– Welcome everyone to Wednesday Night at the Lab. I’m Tom Zinnen. I work here at the UW-Madison Biotechnology Center. I also work for UW-Extension Cooperative Extension and on behalf of those folks and our other co-organizers, Wisconsin Public Television, Wisconsin Public Radio, Wisconsin Alumni Association, and the UW-Madison Science Alliance, thanks again for coming to Wednesday Night at the Lab. We do this every Wednesday night, 50 times a year. And today, is the anniversary of our 12th year of doing Wednesday Night at the Lab. Next week, we start our 13th year, so thanks for coming out every Wednesday night. I appreciate it. Tonight, I’m delighted to be able to introduce to you Mutlu Ozdogan. He’s with the Forest and Wildlife Ecology Department. He was born in Ka-tyr-a-da, Turkey. And I am fluent in Turkish. [audience chuckles] And that’s also where he went to High School.
He went to Istanbul University and he corrected me as I was writing down the word, In-stan-bul. He said, “There’s no N in In-stan-bul”. Well, there’s not two Ns. And for my entire life, I’ve been pronouncing it “In-stan-bul”. This is what we call, adult learning. [audience chuckles] That’s where he got his undergraduate degree in Earth Science. Then he went to North Carolina State University to get his Master’s degree. Got his PhD. in geography at Boston University. Then he post-doc’d at this little place you may have heard of called, NASA. Ten years ago, 2007, he came here to UW-Madison. Tonight he gets to talk to us about mapping the world’s croplands with this great view from up above. Not just a bird’s eye view, but a satellite’s view. Please join me in welcoming, Mutlu Ozdogan, to Wednesday Night at the Lab. [applauding]
– Thank you, Tom.
– Thank you very much.
– Is this hot I think? Well, thank you so much for coming, I really enjoy these events. I think it’s a great service that the University provides. This is my second Wednesday Night at the Lab event, and back then, you know, in the first one, I talked about looking at forests. Now, I’m gonna talk about looking at croplands. Before I start my presentation, I wanna ask this question of why agriculture is important. And I’m sure people have different views about that, but these are some of my views over here. Obviously, agriculture provides food and nutrition. You know that’s something that’s the stuff that we eat. But many people around the world, agriculture is also a livelihood, right? There’s a picture of someone trying to extract some sort of yield from the field so that they can sell that and then make money off of it. Increasingly, the agricultural areas are used for fuel for growing bio-fuel products and there’s a lot of research going on, on this campus. And I would also argue that agriculture is sort of this tradition, which is associated food, and obviously economy. You know, we’re in the Midwest. Around here agriculture plays a big role on the economy.
And it turns out that all of those things are, in my view, interlinked. So, if I put the cropland area’s defined as sort of where crops are grown, a variety of crops, all of these things that I just talked about are interlinked. Right? So the food is related to cropland industry, nutrition, climate. How sort of cropland areas regulates the climate that is sort of happening above that. And then water obviously population, economy. So there’s a lot of sort of linkages that agriculture brings together. Not only that, but as our population grows, and you know, we reach somewhere around nine or 10 billion people, we do have to grow more food, but believe it or not, Earth is running out of space in terms of growing food. We’re actually busting at seams in terms of the best agricultural land, ’cause we already found those agricultural land around the globe and we’re cultivating them. And if you wanna grow more food, we either have to increase the sort of per unit area yield, which we’re working on, or we need to expand agricultural areas. But the only places to expand are places like the Amazon forest, the South American pristine grasslands, and so on and so forth.
And not only that, but agriculture also has this sort of interesting balancing act. This is a slide that I stole from the World Resources Institute. So, not only do we have to sort of increase the food, and also help people bring livelihood out of agriculture, but also we need to do that without wreaking havoc to this planet. Agriculture has sort of huge environmental footprint. Whether that’s removing the existing sort of natural areas for to make pay for agriculture, or to intensive agriculture that uses a lot of inputs, like pesticides and nutrient inputs that we see around here. So, I stole these slides from a colleague of mine, Jon Foley, who was here in the past, and he talks about how croplands are prominent across the planet and covering roughly, about 40% of the planet. And not only that, but to grow that much food, we also need to use a lotta water. So I’m trying to get at this theme of agriculture is great. It provides all those services, but it also comes at a big cost.
