote a couple years ago to outline the last 15 or 20 years of integration of genetic science and social science. We’re both social scientists, although Dalton has a second PhD in biology.
And we walk through some of the highs and lows of the last 15 years or so in this integration. And just to fast forward to the end, because this is now a very dated book even though it was published two years ago. In terms of keeping up with the science, I think what you would find is that our, quote unquote, “Future” in the title, this part of the title needs an update even though it’s only a couple of years old. And that there’s an immense need for policy discussions and implications that were long overdue when we wrote it, and now are even more so. So that’s what I’m going to focus a lot of the discussion tonight on. So the book describes the social genomics revolution, I want to start by saying you need a genomics revolution to have social applications. I’m going to describe what we’re calling a genomics revolution, What’s revolutionary about it.
But first I’ll remind you of a few essential facts to then lead us into the findings. One is just a quick refreshment over what a human genome looks like, and in particular, I’ll call these letters throughout the talk, but the three billion base pairs of nucleotides that make up the human genome. That are about 1.5 billion miles of DNA in your body. And if you took all the letters and made a textbook out of them, then the text book would be about 65 feet high. So there’s a lot of information content in these letters, and each of the cells of our body. That’s reminder one. Reminder two is that although there’s a lot of content, there’s quite a lot of similarity across all humans. Over 99.5% of all these letters are the same, or identical in all humans. So that points to a lot of common humanity, I think, in similarity across everyone you’ll ever meet. It’s also true, so 99.5% or more are identical, it’s also true that 98% of all the letters are identical with chimps. So there’s also some differences. Some of these letters that are different do matter.
That’s the second point. And I would have an example of an instance where they matter. So the first row of letters are, if you zero in, on chromosome 11 and do a quick read on a small number of letters, that’s the top row, you’ll see a normal cell reads out CAA, and so on. If you go down to the sickle cell, you’ll see that all the letters are the same except for one. So this is an instance, in all those three billion letters where a single letter difference across humans could have really big implications. So that’s point two.
Some letters really matter in explaining difference. And then point three is that the letters are permanent in terms that you had the same letters when you were conceived. You’ll have the same letters when you die, approximately. We have a little asterisk there that this is a little less true than maybe the other parts, because there is mutations over your life. But those are, I’m going to call those minor. I’m simplifying there, but I think for the purposes of most of the policy implications that I’m going to describe today, we should think of these as permanent. Set in stone when you are conceived, and following you your entire life. It’s an approximation of the truth, it’s not exactly the truth. But these are the basic facts I want to start out with. So these aren’t revolutions themselves, these are reminders. Then I want to say, I want to describe what the revolutionary part is, because the facts I just outlined were the same today as they were 20 years ago, as they were a hundred years ago. So, those are the facts. What are the components then that make up a revolution? And that’s what I’m going to just take a few minutes time on. So the first component is the technological improvements over the last 10 or 15 years in just measuring human DNA.
And then the third aspect, after you get from facts to the technological improvement, is the use of those improvements in terms of mining very large data sets of human DNA. And using advanced statistical methods to then take the DNA to predict outcomes of interest. What I’m going to describe tonight are some of the policy implications of this revolution. So, the first three components are the genomics revolution, but then they’re going to have implications for social outcomes and policy. So that’s what I’m going to be describing tonight.
A quick summary of a lot of advancement.
I want to first just remind you of just normal life, which is to say that over the last 20 years some prices of goods that you buy have gone up, some have gone down. Some of those on top are education and healthcare expenses. Those prices have gone up in the last 20 years. And you can see some of these education expenses have more than doubled over say 20 years of time. You can also see areas in your life, televisions at the very bottom of the screen, that’s a part of your life that is a lot cheaper now for what you’re getting than it was 20 years ago. So this is just kind of recalling that we’re all living these lives, and we’re buying things and these prices change. Because I want to remind you that to then make the comparison with what’s happened on the DNA side. Okay, so this is normal. This is unprecedented. This is the cost per human genome since 2001. And what you’ll see on the yellow line that is at the bottom, which is pegged to the other unprecedented change in costs which is computer and technology, computing. So this is Moore’s Law, the green line on top, which is flat.
