[Tom Zinnen, Outreach Specialist, Biotechnology Center, University of Wisconsin-Madison]
Welcome, everyone, to Wednesday Nite @ the Lab. I’m Tom Zinnen, I work here at the U.W-Madison Biotechnology Center. I also work for U.W.-Extension Cooperative Extension, and on behalf of those folks and our other co-organizers, Wisconsin Public Television, the Wisconsin Alumni Association, and the U.W.-Madison Science Alliance, thanks again for coming to Wednesday Nite @ the Lab. We do this every Wednesday night, 50 times a year.
Tonight, it’s my pleasure to introduce to you Steve Carpenter. He’s a professor in the Center for Limnology in the Department of Zoology. He was born in Kansas City, Missouri. On the Missouri side. He went to high school in Bethesda, Maryland, where he went to Walter Johnson High School. And, as he pointed out to me, that’s probably the only high school that he knows of that’s named for a major league pitcher.
He got his undergraduate degree at Amherst College, and then came here for his master’s and PhD in botany, here at U.W.-Madison, then he spent 10 years at that university called Notre Dame du Lac, otherwise known to most of us as Notre Dame. But since he’s a limnologist, you gotta throw in there that du Lac. In 1989, he came back to U.W.-Madison, where he’s been ever since. Tonight, he’s gonna be talking with us about early warnings of big changes in complex systems. Please join me in welcoming Steve Carpenter to Wednesday Nite @ the Lab.
[applause]
[Stephen Carpenter, Director, Center for Limnology, University of Wisconsin-Madison]
Well, thanks, everyone, for coming out on – on a night that looks stormy, and thank you, Tom, for that nice introduction. I welcome the chance to talk with you today about some research that I’ve been involved in for about the past 15 years about very large changes in ecosystems. An ecosystem is a place on Earth, and all the animals and plants that live there, along with their abiotic environment. And, as you’ll see in a minute, sometimes those change in a very big way fairly quickly, and we’ve been trying to figure out how that works and if there are ever warnings of it.
So, let’s start with a familiar example.
[slide titled – Toledo Water Crisis 2014 – featuring a screenshot of the webpage for The Blade newspaper with images from their water crisis stories]
In 2011, Lake Erie experienced the largest bloom of toxic algae in history. The limnologists who were studying Lake Erie said, You know, this is a harbinger of things to come, and they were right. In 2014, an even larger algae bloom, again, of toxic cyanobacteria, more about those in a – in a few minutes, emerged on Lake Erie, and shut down the water supply for 300,000 people. It made national and international news. The Toledo Blade did a terrific job of covering it, and if you go their website, you see a lot of articles about it. It was a major environmental problem, and ultimately, a very expensive one for the city of – of Toledo. So, that’s an example of a big change –
[Stephen Carpenter, on-camera]
– in an ecosystem, in this case, the ecosystem being Lake Erie.
Lakes do this all the time, and in southern Wisconsin, we see big algae blooms frequently. They usually occur in July, August, maybe early September, so we’ll start to see them in the news any day now. Lakes can also be in a very clear state –
[slide titled – Big Changes in Ecosystems – featuring two photos under the heading – Lakes – one of a boat reflected in a clear lake and one of a lake full of algae blooms]
– where you could lower a dinner plate into the water a long, long way and – and still see it. An example of big alternative configurations of that ecosystem.
[the slide animates on a new heading below Lakes, titled – Coral Reefs – featuring two more photos, one of a clear healthy coral reef and one of a dead reef]
Coral reefs are another example that’s been in the news recently. We think of coral reefs as this tourist attraction with beautiful fish and ornate corals, but they can suffer massive mortality events. Sometimes those are due to nutrient inputs –
[Stephen Carpenter, on-camera]
– sometimes those are due to invading species, and sometimes those are due to warm water. Just in the last month, the Great Barrier Reef in Australia has suffered the largest bleaching event in history, and a massive amount of corals in Australia have died, particularly in the northern part of the reef, which is the northern stretch of it. And the scientists there think we’re looking at decades to hundreds of years for recovery of that major tourist attraction for Australia.
And they’re also known from rangelands very well. Rangelands have –
[return to the Big Changes in Ecosystems slide now with a new heading – Rangelands – featuring two more photos, one of a grassy rangeland and one of a rangeland cover with shrubs]
– two configurations. One is fairly grassy, shown at the top, and that’s a really good situation if you’re trying to raise sheep or cattle. They can also be covered with shrubs, as in the picture at the bottom, and that’s a really bad setup if you’re trying to raise sheep and –
[[Stephen Carpenter, on-camera]
– and cattle, because sheep and cattle don’t get much out of that forage. Goats maybe would, but – but not sheep and cattle.
What goes on is, if you overgraze the rangeland in a dry year, you eliminate the grass and it can no longer carry fire, and fire is needed to kill off the shrubs and keep them from getting a toehold. So, overgrazing combined with a drought, even a short drought, can switch the ecosystem from grassy to shrubby, and its economic value is down the drain. The only way to reverse it at that point is with a D9 Caterpillar, and that can be costly.
Scientists have developed many mathematical models for how these sorts of large changes in ecosystems occur. And, of course, the details of those models are intricate, and –
[slide featuring two illustrations, on the left are three graphs on top of one another with conditions on the x-axis and system state on the y-axis – the first graph labelled a has a straight diagonal line from the upper left to the lower right with two arrows – one on top and one on the bottom both pointing towards the diagonal line; the second graph labelled b has the same arrows but instead of being straight the line from upper left to lower right is slightly curved in the middle; the final graph labelled c has a large curved backwards S shape in the middle for the line that moves from upper left to lower right; additionally, there are now double sets of arrows pointing towards the line throughout its length. The second illustration is a three dimensional representation of the graphs on the left with small circles representing perturbations in the system states that lead to the differing shapes of the line]
– highly dependent on the ecosystem that – that – that is under study, but they all have some common features, and I’m just gonna show you some of the common features right now.