So the nutrient cost and the water cost, and I’m sure everybody knows this great story of the Aral Sea, as you can see in this satellite, in the last 30 years how withdrawal of water from the Aral Sea for irrigating agricultural areas has led to the diminishing of that area. So, agriculture is a big user of water and globally about 80% of global fresh water resources is used for agriculture. So the joke that I have with my students often, is that those of them who take long showers, that’s okay. You’re not the culprit, agriculture is, with respect to water usage. Now, I think I’ve tried to sort of convince you that agriculture’s important and it also comes with some environmental footprint. Now, even though we know that and that’s a fact, we have very little knowledge of agriculture at certain parts of the globe. Now if you look at the Midwest or the US or Canada, and perhaps Europe or Australia, we have very good knowledge of where agriculture is and what kind of crops are being grown and their average yield value, so that we can sit down and do some sort of interesting analysis. But there are parts of the world where agricultural information is sorely lacking, and I would count Africa, India, and parts of southeast Asia in there. So our knowledge of global croplands, I would argue, is regional.
Not globally fully complete. The second argument I would make is that many of the agriculture, global agriculture data collection agencies, like the United Nations and food and agricultural organization depend on individual countries to report their agricultural areas. And those reports are often what I would call subjective reports, because they don’t necessarily depend on any objective assessment. And the planet is changing really fast, so we’re cultivating more cropland. We’re changing the types of crops that we’re growing in an existing cropland area, so changes happen really fast. And I would also argue that more and more environmental impact analysis associated with agriculture require maps or data sets that are spatially explicit, and accurate, and up-to-date that we don’t have everywhere. And finally, these data sets, if we can get at these data sets that could help us shape the future. So with that, what I’m gonna do is, I’m gonna pose the second question of what is the role of remote sensing? Now for those of you who are not familiar with remote sensing, the text book definition of remote sensing is acquisition of information about an object without physical contacts. Just the fact that you guys are watching this presentation and forming some opinions is actually a form of remote sensing.
So with respect to satellite remote sensing, what we do is we build these equipment, they’re basically souped up cameras, and we put them in satellite platforms and we launch them in space. And as they circle around the planet, they take a variety of pictures in variety of different colors. And those colors include all the visible colors that we all can see, but also colors like the infrared, the short-wave infrared, long-wave infrared and thermal-infrared. And it turns out that many vegetation studies that we conduct are very sensitive to the colors that are built into those cameras. Now there are lots of additional benefits of using satellite observations in the form of remote sensing that is large area coverage, objective observations, because we’re not relying on a person’s opinion but rather the opinion of light. A physical quantity. There are, as I said, spectral capabilities that we can see beyond the visible color the human eye can see. And there is often wall-to-wall coverage. There’s data anywhere within the planet even in the inaccessible places, going back 40 years, so we can do historical analysis.
And these data sets are very accurately located in terms of their geospatial location. So that allows us to ability to acquire a lot of information in a very short time. Now even though remote sensing has these skills and has been around for a long time. And these are some of the different agricultural examples that remote sensing can give you from different parts of the world, from the Midwest US to China, to India at different scales. Remote sensing has not been very operationally used for agricultural assessment. And there are many reasons for that. Even though one of the very first Earth observation satellites were launched because of agriculture, people immediately realized that there were a lot of challenges as well. So some of those challenges are mismatch between the needs and the deliverables. What I mean by that is if you go and talk with farmers, they wanna see their own field everyday with a certain wavelength.
And it turns out that one of the early satellites that were built, simply were not capable of delivering that kind of information. Now, both the public and private organizations are addressing that issue, but that has been a challenge. And then satellites however, have been designed for this multi-purpose design. Engineers haven’t built these satellites just to look at agriculture. They built the same satellites to look at forestry, to water, to urban areas, and et cetera. So there isn’t the ag-specific remote sensing tools or assets that are out there. And then, we actually don’t have the ability to look at every variable. So for example, if you ask me to tell you how much fertilizer a farmer puts on their field, using satellite data, that’s pretty much impossible. Maybe we’ll get there in the near future, but we’re not there right now.