Costs for genome is, this is the unprecedented part, is accelerating much faster than even Moore’s Law, which would be the closest comparator.
So to describe this just a bit more, so in the last 15 years the cost per human genome has fallen by 100 million times in 15 years. So you can see some of the different projections out on the right-hand side of the graph, where depending on what we believe on future advancements. You know, the cost of sequencing a human genome could go from 100 million or more 10 or 15 years ago, to either $100, for $10, or $1, depending on which is the lines you follow. And so this has tremendous implications for data that is then available to analyze. So one implication of these tremendous reductions in costs are companies…
where you might send your biological sample, your saliva, and for relatively low cost direct to consumer, they can genotype you as a human being at relatively low cost. And these are some figures I have up here for a few of the private companies that you’re probably familiar with.
And some figures on their growth, and how many people are in their data sets,
from 2015, 2016, 2017, and a few months ago. So you see five million plus at 23andMe. Seven million plus from last year at AncestryDNA. Family Tree a couple years ago was closing in on one million. If you add those three private companies together you get about one in 20 of the US population. And this is a shift also, as just a larger point to make, from scientific analysis of data to private companies. Scientific meaning, I mean by that, at a place like University of Wisconsin who might analyze a lot of human genetic data, which is true, to private companies that have now five, seven million of individual human genetic samples. And just as a point I’ll come back to, is the expansion. Just so example you see under the AncestryDNA there was a sale after Thanksgiving in 2017 and they received 1.5 million genetic tests over the weekend.
And they can do that because it’s so cheap as the previous slide. So 1.5 million saliva samples is about 2000 gallons of saliva.
(audience laughs)
It’s about the size of a modest aboveground pool. In the future, you know, future’s hard to predict. Here’s a couple tries at predicting how many human genomes will likely be analyzed in the coming years. So there’s different versions depending on the red, the orange, or the blue projections. But the most conservative estimate is by 2025 we have over 100 million human samples analyzed.
So this is a huge expansion in data. This is big data. And I’ll be turning in a minute to the use of this data, I just want to fill out the picture of who’s collecting the data. So you have the private companies, 23andMe, AncestryDNA, and so on, but there’s a lot of government efforts also across the world, in amassing relatively large, you know, in the hundreds of thousands samples of human DNA. Here’s a small list of examples and when some of the enrollments began. So you see Iceland way ahead. And that whole country is now in one data set,
and so on. In a couple of examples from a little bit over a year ago, where Finland had a relatively large government program, 500,000 people was their target enrollment. New South Wales, three million people was their target. Many of you have probably seen something about the US version of this which is meant to collect one million US data points. Just to remind you, one million, that was what AncestryDNA got over a weekend in Thanksgiving.
Just think about scale of what this collection is, versus some of the other data collection. So this is the government version. So that’s a quick summary of the technological improvements. So huge, huge unprecedented reductions in the costs, that leads to a lot of access. A lot of availability at very low-cost of sequencing a lot of people. Okay, so that’s part one. And then there’s part two, what are we, we as governments, private companies, clinicians going to do with all this data. And that’s where statistical improvements and predictive analysis is going to come in. So I’m going to give you a little bit of background on that first figure that I put up where there was 90,000 associations discovered in the human genome that linked specific locations in the genome with outcomes. So what’s behind that, what’s the machinery behind that, and I’m going to spend a couple of slides on that, of what are we doing with the data. And that is with the purpose of having some predictive capacity, mostly in the health arena, but not only in health outcomes, so that’s what I want to describe. So the first figure and you see the chromosomes from before without all the dots next to them.
All of those studies are based on a way of examining relatively large data sets of human DNA called Genome Wide Association Studies. Which is to say the steps are you get a big data set of many people. And each of those people, as you recall, have three million letters in their base pairs in their DNA. And you just point by point scan through the whole data set of up to three billion,
And ask where in the data sets do I find differences among people that are associated in a statistical sense, with outcomes that differ.