The – we think about the state of the system. So, for example, is the water turbid or clear, or is the grassland grassy or shrubby, or is the coral reef in good shape or has it died off? We think about driving conditions, such as the nutrient input to a lake, or the grazing intensity on the grassland, or whether there are lots of algae overgrowing the coral. And in the models, you can calculate something called a potential surface, and I’m not gonna go into how you do the calculation, but I’ve shown five examples of them here in gray. So, in this upper potential surface, we’ve really only got one valley, and the ecosystem represented by the ball is sitting in that one valley. And as we move to this second potential surface, changing the conditions down on this axis here, now, all of a sudden, we have two valleys, and the ecosystem can be in either one. We call that a critical transition because a different set of stable points has appeared. So, we now have two stable points where we used to have one. And the ecosystem, as it turns out, will spend most of its time in the deepest of the – of – of the valleys.
Then in the – I’m having a little trouble; there we go. Waking up my pointer here; its sleeping – in – in the third one, now the new valley has deepened, actually, so the ecosystem will spend even more time over there. In the fourth frame, it’s deepened even more, and, actually, the situation in the original valley is getting quite precarious. And in this last panel, the original valley has completely disappeared, and the ecosystem has moved over here. So, if this is, say, the shrubby state, and we started in the grassy state, or if this is the turbid lake, and we started in the clear lake, we’ve now undergone a very massive change in the ecosystem. And this general pattern of changing valleys and –
[Stephen Carpenter, on-camera]
– the ecosystem moving from valley to valley in a potential surface occurs in all the models of this type of process.
Another important word that I’m gonna use again and again is resilience. Resilience is a measure of how deep the valley is. So, a very deep valley is a resilient one. The ecosystem is not gonna leave there very easily. A low resilience valley is a very shallow one, where a little push could knock the ecosystem out of the valley. I’m hovering, now, over a –
[return to the previous slide with the two graph illustrations with the mouse pointer over the fourth illustration where the new ecosystem is in a very deep valley and the old ecosystem is in a very shallow valley]
– very low resilience ecosystem. So, if we want to predict one of these changes, the key lies in figuring out a way to measure the resilience of the valley where the ecosystem sits and determine if –
[Stephen Carpenter, on-camera]
– that valley is losing its resilience. So, I’m gonna talk about measuring resilience in the slides to come.
Are you all with me?
Okay. This is – Ive – I’ve only got a couple of really technical diagrams. This is a very important one.
Okay, so, the question we started to ask in the early 2000s is, are there generic indicators of –
[slide titled – Generic Indicators of Declining Resilience – featuring two subheadings – Specific Indicators – Monitor the drivers and conditions that lead to big changes; Drivers and conditions may apply to a particular ecosystem – and Generic Indicators – Statistical patterns the emerge before big changes in many kinds of ecosystems. Additionally, at the bottom of the slide are four headshot photos of the researchers involved along with their names – Marten Scheffer, William Buz Brock, Egbert van Nes, and Vasilis Dakos]
– declining resilience? Ecosystem managers all over the world worry about these alternate states that ecosystems can be in, because up until about 15 years ago, we thought there were no good ways of predicting the transition between states, so they generally came as surprises, and often, they were very expensive surprises. We’re now beginning to get a clue that – that – that there are various ways of predicting these traditions – these transitions. By specifics – I’m not gonna talk very much about specific indicators, but I – I – I think those are fairly straightforward to understand. The idea is to monitor the drivers and conditions that lead to big changes and point out that the ecosystem is at risk.
For example, I don’t really need a very sophisticated indicator –
[Stephen Carpenter, on-camera]
– of algae blooms in Lake Mendota, because there are 40,000 cows out there, and those cows have been dumping manure on the ground and in the streams all winter long, and I’m pretty sure that as soon as we get some big thunderstorms, that manure is going into Lake Mendota, and it’s gonna turn green. That’s a very specific indicator. I can’t generalize that to a rangeland or a coral reef, and maybe I can’t even generalize it to another lake, but I’ve – Ive kinda got Lake Mendota nailed, because I know what’s causing that – that green water out there.
By a generic indicator –
[return to the – Generic Indicators of Declining Resilience? – slide featuring the headshot photos of the four researchers and the definitions of specific and generic indicators]
– I mean a statistical pattern that emerges before big changes in many kinds of complex systems. So, if you understood a generic indicator –
[Stephen Carpenter, on-camera]
– you could go to a rangeland, a coral reef, a forest, a lake, an ocean, and you could estimate its resilience. So, I’m asking, are there generic things that – that – that we could measure?
I got started on this – this work with the folks at the bottom of the slide.
[return to the – Generic Indicators of Declining Resilience? – slide featuring the headshot photos of the four researchers and the definitions of specific and generic indicators]
Martin Scheffer is one of the world’s most famous theoretical ecologists, and he is deservedly famous for the terrific work he’s done on alternate states in a wide range of ecosystems over about 30 years. Buz Brock is a mathematical economist here at U.W.-Madison, he’s one of the top nonlinear econometricians in the world, and he got interested in this pro-problem and – and was a key member of – of the group. Egbert van Nes is a physicist in Holland. Vasilis Dakos is a theoretical ecologist from Greece who now lives in Switzerland. And the – the group of us have worked off and on, usually meeting in a nice –
[Stephen Carpenter, on-camera]
– place for a few days on – on – on this problem since the early 2000s.
So, to – to – kind – to focus our thinking about generic indicators a little bit, let me give you another example, and a very dramatic example of –
[slide featuring a photo of a lone camel in the Sahara Desert of shifting sand dunes]
– alternate states in ecosystems. The Sahara Desert right now is a vast area of sand with the occasional camel, an oasis here and there, and – and – and a few migratory people, Bedouins, but it’s a pretty dry place.