And there has been sort of traditional cost and processing associated with this, especially with large volumes of data. So, remote sensing has been around for a while, but there has been some challenges. But, in the last five to 10 years, there also has been a lot of opportunities coming our way. And I’m happy to report that because that’s sort of job security for me. [audience chuckles] So, and some of these developments are related to computation and some of it is related to changes in policies of how data are delivered. So for example, when I started using remote sensing, 15, 17 years ago, you could only purchase a single tile of image that over, let’s say Madison, and maybe at one-time period, and you would have to pay 2,000 or 3,000 dollars for that. And that’s the only data you got. So the data volumes and prices were somewhat, they would inhibit the analysis. Nowadays, we can look at the entire planet and we can look at the, download the entire archive of last 40 years of data and then process that.
But that requires additional processing. So some of the opportunities that I’ve been seeing and that I’ve been employing in my own lab are the ability to process large volumes of data. The second part is, there is this very active and growing open source community. There has been a lot of smart people, and I’m not one of them in terms of computer sciences, have been developing these really interesting tools that are directly applicable to satellite data. And then, there’s also a lot of active community out there that is providing science to open source community and we’re doing some of that at this university. Let me skip that. So these are some of the data tools that I’m gonna show you results of in a few minutes, but these are the kind of tools that we’ve been using. So what I wanna do is the title of this research, this talk is “Observing Crops From Space,” so what I wanna do is, with that introduction, give you some examples of how we observe crops from space. And I’m gonna do that in sort of three different contexts.
The first one is, we’re gonna look at the entire globe, and figure out where crops are, and we’re gonna try to do that at various high spatial resolution, if you will. The second one is, trying to map crop types, specific crop types, corn, soy bean, wheat, in large areas. And I’ll show you examples of that. And the third one which is more dear to my heart, is trying to get at the crop yields at the field scale. So, if you look at Dane County for example, and let’s assume that there are 1,000 corn fields in Dane County, wouldn’t it be nice to look at and estimate yields of every one of those fields and then use that information in a variety of ways. So let me start with the first one. So, about four years ago, we had a project funded by NASA. And NASA has a program called Measures, and Measures, you know NASA loves acronyms and Measure is also an acronym. And the idea of a Measures program is that they wanted to fund researchers that would utilize satellite data, mostly NASA data, and other forms of data to provide products, or produce products, map products, for societal good.
So we submitted a proposal, and we got a proposal accepted. And the idea of that proposal was to map global croplands at different scales and regions at 30-meter spatial resolution. And those of you who are not metric, 30 meters would be about 30 yards, so each pixel would see a little less than a tenth of an acre. So imagine dividing the entire Earth into less than tenth of an acre plots, and then trying to map whether there is an agriculture or not agriculture in that particular plot for the entire global landmass. We called that product GFSAD30, so Global Food Security Support Analysis Data at 30 meters. A mouth full. And we’ve been leveraging this idea of cloud computing because of just sheer volume of data, and those data sets are being delivered right now as we speak. The whole goal of that project was to produce four map products. The very first product was to simply tell people whether a particular piece of land was cultivated or not cultivated.
So a binary mask, if you will. Now once we derived that particular map, then the next idea was to, sort of, driving this map of cropping intensity. Now, cropping intensity, now in this sense, simply means the number of cycles, growing cycles within a calendar year, or within a growing season. Obviously around here, our growing cycles are only one because we have a short growing season. We can only fit in one crop barely, if you will, around here. But there are places around the world where you can grow two, three, sometimes four crops. So this second product is looking at the number of vegetation cycles, number of growth cycles of agriculture in that particular parcel of land. The third one, which is probably a more difficult product is a crop type map, in which we would tell you what is the dominant crop type in that particular parcel of land that I’ve talked about? And I use the word, dominant. That’s because every year, crops parcels change.