And that’s where this last point is, is that your scanning across all of the genome, so that scan can be in the tens of thousands of analyses, up to tens of millions or more analyses, in each of these Genome Wide Association Studies. So the picture version of this is that once you do these scans, you have 10 million results. And then you’re going to want to visualize all those results in some way to ask is there anything in the DNA that I’m finding that seems to be related to my outcome of interest? And the outcome of interest could be cardiovascular disease, or could be height, or could be any number of outcomes. So that’s the left-hand side, is that there’s going to be a scan of the whole genome, in some places you might find hits or associations. And then you’re going to take in some way, and I’ll describe what the someway is in a moment, in some way you’re going to take the information that you just found and try to use it to predict outcomes of people. Those of the two steps. So I’m in ago in a little bit more detail now of just some clarifying examples, I think. So here is an outcome, it’s not height. This outcome is the inability to smell the distinct order produced in urine after eating asparagus. So that’s an outcome, that’s a yes or no outcome. And a Genome Wide Association Study would go point by point across all those chromosomes that are differently colored. And what you’ll see is that this analysis found nothing except one place. And that one place is that huge spike of association. So all the other areas there was no relationship between what letter you carried and this ability. But in one place in the genome there was a huge signal, and you see that here. And that’s what’s called a Manhattan plot. You’re looking for tall buildings in Manhattan plots. That means that you might have found a genetic hotspot that is associated with your outcome. So this is a very easy answer. There’s one place, and there’s no other places in the genome that seems to be related to this outcome.
Here are a little bit more complicated, genetically complicated outcomes. So these are an allergy, food allergy domain. Upper left is any food allergy among peanut, egg, and milk. And then the other figures show separately peanut in upper right, egg in lower left, cow’s milk in lower right. And what you’ll see is, you do see some tall buildings again, but you don’t see a single tall building. You see, in some cases a single tall building with other medium-sized buildings. And in some cases you say maybe many medium-size buildings. So this you might, after running this scan across a whole genome and finding a lot of hits, not just one, you might say that this is more complicated genetically, but it still has some genetic basis, these different food allergy outcomes. And so this is the basis of those 90,000 hits that I described in a very beginning. Each of them comes from something like this where you scan the genomes of hundreds of thousands of people or more, and you do that hundreds of thousands, or millions of times, and in a lot of places you find nothing. And in some places you find something.
And this is a figure of this type of analysis over the last 15 years. And what you see is there was nothing until about 2006, and since about 2006 to the present day there’s been closing in on 4000 of these studies. And over those 4000 studies, all of them will have a picture like this in there.
They have found over these accumulated 4000 studies over the last 10 years have found over 90,000 places that look like this in the outcome.
That level of analysis is about one per day. You’ll get about one of these per day. So a couple days ago here is I think like a one of these. This is for knee pain.
See you take 200,000 people, you run a million tests across the genome, in a lot of places you have not very large buildings. That would places that don’t seem to be associated with reports of knee pain. In some areas like on the very left you see this higher building and that might be a place that you might then go and investigate further what it is about this area in the genome that seems to be associated with knee pain. I just pulled this up because this was a couple of weeks ago. But every week you’ll be able to get a picture like this from a Genome Wide Association consortium of some sort. Okay so that’s the Genome Wide Association Studies. And then like I said, a picture like this you might want to take the information you’re learning and summarize it in some way for prediction.
And that idea, and that use of these results are called creating a polygenic score. Polygenic, many genes, score. Which is like a credit score. Which is amassing a lot of information about you and your patterns of buying, And whether your late on payments, and so on, and creating one number. This is the genetic version of this. It’s looking across the whole genome and saying well you have some knee pain letters over here, and you have none over here. But you have these big ones over on the right-hand side. And you sum it all up, and some people are going to be high knee pain genetic load, and some people are going to be low knee pain genetic load, and it will look like on the lower right. Often these are normally distributed in the end where like I said some people of high genetic risk, and some people have very low genetic risk. And then finally you have this predictive measure that you can take to the clinic. And when you bring someone into an office visit, you have the lower left-hand side, which is that you can assess this with like I said, a very cheap saliva sample that will cost maybe right now it’s a hundred dollars to do.
And then potentially, you at least have the option from the clinical side, to make decisions based on that genetic analysis. And so in this case you might have people with high risk for cardiovascular disease that you treat differently in the clinic then people with low genetic risk of cardiovascular disease. So are we comfortable with that so far? Genome Wide Association Studies. Hits, no hits. Places in the genome that have tall buildings. Taking that, and then amassing that information like this for knee pain, and smooshing it all together to create one score. Some people are going to be high on that score, some people low on that score. So that’s all okay so far?