[the slide animates on a photo of a hippopotamus over the top of the photo of the camel in the desert]
A few thousand years ago, it was a vast wetland with rivers and lakes all over it, hippopotamus, a very moist area, dramatically different from the way it was today. And what went on –
[new slide featuring two photos, the same photo of the hippopotamus in the lower right, and a photo of a dust storm from the Sahara Desert over the Eastern Atlantic Ocean taken from a satellite on the left. Additionally, on the upper right is a graph with Years Before 2000 on the x-axis and Dust Deposition on the y-axis and showing a slight increase in dust deposits over time]
– there? We know a lot about the Yahara (sic) [Sahara], because we can measure the dust that fell into the Atlantic Ocean for thousands and thousands of years. So, the left-hand panel there shows the outline of Africa. This is a satellite image, and you can see a billowing dust storm out here of dust blowing out from northern Africa over the Atlantic. It sinks in the ocean, some of it sinks in the ocean. And you can go out and core the ocean and reconstruct the dust deposition rate for thousands of years. And between about 9,000 and 5,700 years ago, it was a pretty low dust deposition rate. And that’s one of the reasons that we knew – know we were dealing with a very wet environment in the Sahara, because if – if it was dry, there would be a lot of dust moving around. Now, if you were that – this –
[Stephen Carpenter, on-camera]
– hippopotamus sitting there, and you were a scientifically inclined hippopotamus, you might look at that data and think, this ecosystem is staying wet. The – there is not much trend there in the dust deposition, and it – if I had to predict the dust level for the next thousand years, I’d say it’s gonna be between 40 and 50, which is – is a low number. So, I’m feeling pretty good, if I’m the hippopotamus.
But here’s what actually happened. Between –
[slide featuring the same graph with Years Before 2000 on the x-axis and Dust Deposition on the y-axis and now with the years 5,700 to 5,000 years before 2000 highlighted in blue and showing a huge increase in dust deposits during that time that continue up until 2000 on the top of the slide and the two photos of the hippopotamus and the camel on the bottom left and right, respectively]
– about 5,000 and 5,700 years ago, there was a marked increase in dust deposition, and we now know from many palaeoecological studies that that period of a few hundred years marked a rapid transition in the moisture regime of the Yahara – of the Sahara –
[Stephen Carpenter, on-camera]
– region. So, it moved from relatively wet to relatively dry in a fairly short period of time. The point I wanna make is that simply by looking at that time series from 9,000 to 5,700 –
[return to the previous slide with the graph and the two photos]
– years ago, you wouldn’t really have guessed that this was gonna happen, and that this would become the new normal.
This is the kind of situation we’d like to have a generic indicator for.
[new slide with eight graphs on it, the two of particular attention are at the top of the slide and marked a – High resilience and b – Low resilience. Slide a shows an environment in a deep valley where outside pressures have little effect on moving the environment up the hill to change it to a new system state. Slide b shows an environment where the valley is extremely shallow and outside pressures are extremely likely to move it over the small bump to a new system state. Under graph labelled a there are three graphs labelled c, d, and g – graphs c and d have Time on the x-axis and System State on the y-axis and graph c shows that if there is a perturbation at a certain time in a highly resilient environment that environment recovers quickly, or shown another way in graph d it is a very short waveform that is centered on the graph over time; in graph g the x-axis is the Environmental State (t) and y-axis is a Future Environmental State (t+1) and the results show a bunching in the middle of a diagonal line drawn from the bottom left to the upper right. Under graph labelled b there are three graphs labelled e, f, and h – graphs e and f have Time on the x-axis and System State on the y-axis and graph e shows that if there is a perturbation at a certain time in a low resilience environment that environment recovers very slowly, or shown another way in graph f it has very long waveforms that very widely on the graph over time; in graph h the x-axis is the Environmental State (t) and y-axis is a Future Environmental State (t+1) and the results show an even distribution along a diagonal line drawn from the bottom left to the upper right. ]
This is my second fairly technical slide that you have to pay attention to. There are some other data slides you can ignore, but this is another one you have to pay attention to, and it has to do with the behavior of the ecosystem in its valley, in cases where the valley is highly resilient or not very resilient.
In the case where the valley is highly resilient, on the left, the valley’s pretty deep. If we perturb the ecosystem a lot, really whack it and drive it up the side of the valley, it’s gonna snap back pretty fast to where it was. It’s not gonna take its time to come back. And it will – it turns out that if you expose the ball to small shocks for a long period of time, you get a very constrained variability, like I’m hovering over now. It also turns out that if you try to predict next year from this year, so, you try to predict the state in year – in year T plus one from the state at year T, you don’t get a very good prediction. All the data sort of cluster in a ball here and theyre not giving – so, knowing conditions this year doesn’t really help you very much in knowing conditions next year. You know you’re somewhere in the ball, but that’s about it.
Contrast that with the situation in this low resilience ecosystem over here. So, now, the valley is pretty flat. And – and, in fact, we know the ecosystem is pretty precarious. It – it could fairly easily be tipped over into the more resilient valley. But let’s think about resilience in this low resilience valley. Now, if we perturb the ecosystem by driving it a long way up the slope, it’s gonna take much longer to come back. Its recovery time will be longer. If we look at the variability over time, we see these wide spring – swings, and it’s sort of sticky. So, it swings up somewhere, gets stuck for a little while, then it swings somewhere else, gets stuck for a while. Very different pattern over here than we saw over here in the variability. And we can measure statistics such as the variance or the standard deviation, which I’ll use later in the talk. Those are just ways of measuring how variable it – it is. We can measure that variability.
Down here, we see that if we want to predict next year from this year, we can do pretty well, actually. We’ve got a pretty tight relationship between this year and next year. That’s called autocorrelation. That means the system has memory, or it’s sticky, as I mentioned a few –
[Stephen Carpenter, on-camera]
– moments ago. It doesn’t change very quickly. So, if you know the state at a given time, you know quite a lot about what the state of the system will be the next time. For example, if the system is right here, I know it’s gonna be right about there next time. So, it’s relatively predictable.