So around here, if I was to create a label for a parcel of land, that label would look something like corn soy dominated. And in North Dakota, it might be wheat soy dominated. And in parts of India, it might be rice dominated agriculture, because of their changing nature of agriculture. And final product that we were interested in driving that we’re delivering right now is this idea of irrigated versus non-irrigated. So it’s taking a look at the same parcel of land and tell you whether that piece of land receives irrigation or not, and there are some abilities in remote sense satellite data that would allow us to detect whether that piece of land is being artificially irrigated or not. And the reason that’s really important is because, as I said, globally 80% of fresh water resources are used for irrigation, and only about 18% of global agricultural area is irrigated. But the yields in those locations are really high. So for this presentation, I’m only going to show you the examples of the very first product up there. And then some examples of the third product in a later part of the presentation.
Now the way that we approached this problem is we had a large team, and each of those team members had experience, geographic experience, in different parts of the world. So a team took North America. Another team looked at South America. My team looked at Europe. You know, I’m from Turkey, so I’ve been in that area quite often, so we know that area well. We took Turkey, Middle East, and Central Asia. And then other groups have looked at different parts of the world, and what we did is we used slightly different computer algorithms to drive the maps that I was telling you about, and simply stitched them together to make a global map. And then delivered that to public resources that you and anybody else can download. The way that we approached this problem is by taking satellite data.
So this is a mosaic of a particular satellite data over Africa, shown in what we call a false color composite. And false color composite simply means any place we see a red color would be actively vegetated. That’s why it’s called false color. And this is actually a term that is left over from the World War Two. Then we could take those indices data and turn them into vegetation indices. So, in this case, the more green it is, the more actual vigorous green it is. But not only that, but we could also take additional variables, like topography, slope, and et cetera, and then bring all of these into a computer machine learning algorithm in which you provide examples of what cropland looks like, and simply computer finds very similar examples for you. But instead of doing it for one pixel or one location, it does it for billions of billions of locations in those little parcels that I talked about. Now I don’t know if you can see it, but if you look at the middle part of that very left picture, you can actually being to see little square boxes.
I don’t know if you can see that. And the reason you are able to see those is because those are the individual tiles of satellite image that is being collected with the satellites that we’re using. So in this case, we have to take many of them, in this case, literally tens of thousands of them, and then clean them for clouds and other issues, and stitch them together to make these mosaics so that they can be used in an image classification algorithm. Now the type of classification algorithms we use were what’s called a supervised classification algorithm in which an analyst, like myself, would have to provide examples of what a cropland looks like to a computer environment. And those are not that easy, especially when you consider a global agriculture, because global agriculture is highly variable. And how do you get the sample? So we reached out to global organizations like the FAO, the United Nations, and other organizations that helped us collect literally thousands of on the ground examples of what agriculture looks like, so that we can take those examples, and then feed them into a smart computer algorithm so that those algorithms could recognize what agriculture looks like. And obviously, that is really different across different parts of the world. In the Midwest, agriculture looks very different than what it looks like in China or in India for example. So that was a great effort.
I would say we spent half of our time just doing that, instead of processing satellite data. So these are some examples. This is an example from Australia just within the continent of Australia or country of Australia, there is a lot of variation. Everything from rain fed single crop wheat to rain fed single crop lupine to canola and et cetera. And this is within a single landscape. And if you wanna map agriculture in this landscape, you would have to need to know all of these different sort of character or characterization of agriculture. Similar things in Africa? So Africa was even a more difficult case, because in Africa, there isn’t a whole lot of commercial agriculture. There are parts of Africa that have commercial agriculture, like South Africa, Egypt and northern part, and some in Kenya. But there are other parts of Africa where agriculture is just sort of a very transient in livelihood.
And then how do you collect sample so our team members from the UN and FAO has been instrumental in getting to the field and collecting those samples for us. Now once we took those samples and then ran them through a computer algorithm, along with our satellite data, this is the kind of map that we produced. So you can go to that website and you can download and you can look at these. I think it is also mobile able. But all the green, this bright green areas show the places where global agriculture is. So what I want to do is, so this is the first product that I was talking about earlier. So what I wanna do is, I wanna take a few places around this global map and show you examples of detail product. So here’s an example from Bulgaria. So it’s right there, for those of you who don’t know where Bulgaria is, right there.