Okay, so let’s move to social genomics. Where everything I just said you can do with not just knee pain, you can do with how far in school did you go.
And here’s what that looks like two years ago. This was in 2016, this study. So it’s essentially the knee pain study but instead of knee, it’s how far did you go to college?
And the study found 74 locations in the genome where if you have an A versus a T, a C versus a G, you’re more likely to go farther in school.
So this is an example of a complex trait, how far in school you go, that it has potentially some underlying genetic predispositions for it. And if you’re comfortable with the machinery so far, where you’re looking at a Manhattan plot and you’re looking for buildings, it’s the same machinery here. You were scanning the whole genome looking for places where differences in genetic, these letters, are related to this outcome. This outcome is years of schooling, instead of knee pain. So this was way back in 2016.
So whatever discussions that we’ll have in a minute, I’m just highlighting that they probably should’ve happened many years ago. This is now dated. The new version of this came out, the one that I just described was from 300,000 people, now it’s over a million people. And instead of 74 locations on the genome they’re at over 1200 locations in the genome.
So here’s the Manhattan plot for again how far in school did you go. And the same next step is possible here. Which is to create predictive polygenic scores for how far in school people will go. And what’s interesting here, in addition, is that instead of the clinician’s office being the next slide, The next slide is you can upload your DNA to the Internet, in this website in particular, and without a clinician’s oversight, you can get your polygenic scores back to you for years of schooling.
So now it’s both an expansion of the traits of potential interest, from knee, and urine and asparagus, and so on, health-related cardiovascular disease, to much broader outcomes. And, there’s no need to consult a doctor in any of this.
So that suggests, and as you might already anticipate, a lot of companies can come in and fill in gaps of potential interest. So here’s one that’s on the health side, no need for a physician here, There’s a company for $199 that can if you send in a saliva sample they’ll send you back a breast cancer risk. No need for any visit to the doctor. There’s broader companies that, same thing, if you’d like to send them your DNA they’ll “Unlock insights about health fitness, “even the wine you might prefer.”
Here’s another one from just a few months ago, this is Spotify the music service will create music playlists for you based on your DNA.
(audience laughs)
So it’s expanding. So let us now roll back to some a little bit more serious questions about potential policy impact. So the themes here are expansion of these large-scale Genome Wide Association Studies into not narrow health outcomes, but both broad health outcomes, but also into social domains like years of schooling.
And there’s a decentralization of knowledge. Meaning that there’s not a gate keeper called your clinician that is the only person you can get this information from. There’s direct lines to direct consumer companies that can provide whatever it is that you want in terms of predicting outcomes based on your genetic information. And I think one policy implication that will be a major theme here that is this allows a lot of new directions in discriminating against people, or for people, based on their genotype. That 10 years ago, 15 years ago, was just not allowed because the technology was not there. It was not cheap to sequence human DNA 20 years ago. We did not have all of these Manhattan plots to examine 15, 20 years ago. Now we do, so those technological barriers have been moved. And there’s no barriers in terms of having to interact with the medical establishment. Now I think there’s more serious questions about what areas in our life would we like to be discriminated for or against based on our DNA. Let me give you some examples.
So I think it’s a good idea maybe to start from the very small area in our life that you cannot be discriminated against based on your genetics, which is health insurers now in the US cannot legally discriminate against you or for you, for that matter, based on your genetics. So this is the GINA law from 2008.
Employers cannot discriminate against you based on your genetics. There’s no rest of that sentence. There’s no other areas in our life that I would start filling in that cannot discriminate against you based on your genetics. So that’s opened the question, do we want to fill in any other areas in our life where we do not want to be discriminated for or against based on your genetic code? And I also, so I’ll come back to this.
And then obviously,
as this information proliferates, there’s other areas in our life that we might not of first thought would be areas of genetic discrimination, that may be. So my co-author wrote this middle column in 2017 speculating what would happen if 23andMe which is a consumer DNA company, merged with online dating companies.