That can be measured, too. This – this – this – this phenomenon, this second one that I mentioned, is called autocorrelation, there’s a simple statistic for measuring that. So, we can measure the variability and the autocorrelation, and if those are going up, resilience is going –
[Female audience member, of-camera]
Down.
[Stephen Carpenter, on-camera]
Down, you got it, right. If those are going down, if autocorrelation’s going down, and variance is going down, resilience is going –
[Several audience members, off-camera]
Up.
[Stephen Carpenter, on-camera]
Up, right. So, we’ve got a tool now. We’ve got something we can measure to work with resilience in these complex systems.
This looks complicated –
[slide featuring five graphs two on Cyanobacteria and three on Yeast. The first Cyanobacteria slide features Time in days on the x-axis and Extinction on the y-axis and showing 6 perturbations labelled p1-p6 and showing very little movement along the graph line at each perturbation. The second Cyanobacteria graph has Increasing Light Intensity on the x-axis and Recovery Rate on the y-axis and shows a linear result from upper left to lower right for each perturbation. The Yeast graphs have Dilution factor on the x-axis and Population Density on the y-axis and the top graph shows a stable fixed point along the perturbations along the top until they hit a certain dilution and then the line curves back in the opposite direction along an unstable fixed point. The second Yeast graph is labelled Autocorrelation time and shows a stable line across the bottom until it hits a dilution factor of 1,000 then it jumps up sharply; the third Yeast graph is labelled standard deviation and shows a steady uptick as the dilution factor is raised]
– but I’m gonna give you the bottom line very quickly. These are two laboratory experiments, highly replicated, very carefully conducted laboratory experiments that test the theory that I just showed you. In the experiment on the left, cyanobacteria, the toxic algae that I was talking about a minute ago, are exposed to little shocks in the nutrient level that they receive at different levels of light extinction. And, as these cyanobacteria are very sensitive to light, so when the light intensity goes up, they do not do as well. And the experiment measured the recovery rate, in a – in a – that’s how long it takes to come back from a perturbation, as a function of light intensity, and it went down. And here, at point six, this point right here, they collapsed. They didn’t come back at all. So, this experiment shows the decrease in recovery rate that I talked about just a few minutes ago in a very carefully controlled laboratory experiment.
The right-hand experiment is one with yeast, and these yeast, in this case, actually have two stable states. One is a high concentration of yeast, and the other is when they’re extinct. So, they’re – theyre gone there. That’s a really bad alternate state that we all should try to stay out of. But nonetheless, the researchers were able to measure resilience indicators as they moved along this curve. And they knew a lot about the yeast, so they knew a lot about the growth. And they measured the autocorrelation, and dilution factor is the stress. So, as dilution factor goes up, eventually, the yeast collapse to the extinct stable state. And the autocorrelation time, which is just a – an exponential transform of the autocorrelation, goes up. So, autocorrelation goes up as we approach the collapse point, exactly as we said it would. Resilience got low; autocorrelation goes up. And standard deviation, which is a measure of variability, standard deviation is just the square root of – of the variance. It goes up. So, this experiment also corroborates the theory. It says that as autocorrelation and variance go up, resilience goes down, even to the point of causing this yeast population to disappear.
So, it’s just –
[Stephen Carpenter, on-camera]
– experimental evidence for the theory I gave you a minute ago. Okay?
Okay.
Alright, now I’m finally back to the kinds of ecosystems I like to work on, which is big ones. And so, if this theory is gonna be any good, we have to be able to use it in real world ecosystems to measure resilience, and to do that, a research team of –
[slide titled – Can We Measure Changing Resilience in Real-World Ecosystems? – featuring six photographs, one an aerial photo of the lakes upon which the research was done, one a photograph of the Field Team that did the research and then four photos of the researchers involved – Jon Cole, Mike Pace, Jim Kitchell, and Jim Hodgson]
– lake collaborators helped me out in designing an experiment to test the theory, and some of these people are my very oldest friends. John Cole and I were undergraduates together, we worked in the same lab when we were sophomores. Jim Kitchell was on our – on my PhD committee. Mike Pace and Jim Hodgson are long-term friends. Mike is at University of Virginia, Hodgson at St. Norbert College in De Pere. Jim Kitchell is retired from U.W.-Madison. John Cole is retired from the Ecosystem Center in New York.
We worked on some lakes here in northern Wisconsin, there’s our crew there in that –
[new slide titled – Whole-Lake Experiment – Trophic Cascade as a Test Case – featuring two photos and two diagrams. On the left are the two photos stacked on top of one another, one an aerial shot of lakes in Northern Wisconsin and one of the teams research boats out on one of the lakes. The two diagrams are a visual representation of the Trophic Cascade each with four panels and read from left to right – in the first illustrated diagram the last panel shows that there are no large fish (as a red circle with a line through it), which leads to an increase in smaller fish represented an illustration of numerous small fish and an arrow pointing up, this leads to fewer zooplankton which is represented by a few illustrated zooplankton and an arrow pointing down, which leads to an increase in algae which is shown by numerous algae illustrations and an arrow pointing up. In the second situation, the far left has an illustration of a large fish, which leads to a decrease (down arrow) in the number of small fish (the small fish illustrations numbers 2 now), and this leads to an increase (up arrow) in zooplankton (with a plethora of illustrated zooplankton now in this panel), which leads to a decrease (down arrow) in algae (with an illustration of very few algae)]
– team picture.
The basic idea was to use something called the trophic cascade as a test case. Kitchell and I discovered the trophic cascade in the early 1980s, and published a number of papers about it, and – and we have figured out how it works pretty well, and we know it’s a critical transition. And the – the two states of the lake are shown in the diagram here. In one case, you have a lot of large, big, veracious predatory fish, that’s the bottom case. And I’m trying to, there we go. So, we’ve got a lot of these big guys. They eat, or in some cases terrorize, the little guys, and – and so, they leave. The little guys normally would eat these crustacean zooplankton, particularly Daphnia. Those are little animals about this big that live in the lake. But if they’re terrified or absent, they can’t eat the Daphnia, and the Daphnia eat the algae, and when there’s a lot of Daphnia, there are not very many algae. That’s called a trophic cascade, ’cause it goes bang, bang, bang, bang, down the trophic levels of – of the lake.