So, here is what a global Google Earth image background would look like. Here are some mountains with dark forests, and then this is where the agriculture is. And our computer algorithm capture where agricultural is and not capturing anything that is forest or urban or roads and et cetera. So that’s one example from Bulgaria, sort of a temperate location, if you will. Here’s an example from Kingdom of Saudi Arabia. So Saudi Arabia 15, 20 years ago, decided that because of the ground water resources that they have that they were gonna grow a lot of wheat and then export that wheat. What a crazy idea. So this is one of their big project areas. So all of those are large scale irrigation projects, and our product captures accurately those.
And I would say that those are easy examples in Kingdom of Saudi Arabia. Then here is an example in Vietnam, where here is the river. This is in the Mekong Delta area, here is an urban area. There are some roads, and there is a lot of rice cultivation between those areas. So you can do this yourself. You can go it, to that particular website. You can look at it on a mobile phone or iPad or a computer, but those maps exist. And we are very proud that we were able to produce products like these that are of service to a variety of organizations. Now, when I teach remote sensing, I tell my students to promise me two things.
And those are, never take a map from someone without quantitatively assessing its accuracy, ’cause anybody can make a map. Right? Making an accurate map is important. And the second promise is never deliver a map without first assessing its accuracy and delivering that product with that accuracy number. So we spent a whole lotta time validating our map, and validation simply occurs by going to different places or extracting samples that are independent of the training samples, and simply trying to figure out how much of those samples that are on the landscape have been accurately mapped by the algorithm. So here is a sample data set. So we developed some mobile tools to look at very high resolution imagery on an iPhone or on a mobile device, and then simply put a dot in the middle of it to figure out whether this is agriculture or not. And we’ve done that over thousands of locations, and in some locations we had access to other data sets. That’s why there’s this important break over here. And then we compared the maps that we made against those, and I’m happy to report that on a global average, that particular map is about 90% accurate. Now that’s a global average number.
There are places where the map is 99% accurate, and there are places where the map is only 60% accurate. That’s just the nature of agriculture and how it exists in that location and how remote the sense data may or may not be able to pick it up in that particular location. I can tell you that in the Midwestern United States around here, mapping agriculture is not that easy. And that’s because we have large fields. So agriculture is really established and you can really see in the remote sensing signal what is agriculture and what is not. But if you look at other places like China, or India, parts of India where we didn’t a place as big as this room, there’s probably five different land cover types, including several trees, animals, humans, a couple of houses. Agriculture becomes a really complex variable to determine. In those areas, map fails to deliver at that sort of 90% accuracy. So these are just examples of those apps that I’ve told you about.
Another way of doing validation is to compare our product to other products. So in the beginning of this talk, I mentioned that even though there are existing products out there, they’re not always accurate, or they’re not always capturing the type of agriculture that we’re talking about. So this is a map that simply shows three different products. So here is a background image. This is in southeast part of Egyptian delta. So here’s agriculture, here’s non-agriculture, and here’s some agriculture over here. This is a global product that has been produced 10 years ago at 10-kilometer resolution. So that’s a pretty coarse resolution data set. And here is a map that has been produced at 250-meter resolution.
And here is a map that has been produced at 30-meter resolution. So as you can see, even though they’re all showing agriculture because of the sort of spatial resolution issues, you don’t necessarily get the right area, or the right location. Now the reason I’m showing this, is because many of the global aid agencies, whether it’s USAID or World Bank or FAO, has been traditionally relying on maps that are made from those, because those were the only available products at scale that those organizations could use. And they were extremely excited to see the type of products that we’re producing at extremely fine detail. The second topic I wanna cover is refining the maps that I described on a more categorical level. So, in addition to mapping a parcel, as being either agriculture or not agriculture, the next level of information is what is being grown in that particular parcel of land. Now that we know that it’s agriculture, but what is really cultivated with traditionally, or predominately? So I wanna show you examples of that, and then that’s a slightly different problem. I would say it’s a bigger problem. And the reason for that is two-fold.