Well, and do we want to make that illegal or not? So of course that was then, that was way back in 2017 where he speculated, so of course that’s a long time ago, versus now, which of course that is exactly what is available now. Which is companies that are advertising matching you with a partner based on your genotype.
So that’s one area we can be for or against the use of genetics to discriminate, either for or against you. Just as an example, there are areas in your genome that put you at much higher risk for Alzheimer’s disease. As a 20 year old, would you like that in your matching profile?
And then the third area is maybe even the larger set of concerns, which is, just this leading question of what else might we want to predict. Not just about health outcomes that your health insurer might like to know, or your employer might like to know, but what about in our personal lives might we like to have more information about? And that’s getting back this first point that the same genetic code that you had when you’re a fetus, you have now. So what might we want to predict about a fetus, or a baby? So sex, eye color is very straight forward, hair color quite straight forward. Height, quite straight forward. Even maybe bigger domains that are a little bit more squishy like athleticism is predictable. IQ, predictable based on genetic information. Even something like facial features. You might think, well we could never do that. I’ll show you that people did that two years ago. But the question I’m raising now is what would we like to be available to be predicted? And what information would we like to be sent to parents, of either a pregnant woman or someone who just had their baby, when you have the baby with the heel prick in the hospital, would you like to have a genetic readout of all these things. ‘Cause it’s cheap.
We don’t do that now because we can’t technologically, we can technologically, the question is do we want to make any of these things off limits or not. And would we ever want to do that? So there’s one long held interest of predicting children’s characteristics, this is coming from 1939, the Doctor Seuss has a patent for this device which served the purpose of predicting the facial features of their children. So you take a picture of both of the couple, you overlay them, and then you give the parents that’s what your kid’s going to look like. So this one failed.
(audience laughs)
Right, it was not accurate. But we’ve changed the technology such that there’s a possibility to provide potential parents a different view that’s not from these overlapping pictures. As I mentioned a minute ago, so this is two years ago. This is a study in a scientific journal that used genetic information only to create computerized images of people’s faces and then compare those to their actual faces. So, one row is only based on As, and Cs, and Ts, and Gs of people’s genetic code, and the other row is their actual faces. And I’ll remind you that these As, and Cs, and Ts, and Gs are those letters that you had as a fetus. So when you ask would we ever be able to predict, or make predictions to parents about what their kids are going to look like, we could two years ago.
And facial features won’t obviously be the only thing that parents might care about. And there’s already an avenue that many of you I’m sure are familiar with, of examining very early in embryonic life potential genetic conditions where you might want to not implant embryo for example, because there’s some, maybe severe genetic condition. The same tools that one would use to genotype in day three a cell from an embryo, and get the As, and Cs, and Ts, and Gs, elicit those from the cell,
that’s the same information one could get then to ask well what’s that cell going to look like if allowed to develop?
And the issue to restate here is there’s essentially no legal limits in what information can be assessed in these early days. Should there be? That’s going to be I think the question to consider further.
And then directionally, I don’t think we’ve stopped at being– I don’t think we’re at the frontier of prediction and of large data sets. In fact, the future is much larger capacity, directionally, the capacity’s going to be to make better predictions, more accurate predictions, in any of the outcomes I just described. Example is the education one that I showed earlier, where in two years–
This is the first picture I showed you, there was 74 different findings. Two years later, tripling the sample size, there were 1200 findings. This is the direction that many outcomes are going. This is higher accuracy, larger data sets. And that then increases, directionally again, it increases in discrimination opportunities, both for and against people. The set of outcomes are expanding. The domain over which you would like to discriminate is expanding, it’s not contracting.