The opposite state is a state where heavy fishing pressure has driven out the big fish. So, the big fish numbers are reduced to very low, or maybe they’re absent. In that case, you get a lot of the little fish. They quickly wipe out the Daphnia and other big zooplankton, and you get lots of algae. We know this is a critical transition, and we thought we could use it –
[Stephen Carpenter, on-camera]
– to test the theory in a – in a lake.
The first thing we do before we do any of these experiments is we do a lot of computer modeling, and we – in the case of the trophic cascade, we had a really good computer model that’d we’d been honing for more than 20 years at the time we did the experiments. And the – what I’ve shown diagrammatically –
[slide titled – Simulation – Control of Planktivore by Predation – featuring two graphs; the top graph has 0 to 1 in tenths on the x-axis and two factors on the y-axis, planktivore (in pink) and piscivore (in blue) and additionally showing a large fish at the number 1 and small fish at zero on the top of the graph – the graph shows inverse relationship between fish and plankton. The second graph still has 0 to 1 in tenths on the x-axis and the standard deviation (in green) and the autocorrelation (in red) graphed on the y-axes and showing a bell curve for the autocorrelation of phytoplankton and a modified bell curve with a dip in the middle for the standard deviation of the phytoplankton]
– here is the transition in the fish from big fish to little fish. And the point of it is that the standard deviation and the autocorrelation measured in the phytoplankton, so, the phytoplankton are the little green guys floating in the water. You can actually measure changes in their autocorrelation and their standard deviation, in the model at least, as you get this transition from big fish to little fish or from little fish to big fish, and that’s important, because measuring fish populations is really, really hard work. You would think it would be fun because –
[Stephen Carpenter, on-camera]
– it’s fishing, but you have to catch zillions and zillions and zillions of fish –
[laughter]
– and it gets tedious after a while, and the statistics are not very good. We can just get robots and put them on buoys and stick them in the lake, and they measure the phytoplankton every five minutes, and we don’t really have to do anything except go download the data every once in a while. So, it’s – its really great that the phytoplankton can be the sentinel for us.
We did the experiment in this set –
[slide titled – Experimental Lakes – featuring an aerial photo of the lakes involved – Peter, Paul and Tuesday – as well as an illustration of the two lakes with illustrated large fish on the left hand of the illustration and illustrated small fish on the right side of the illustration]
– of experimental lakes, I’m gonna talk mainly about Peter and Paul. Paul is a – a reference lake, or control. We call it a reference lake because it’s like a reference electrode in electrochemistry, if you ever took electrochemistry. For our purposes, you can remember Paul is small and rhymes with control. Sort of.
[laughter]
And Peter is the manipulated lake, and Peter started out with a lot of little fish, and we gradually added big fish. Just a few at a time. We didn’t just chuck a bunch of them in there. We’d get a couple, put them in, see what happened, get a couple –
[Stephen Carpenter, on-camera]
– put them in, see what happened, and monitor the behavior of the little fish to see if they’re getting scared.
This summarizes the experimental design in a little more detail.
[slide titled – Whole-lake predator manipulation – gradually add top predators (largemouth bass) – featuring an illustrated table with Trophic Group Metrics in the 4 rows and Start Year 1 and End Year 4 as the two columns. At the start of Year one there were Less Than 20 adult largemouth bass, the Catch Per Unit of Effort (CPUE) for small fish was approximately 15, the mean size of the phytoplankton was .4mm, and the level of Chlorophyll was greater than the reference lake. At the end of Year 4, the number of largemouth bass was greater than 100, the CPUE of small fish was around 0, the mean size of the phytoplankton was 1.0mm, and the level of Chlorophyll was equal to the reference lake]
We went from less than 20 adults of largemouth bass in Peter Lake to more than a hundred. CPUE is catch per unit effort. That means we were getting 15 little fish per minnow trap per hour, and that dropped to essentially zero. It – it first dropped because they were terrorized, and then it dropped ’cause the bass ate em all. So, they really had a good reason to be scared.
[laughter]
The zooplankton went from pretty little, .4 millimeters to one millimeter. And it’s really size of the zooplankton – its body size, more than biomass – that determines how much grazing they do. When we started the experiment, the chlorophyll, which is a measure of phytoplankton, was much greater in the manipulated lake than the reference lake. At the end of year four they were about the same. So, the trophic cascade worked in –
[Stephen Carpenter, on-camera]
– in this experiment, and the indicators worked too. So, here I’ve shown you, this is actually the four years, so, A is –
[slide titled – Example – Variance of Phytoplankton Biomass (Chlorophyll) – featuring four graphs (one for each year of the experiment, labelled A-D), each with Variance of Chlorophyll on the y-axis and day of year (DOY) on the x-axis and featuring a blue line for the Reference Lake and a red line for the Manipulated Lake. The graphs show a decrease in the amount of Chlorophyll as the number of largemouth bass increase culminating about halfway through year 3 (Graph C) when the amount of Chlorophyll in the Reference Lake and the Manipulated Lake become equal. This point is labelled Regime Shift by a line through that years graph at that point]
– 2008, B is 2009, C is 2010, D is 2011. And what I’ve plotted here is the variance of chlorophyll. This is the phytoplankton variance in Paul, the reference lake, and Peter, the manipulated lake. You can see that it began to go up pretty consistently here in the second year. And in the third year, it was just screamingly high. So, we had an –
[the slide animates on a box that covers the last of Graph B (Year 2) and half of Graph C (Year 3) that is labelled Early Warning]
– early warning of the regime shift. By – by day 220 of the third year, essentially, the minnows were either gone or completely terrorized clear up in the bog mat. The zooplankton had switched, and the phytoplankton had switched. So, the regime shift was over by day 220 of the third year, but we were getting these warnings more than a year in advance.