One is as the information that we require from satellites becomes more refined, like going from agriculture versus not agriculture, to what type of agriculture it is, the signal that exists in satellite data declines. That’s because if I was to show you corn and soybean, in a satellite image in a particular time of the year, you couldn’t really distinguish them from each other. That’s because at that particular time instance, they are both green, and we don’t necessarily see the leaves, as I showed you some of the spatial resolution issues, you can’t really separate them. So we need to have these additional variables in our possession to be able to separate those crops. And it turns out that to be able to separate individual crops from each other, the temporal information becomes the most important piece of information. But that means that when you process the data, you have to access this time series of data, starting from the beginning of planting, all the way to harvest, for all the crops that you’re interested in a particular land. So one example around here, for example, is separating corn from soybean. As you know, one of them is planted a couple of weeks earlier, and it grows slightly faster, and it peaks at a vegetation that it’s particular time. One is earlier than the other one, and the senescence a bit earlier.
So if you have a time series data that captures that small difference, couple of week difference, you would obviously separate corn from soybean. But what happens if a farmer decides to plant his or her crop two weeks later? Then in that case, they become somewhat indistinguishable, and those are the kind of issues that you would run into. So, this is a different image processing or satellite processing problem. And the idea over here is to convert these satellite observations, here is an example of what a true color composite, where you can see individual fields, into a map like this that shows where the individual crops are, and then simply separate them from each other. And as I indicated, the way that we do that is to assemble this time series of data. So, we have observations that are roughly observed about a week apart. So anywhere on the planet, we can get an observation on a week apart. Now when I say week apart, we get a potential for observation today. And then another potential for observation about seven or eight days from today.
Now that doesn’t mean the observation will be made. That’s because Earth is a cloudy place. And especially around here in the summertime, you get a lot of clouds. So there might be times in Madison, where you don’t see the ground at least with the type of satellite data that we use, for a month. So that means you have this entire month of data gap in your observation, and what if that’s the time of separation of corn from soybeans? So what we always try to do, is we’re always trying to find ways to fill these gaps, either by using additional data sets, which may have different characteristics themselves, or try to fill them with additional gap filling methods that are more rooted in mathematics and computer sciences. But at the end of the day, our goal is to build this time series and apply that. Now a few minutes ago, I said mapping crop types, it has two problems. One is assembling the time series. The other problem is how do you get training and validation data? Now I could give all of you a mobile app and I would be highly certain that all of you can tell me whether you’re looking at an agriculture land or not agriculture land.
But it becomes much more difficult to tell me if it’s corn or soybean, just by looking at the satellite data. So even though we had 100,000 samples for mapping crop, non-cropland, we only had 1,000 samples for knowing where crop types are, in terms of a global sample. So there’s this multiple fold decrease in both validation and training data that we could fit into our algorithm. So, I’m gonna show you some examples from Dane County because we all know where Dane County is. So here is a product that we didn’t generate, and I’m showing this just as the example of what’s possible out there. This is a product that has been generated by the US Department of Agriculture. And here is a crop type map for Madison and Dane County, and this is a crop type map for year 2015. And here is year 2016, and obviously, if you go back and forth, the last years corn areas became soy and last year’s soy areas became corn. That’s not always the case, but that’s the most of the case around here.
So the reason you’re able to do things like that in a place like Dane County is because there are known patterns that we can extract data from and then map them. Now try doing that in Asia, or in India, or China where there is no specific rotation pattern, or there’s very little ground related information. Anyway, that was 2015, 2016 and here’s your 2017 data that just came out. Now, for certain places around the world, we had really good data, and because some of our partners were actually from India, and they were able to provide the ground data so that we can feed that ground data into our computer algorithms and map crop types. And not only crop types, but also whether they’re irrigated or double crop or single crop, crop type. So I’m just gonna read some of these for you. So here is an irrigated ground water irrigated rice, maze, and chick pea color, wherever that color is right here, for example, that parcel of land has that kind of attribute. And obviously, in order to determine that fine scale or fine categorical detail, you would have to know a whole lot of information about the ground and without our Indian partners, this would’ve been impossible. So the message I’m trying to give over here is that we are able to make maps like these, but those maps will vary highly in their quality depending on how much ground data that we would have access to, and how much satellite signal, or how easy it is in the satellite signal to define that particular crop type.