The possibility of private data becoming public, another possibility maybe, is understating the very high likelihood that private data becomes public. And then just ethical and legal and policy discussions around some of our comfort with precision medicine, which is to say precision medicine is part of using genetic information from a patient to make practice calls of which treatment you might assign that individual, or what course of therapy you might assign. What the same technology could be used to, the same steps could be used for, withholding or not withholding policies. Interventions that are outside the clinic. What sets of laws and discussions might we want to have for those. So let me outline a couple of recent events, some of them even a little dated, that I think maybe further concentrate our minds on the policy, legal and ethical questions here. And I’ll focus a little bit on privacy because you all know that there’s been massive data breaches in the last few years. One from credit card companies, that seems terrible. With severe implications of those that have had their data stolen. But the point here is that there’s an escalation with a breach of DNA data. Which is to say, when you’re release someone’s social security number and to their driver’s license information, and their address, that has some potential negative implications to that person. But what you’re releasing in general are attributes of a person they can change. One, most of the things your name, your address, even your social security number, your driver’s license, most of those things you can actually change. And more important, most of those elements are not very predictive about you and your Alzheimer’s risk. DNA, if it’s released to the public, your DNA if it’s released to the public, is predictive and it’s permanent. So it doesn’t have the features of a credit card hack so much, it has something that’s much scarier actually. Releasing your own Alzheimer’s risk is not something that you can change.
And it is predictive of your future outcomes.
Another subset of this privacy concern is how to think about and how to deal with personal decisions people make to contribute their DNA, and the implications to their biological family members of that decision. When you contribute your DNA, that information is also informative about your biological children, your biological parents, your other biological relatives, and their own Alzheimer’s risk, or their own other,
any risk related to genetic information. Here’s a recent report from the Journal of Science from just a few months ago, the main part is the last line which is the techniques they’re using that on one hand is used to capture serial killers from 30 years ago, which is to find not the serial killer’s DNA in a database, but the third cousin of the serial killer’s DNA in the database. And then find that serial killer through that familial search. But what this paper’s describing is because there’s so much DNA out there already, they’re talking about 1.3 million individuals that they had public access to their DNA, that the technique that they’re using through familial searches, the last line, could implicate, could find, nearly any US individual of European descent in the near future, in the US. Because we’re all related. And you only need your third cousin in this data set for you to be partially in the data set, is the point. And this is again directionally, is going to get worse, not better, as these data sets get bigger and bigger.
So we need to make proactive decisions about how these data can and cannot be used. Going back to the two areas in our lives where our data cannot be used against us, health insurers and employers. I’ll just remind you that several times in the last two years, this law was almost eliminated. So a year and a half ago one of the repeal and replace efforts of the ACA, part of that act was to eliminate part of GINA. So, meaning that it’s a fragile law.
And this is one of those cases where it lost by one vote. One vote, one way or the other, and we no longer have protection from health insurers or our employers in using genetic information to discriminate against employees or patients. Another end around, the protections we have, so this is just from, I guess now almost a year, employees being offered free genetic testing from their employers. So it’s not– Right? So what happens then is the employers don’t have you as a specific employee’s genetic data, but they have the aggregate of all of their employees to make decisions. So again, it’s a little bit of a funneling away of some of the protections. And the question I think on the table is whether we want to increase protections or reduce protections. And in what areas of our lives we want to increase protections or reduce protections. On the child’s side, or on the fetal set of questions of what information you would want about your child. A new DNA test, now this is new being a year ago, is with no interaction with your doctor. This is $650. You can get direct to consumer a readout of 190 diseases in a newborn’s genetic code.
So some of these larger questions are on privacy about what framework we want to have guiding who owns the DNA that you contribute.
And how, as I brought up a minute ago, how to weigh individuals’ interest in getting their ancestry reports, or getting their reports on their health risk
versus the information that they’re contributing about their biological family members. We have other questions that are on the table about who has access to these technologies. So in England there’s a recent effort by their national health system to provide free genetic testing to anyone who wants it. The caveat being that if you say yes, then you give your data away to the national health system. But that’s the trade-off. Anyone in the country can have a free genetic test, a free human genomic sequence, but the trade-off is you’re providing that data to the national health system. Representation, that’s a much longer conversation. I’ll just start it by saying that people from three countries have contributed, are behind, over 70% of all genetic findings. I wonder if you can think about what those three countries might be?
(audience murmuring)
So the US, UK, and I thought the one I was going to get you with was Iceland. Iceland, that tiny little Island of 300,000 people is one of the main contributors of all the knowledge of human genetics we know. Because the whole country’s been sequenced in one single data set, and it’s underneath a lot of these Genome Wide Association Studies. So that is to say that over 80% of all of the people who are in all these studies are of European ancestry.
There’s very limited representation of any other ancestral group.