So, if we had only –
[Stephen Carpenter, on-camera]
– been measuring variance, and, by the way, autocorrelation had essentially the same pattern, we would have known that the shift was – was coming. And, of course, this is an experiment, we were making the shift, so we weren’t surprised. The – the point wasn’t that we could make a shift, we knew we could make a shift. The point was, would we see the indicators in the manipulated lake and not in the reference lake? And we did. So, that’s pretty cool. So, it worked.
The next thing you might say is, So what? The – I mean, suppose you can measure these indicators. What if you were managing the lake and you changed the way you managed it when you detected the indicators? And would the lake recover? Would it go back the way it was before, or is it committed to collapse by you – the time you detect the indicators? Maybe the thing is going off the cliff anyway by the time you can discern the change. And in that case, the indicators aren’t really very worthwhile. So, that was the next question we asked –
[slide titled – Can Resilience Indicators Provide Warning to Avoid Bad Outcomes in Real Ecosystems? – featuring a graph with time on the x-axis and system variance on the y-axis and plotting three indicators – Water Quality (green), Distance to Threshold (blue), and Indicator (red). The graph shows the Water Quality as constant over time, the Distance to Threshold staying fairly steady early on and then dipping down rapidly at the threshold, and the Indicator as also fairly stable over time until right before the threshold where it then suddenly ticks up]
– is could we actually recover a lake once the indicators fired off? So, if you could imagine a situation where you’re measuring water quality, water quality is doing just fine. You’re measuring an indicator, and the indicator is starting to squeal, and that indicator is related to the resilience or the distance to a threshold for collapse, the – the depth of the valley. So, the valley is getting small – smaller, the indicator is firing off, water quality looks fine so far. Could –
[the graph animates on a line titled – Intervention – right at the point the Indicator goes up and the Distance to Threshold starts going down which results in the Water Quality dipping a little and then returning to its even state resulting in the Distance to Threshold going back up and the Indicator going back down. The Question – Does this work? is posed at the bottom of the slide]
– you intervene? Could you go in and do something about it and maintain the water quality, or is the ecosystem committed to falling off the cliff? That’s what we wanted –
[new slide titled – Can We Detect and Prevent Algae Blooms? – featuring three photos – one of a researchers hand holding up an algae bloom, one of the 2015 Field Team doing the research, and one of the three lead researchers, Stephen Carpenter, Jon Cole, and Mike Pace]
– to find out. We switched to algae blooms for this experiment because they’re a lot easier to deal with than – than fish. With fish, youre – in the trophic cascade, you’re keeping track of four trophic levels. I already mentioned it’s difficult to census fish. And we had discovered that the phytoplankton –
[Stephen Carpenter, on-camera]
– worked so well, we thought, Let’s just focus on the phytoplankton. And we knew we could make algae blooms with nutrients. We – we dont even have – we can skip the cows. Unlike Lake Mendota, we don’t even need a cow, we can just buy nitrogen and phosphorus and put it – put it in there.
This is the research team. My –
[return to the – Can We Detect and Prevent Algae Blooms? – slide featuring the photos of the researchers and Field Team]
– P.I. collaborators were Mike Pace and John Cole again, by then, Jim Hodgson and Jim Kitchell had retired, and there we are. You can see we’re a lot better coordinated for this second experiment.
[laughter]
We have matching T-shirts, and it really looks like a team, whereas we were pretty slovenly looking –
[Stephen Carpenter, on-camera]
– the – the first time.
As in the previous case with the trophic cascades, the first thing we did was we ran ecosystem models to find out if this was gonna work. In the case of algae blooms, there are a bunch of models –
[slide titled – Theory – Resilience Indicators for Harmful Algae Blooms – featuring a box labelled, Many models of blooms suggest critical transitions on the left and containing the following bibliography – Batt et. al., Theoretical Ecology, 2014; Beninca et. al., Am.Nat., 2011; Rosenzweig, Science, 1971; Scheffer et. al., CJFAS, 2000; Serizawa et. al., Ecological Modelling, 2009 and others. On the right, are three small graphs stacked from the Batt study that have Potassium on the x-axis and Unicells, Colonial, and Zooplankton on the y-axis, respectively and all showing a stable level of potassium until they all reach a certain point where the levels skyrocket. The statement – Rising nutrients (increasing carrying capacity K) lead to rising variance and autocorrelation before the bloom of colonies – in a box at the bottom of the slide]
– and they all suggest that the early warnings should – should work. So, we had a lot to choose from.
It’s really important to do this kind of modeling study. It – in this – in this case, it took a graduate student about six months to do the modeling study. The experiment took eight people, several years, and probably cost a million dollars. So, it’s definitely worth it to do this in silico before you go to a –
[new slide featuring a photo of the researchers on the lake at a testing station as well as a group of graphs showing the changing data over time and the statements – Data from sensors downloaded daily – at the top, that then has a large blue arrow pointing down to the next statement – Time series and statistics updated, in the middle, that then has another large blue arrow pointing down to the final statement – Early Warning? Send automated text or email. At the bottom right of the slide highlighted in a blue box is an example of the automated text – ***An alarm has sounded. Please check website*** New alarms were found for the following – Ar 1 of Chla_Conc_YSI in Tuesday. Alarms have repeated for the following indicators Ar 1 of DO_Conc_YSI in Peter (DOY=186)]
– real lake.
As before, we worked with the sensors. We had a little bit more sophisticated system this time. We downloaded the data from the sensors daily, updated the time series, and we also updated the indicator statistics. For this experiment, we needed an alarm. We needed to know when things – when the indicators were so strong that we should reverse the manipulation. And so, we used a method –
[Stephen Carpenter, on-camera]
– called quickest detection, which was developed during the Cold War to see when missiles are coming in. And it is an extremely sensitive statistical method for detecting a change in – in an indicator.