So, in India, we are able to do this. In Europe, we are able to do this. In China, unfortunately, we are not able to do this for a variety of reasons. If I was to show you that same map in a more simple manner, you could also take that map and simply turn it into irrigated versus non-irrigated agriculture. And because India is the number two global irrigator in the world, and those are the places in India where you can see irrigated croplands in green, and non-irrigated croplands in brown or red. So, again in places where you have this data, ground data, we are able to map them, but that’s not always possible everywhere on the planet. We have examples from Bangladesh as well. So this is an example of different rice types from Bangladesh, and we had partners in Bangladesh that gave us great support. So one of the jokes that I have with people that I meet, and maybe I can do that with you, is that if you travel somewhere, tell us what kind of crops you’re seeing.
I’ll give you my email at the end of this talk, so that we can utilize that data to make a better map. Or if you know people in other countries. The last example I wanna show you, which I’m really excited to share is, this idea of yield assessment. And when I say yield assessment, I’m particularly talking about getting at actual crop yields on a field by field basis. As I indicated earlier, what if we had thousand corn and soybean fields in Dane County, and what if you were able to produce yield data for every one of them? Now obviously, farmers who are farming that land, know exactly what kind of yields they have. But that information is in private hands and government would like to access it, or other organizations would like to access it for a variety of reasons, but it’s simply not publicly available. What if we had the ability, or produce the ability to get at these idea of yield data? And the way that we have been approaching this problem, and I think we’re somewhat successful, but it doesn’t work everywhere, and I want to show you some of that. And the way that we approach this problem is we look at satellite data. Here is a landscape with corn and soy bean data.
So different colors refer to different crop types in the raw satellite data. And we can take that and turn into a crop type map. So green is soybean. Yellow is corn. And then, using the time series data that I showed you earlier, we can take the satellite data and turn it into a biophysical variable. And biophysical variable in this case is a variable called LAI or Leaf Area Index, and is simply defines how much green leaf area a particular plot of land would have. So if I was to go to a highly vigor cornfield, for example, and simply cut all the leaves of the corn and lay them down in a one square meter area, and if I fill that one square area one time, Leaf Area Index would be one. And if I fill it with twice, Leaf Area Index would be two, so on and so forth. So with the time series data, we could convert these satellite observations into these biophysical variables that obviously have biophysical meaning, and yield is a biophysical variable that we would have to get at.
And we use a simple formula to do that and that formula looks something like this. So yield is equal to some Leaf Area Index times the amount of solar radiation. So you can think of these plants in a basically a sort of solar panels. They have these leaves. They’re absorbing all the radiation and they’re converting that solar radiation through photosynthesis into biomass. And at some point, that green biomass becomes grain yield, and then you can allocate part of that photosynthesis into grain yield. And the beauty of this particular approach that was put forth 40 years ago is that it nicely lends itself to remote sensing because one of these variables is actually, this particular variable is the variable that we can get from remote sensing. So, we take these satellite data for every pixel or every field in there, we assemble a time series and then that time series variable becomes the biophysical variable. And then we can simply accumulate the amount of photosynthesis that the crop is going through, and then simply accumulate that and through some simple conversion, turn that into a crop yield.
So, here is an example from Dane County. So this map is for corn-planted fields. So every one of these little polygons is a corn field, judged to be corn field in the year 2003. So if you’re a farmer and if your field is here, you can point to it. Right? And this is the yield data that we produced for that particular year. So sorry, this is in metric. So 13 tons would be about 210 bushels per acre. Pretty good yields. So as you can see, there is this wide variety of yields that you can get in a Dane County. Now obviously, producing a map like that, as I said earlier, is easy.