Discrimination, what interactions would we like protected. So the first line is what our current protections are, health insurance and employers, and the other ones are just– You can have a long list of where you might like protections or might not like protections. Schools, K-12, universities. Would we like admission decisions partly based on genetics? Criminal justice. Long-term care insurance is not part of health insurance protections. Long-term care providers can discriminate against you based on your genetics. Car insurers could, and so on. So that could be a much longer sentence. Even in clinical decisions, how comfortable are we with allowing clinical decisions based on genetics? That is how comfortable are we with precision medicine in general? And to the extent we are comfortable with that, are we also comfortable with the same kind of analysis done for other interventions, like educational interventions in schools? Where would we draw the lines? So these are all open questions. And then what information should we shield or allow to potential parents about the genetic status of their embryo? So we have a limited amount of information that can be shared now, maybe about Down syndrome, about a certain narrow set of genetic conditions. But there’s no law now that says that a provider couldn’t also provide genetic predictions of a fetus’ IQ.
Wisconsin is leading the way in some of these questions. We were one of six clusters at the university level that were approved to bring in new faculty from the central campus, from the provost office, and from WARF actually, to become a hub of interest with new faculty that will work with some of the ones that I’ve listed here who are current faculty, on some of these questions. So I think that’s at least a good direction for the university to be supporting. And I’ll conclude by acknowledging some of the financial support I’ve received for some of this research. I’m very appreciative of. And give you some of my information for follow-ups and then open it up for Q and A.
(audience applauds)
Search University Place Episodes
Related Stories from PBS Wisconsin's Blog

Donate to sign up. Activate and sign in to Passport. It's that easy to help PBS Wisconsin serve your community through media that educates, inspires, and entertains.
Make your membership gift today
Only for new users: Activate Passport using your code or email address
Already a member?
Look up my account
Need some help? Go to FAQ or visit PBS Passport Help
Need help accessing PBS Wisconsin anywhere?
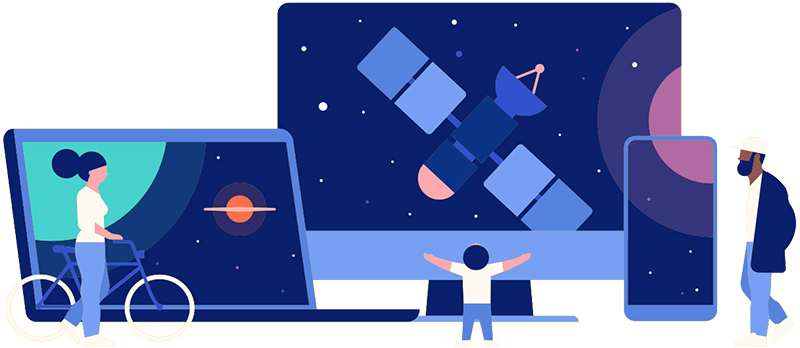
Online Access | Platform & Device Access | Cable or Satellite Access | Over-The-Air Access
Visit Access Guide
Need help accessing PBS Wisconsin anywhere?
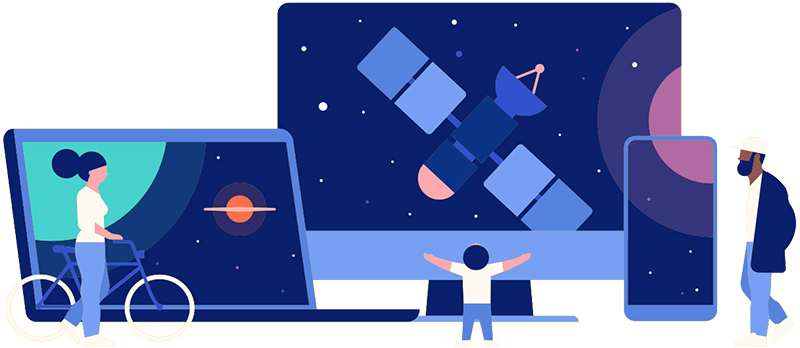
Visit Our
Live TV Access Guide
Online AccessPlatform & Device Access
Cable or Satellite Access
Over-The-Air Access
Visit Access Guide
Follow Us