So, every day we calculated variance and autocorrelation of everything that our little robot measured, and we also calculated the quickest detection statistic to see if an alarm went off. And then the computer, if an alarm went off, the computer immediately texted us and sent us emails. So, your phone would go off, Uh-oh, I got a text. My buoy is calling, and my buoy said there’s something happening, look at the website, and the website is updated every day with all the data. So, we had really quick feedback, and even though we had people all over the continent, you know, the P.I.s were on the east coast, I – I was – I was here, we had some students up there. Everybody on the team got the warning, got the – got the information at the same time. We could get on Skype or get on the phone and figure out what we’re gonna do.
So first, let me show you the results of an experiment to test the indicators for –
[slide titled – 2014 – Study Design to Test Indicators – featuring two diagrams, one for the Manipulated Lake (Peter) and one for the Reference Lake (Paul). The first diagram for the Manipulated Lake shows the statement – Daily nutrients additions – on the far left and then a green arrow pointing to the next question – Warnings of an impending bloom? – and then another green arrow pointing the statement – Continue nutrient additions – and then another green arrow pointing to the question – Outcome – HAB (Algae Bloom). The diagram for the Reference Lake has the statement – No manipulation – on the far left and then a long, white arrow pointing to the far right, and the question – Outcome- No HAB (Algae Bloom)?]
– algae bloom. Again, we’re gonna use Paul as the reference lake, Peter as the manipulated lake. This is a pretty simple experiment. We’re gonna very slowly add nutrients, just a little bit a day, ’cause we don’t want to go into the bloom too fast. Measure for warnings, check to see if we have warnings, continue adding nutrients, and see if we get a bloom. So, this basically tests whether the warnings work at all for the –
[new slide titled – Cyanobacteria in 2014 – featuring three graphs and a photo, the first graph has the Day of the Year on the x-axis and the amount of Phycocyanin on the y-axis for the reference lake, the low color enriched and high color enriched; the second graph maps the day of the year on the x-axis and the standard deviation on the y-axis for the three factors; the third graph maps the day of the year on the x-axis and the autocorrelation on the y-axis for the three factors; the photograph is a picture of two beakers, one on the left that is filled with water with a high amount of algae and one on the right that is filled with clear water]
– algae bloom case. And it turns out they do. So, this is the data for 2014. Paul is in blue, Peter is in red, and there’s another experimental lake that Ill – I’m not gonna talk about due to time tonight in black. Let’s focus on the red one. And here are the statistics for standard deviation and autocorrelation. And they start firing off. The – the – the ball is an alarm. So, that’s the quickest detection statistics as a missile is coming, but, in this case, it’s not a missile, it’s a blue-green algae bloom. So, we started to get alarms around day 180 or so, which is late June, about now. And you can see that we had a big algae bloom, this is – this is large numbers, by about day 200, 220, something like that. So, the warnings went off 20 or maybe 40 days before the algae bloom, and then here I have some Erlenmeyer flasks. The clear water is from Paul, the turbid water is from Peter, so, yep, that’s an algae bloom. So, basically, the indicators worked again for this situation. Now, let’s see –
[new slide titled – 2015 – Treat and Halt Study Design – featuring the same diagrams for the Manipulated Lake (Peter) and the Reference Lake (Paul) as the prior study design slide]
– if we can halt the manipulation and get an effect. So, in 2015 now, we – this is the same experimental design diagram you saw a minute ago, but now, we’re gonna –
[the slide animates out the statement – Continue nutrient additions – at the third stage and replaces that statement with an illustrated man with his hand sticking out to halt along with a red circle with a line through it. A red box also animates around the final question – Outcome – HAB (Algae Bloom)]
– change a couple of things. So, we – if we’re gonna halt the manipulation when we get a warning, so, when the –
[Stephen Carpenter, on-camera]
– warning occurs, we’re gonna halt, and see if we get the harmful algae bloom or not.
HAB stands for harmful algae bloom. Sorry for that.
And so, the intervention decision depends on getting alarms in both phycocyanin, which is a pigment of cyanobacteria, it’s unique to cyanobacteria, and chlorophyll, which is a generic pigment, the one that I talked about a few – a few minutes ago.
So, we actually wrote down a priori the conditions for an alarm and programmed that into the computer that was monitoring the data so we couldn’t fudge it ourselves later. It – you know, the computer was telling us what we wrote down months before the experiment occurred.
So, here’s what happened in the first –
[slide featuring three graphs and a photo. The photo is an aerial shot of the two lakes Peter and Paul; the first graph is of the day of the year on the x-axis and the amount of Phycocyanin on the y-axis for the reference lake, the low color enriched and the high color enriched lakes; the second graph has the day of the year on the x-axis and the Phycocyanin Standard Deviation on the y-axis; the third graph has the day of the year on the x-axis and the Phycocyanin Autocorrelation on the y-axis]
– part of 2015 up to about day 180. Again, we’re looking at the red line. So, that’s Peter Lake. And you can see phycocyanin, which is the indicator of cyanobacteria, is going up at a pretty good clip. Chlorophyll was too. And the standard deviation, the measure of variability, is going up, and the autocorrelation is pretty high. It went up quickly and stayed pretty high. So, we’re getting some indicators here, and we’re getting some alarms, and we had them in chlorophyll too. So, we had basically met the criteria for pulling the plug, and we decided to end the nutrient addition on day 180, which is the 29th of June in that year. The air photo just shows what the lakes are looking like then, and you can see Peter’s beginning to green up. This is Peter over here; it’s beginning to green up pretty – pretty well.
So, what happened? You could imagine, you know, we’d already added a bunch of nutrients.
[Stephen Carpenter, on-camera]
These things are growing exponentially. Maybe they just kept going up. On the other hand, you could think, Well, we added all the nutrients we were gonna add, ’cause we stopped on day 180. Maybe they’re just gonna flat line, and they’ll just hang in there, ’cause we – we did add those nutrients and they’ll use them. Or maybe, they’re gonna go down. Maybe they have not crossed a threshold and the lake is gonna recover.