Anybody can produce that, but how good is it? Right? It’s an important question. So, one of the ways that we can assess how good that map is, is to compare it to what the government USDA estimates are. So, because we have the entire population of every field in Dane County, we can produce histograms. I love histograms. Right? So, we can produce histogram and that histogram would look something like that, it has an interesting shape. Somewhat bell shaped, but it’s not exactly. And then, on top of it, we could plot the USDA estimate for that year, which is this red bar over here. So what this particular analysis is telling me is that we are somewhat over estimating yields on the average value, but it’s not that bad. So I think it’s a good start. So one of things that I’m really excited about doing is to expand this kind of technology to the entire Midwest.
Imagine doing this for entire southern Wisconsin where croplands are important, or Illinois or Iowa for that matter. And then, produce that information and then perhaps compare them to government estimates, and then somehow use that information for a variety of ways, including environmental assessment, so on and so forth. So, what I wanna do is conclude by saying agriculture has great data needs. It’s important, but it also has data needs, and I think remote sensing can address some of these needs, but not all of them. And it might be able to do some of these data needs really well in some locations, but not well in other locations. And there are challenges remain, but I think we’re overcoming them because of computation power that we have, and then there are a lot of exciting developments that offer a number of solutions. And I think we’re doing our part at this University to address some of those challenges. So, here’s my email address as I promised. So if you travel somewhere and see interesting crops, let us know what you’re seeing.
But we need to know where they are. With that, I’ll thank you and take questions. [audience clapping]
Search University Place Episodes
Related Stories from PBS Wisconsin's Blog

Donate to sign up. Activate and sign in to Passport. It's that easy to help PBS Wisconsin serve your community through media that educates, inspires, and entertains.
Make your membership gift today
Only for new users: Activate Passport using your code or email address
Already a member?
Look up my account
Need some help? Go to FAQ or visit PBS Passport Help
Need help accessing PBS Wisconsin anywhere?
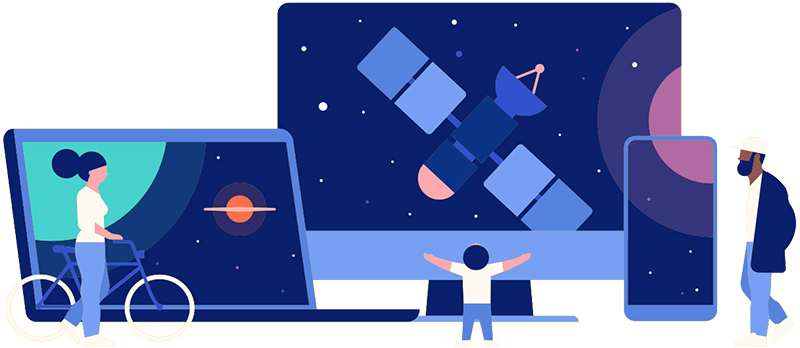
Online Access | Platform & Device Access | Cable or Satellite Access | Over-The-Air Access
Visit Access Guide
Need help accessing PBS Wisconsin anywhere?
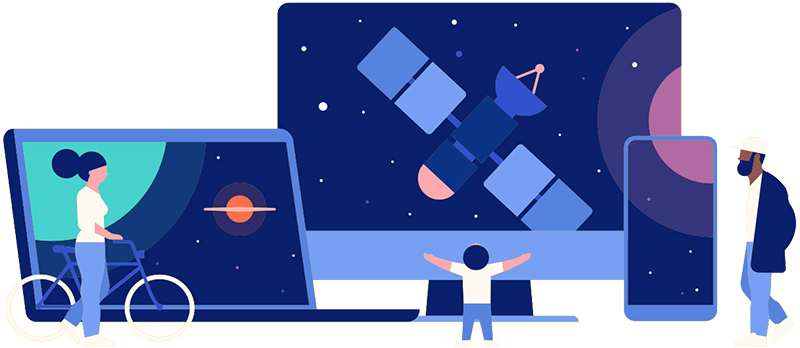
Visit Our
Live TV Access Guide
Online AccessPlatform & Device Access
Cable or Satellite Access
Over-The-Air Access
Visit Access Guide
Follow Us