How many of you think they went up?
[wide shot of the audience with one or two hands in the air]
Okay, one, alright. How many – oh, two, alright.
How many think they flat lined?
[wide shot of audience with one or two hands in the air as well as Stephens]
That’s what I thought. Okay, a few.
How many think they went down?
[wide shot of the audience with few hands in the air]
Well, there’s a lot of people out there that didn’t vote.
[laughter]
You know, in Australia, you can be fined for not voting –
[laughter]
– so, we’re – were gonna be taking names.
They went down.
[slide featuring a larger version of the first graph in the previous slide with the days in which nutrient were added highlighted in green and then showing after nutrients were stopped being added after that date the amount of phycocyanin going back down. At the top of the slide are aerial photos of Lake Peter over time that show the amount of algae being reduced]
So, we – the green box there is the period of time we were adding nutrients, and literally the day after we stopped adding nutrients, they went down.
So, what happened here is we got these warnings, most of them around starting at day –
[Stephen Carpenter, on-camera]
– 160, and, actually, the warnings were complete by day 176. On day 180, we pulled the plug on the nutrients, and the water quality improved immediately. So, the early warning came soon enough to prevent the transition to the turbid state, the permanently turbid state. So, this is pretty encouraging, actually. I mean, maybe – maybe this could really work to – to – to do something in the world.
I think we have a couple of minutes, so I’m gonna tell you about another really cool development that’s very, very recent. I’ve been talking about variability in time, but what about variability in space? It would be really useful if variability in space could give us an early warning of a change, of a big change, in an ecosystem. The reason is that it’s very, very laborious to measure everything, every day, all the time. It takes a big crew, it takes a lot of gadgets, and the gadgets have to be completely reliable. They’ve got to function every five minutes for hundreds of days at a time, and that’s a difficult thing to do in the real world.
But if you could do it with space, maybe you could measure the spatial pattern just every once in a while. Maybe every couple of weeks or something like that which would be much less work. That just means you have to make your machine work once every two weeks, and you have the other 13 days to practice with it, you know, to hope that it works. Or maybe you could even measure it from a satellite or from an aircraft. I think that – I think that would be really, really great, although, we didn’t do that in this experiment.
Turns out that Emily Stanley –
[slide titled – Do Spatial Patterns Change Before Algae Bloom? – featuring four photos, one of Emily Stanley, the lead researcher, one of another researcher, Anders Uppgard, in a boat measuring algae with FLAMe with another team member, one of an aerial shot of Lakes Peter and Paul which are now covered by a FLAMe pigment map, and one of another researcher, Vince Butitta at his laptop]
– who’s another professor in the Center for Limnology, and her team have devised a gadget that can map the pigments in a lake in about an hour. And so, here you see Anders Uppgard, who did an undergrad thesis on this, driving a boat around, and Anders can just do this squiggly path in the lake and get a pretty good map of pigments in the lake, and we can calculate all sorts of variability statistics from those maps.
And Vince Butitta, who’s a master’s student with Emily, is the guy who did these calculations. So, it’s potentially a really neat tool.
[Stephen Carpenter, on-camera]
Once again, we have models that tell us how the pattern should change. What – what this diagram shows –
[slide titled – Hypothesis – Variability Increases Prior to the Bloom – featuring six graphs, labelled a-f, which have amount of nutrients on the y-axis and variability in space on the x-axis. The first graph is completely blue showing little to no nutrients and each subsequent map shows little swirls of green to show more nutrients being added until the final graph is completely green]
– is results of a model at increasing nutrients. So, top left is low nutrients, then they go up a little; Top middle, up a little more; top right, a little more; bottom left, it’s just like reading in – in a western language, a – a little more; bottom center; and – and – and the most, bottom right. And so, it starts out with pretty much no variability in space, and, actually, not much autocorrelation. Then it begins to develop more and more variability, and – and also more autocorrelation, until, actually, the variability and the correlation start to disappear. And over here, in the green state, there’s really low variability and autocorrelation.
So, actually, the variability and autocorrelation in space in this model change much the way they did in time, but in this case, we only sampled it six times instead – in over – in the model. Only six times over a very long period instead of every five minutes. But we had to do it in space.
[Stephen Carpenter, on-camera]
So, what happens when we do it in the real lakes? So now, Anders goes out, he drives the boats around, makes the squiggly lines. The – Vince takes the data, runs them through a bunch of statistics. So, what you’re seeing here –
[slide featuring a graph with Days of the Year on the x-axis and the Standard Deviation and amount of phycocyanin on the y-axes and the standard deviations of Lakes Peter and Paul as well as the amounts of phycocyanins graphed for each lake. The graph shows a large increase in the amount of phycocyanin and a high standard deviation in Lake Peter until day 175 where they both crash precipitously by day 190]
– in this panel, the Peter phycocyanin is the pink polygon. The Paul phycocyanin is a pale blue polygon that you can barely see, so Paul had hardly any in it. And you can see this bloom that you saw a couple of minutes ago at just before day 180. These are actually the spatial data, not the middle of the lake data, so they’re slightly displaced. The red is the standard deviation, a measure of variability, now in space, and – and the blue is the standard deviation in Paul Lake. So, you can see that the standard deviation went up and got pretty high, and then came down after we turned off the – the nutrients. So, we could have used the variability in space as the early warning indicator for this experiment. It – we didn’t do it with space, but we could’ve if we’d known – we could’ve done it in space.
And –
[the slide animates on a new graph now with Autocorrelation Distance on the y-axis replacing the Standard Deviation and showing the autocorrelation of Lake Peter follows a similar pattern to the Standard Deviation graph]
– I’ve got some other statistics here, but in the interest of time, I’m just gonna skip over them, but –
[Stephen Carpenter, on-camera]
– we can go back to them later if somebody’s fascinated by them, because I think I should wrap up.
So, I’ve – Ive told you a lot today, and I’ve bounced around from –
[slide titled – Conclusions – featuring the following bulleted list – Big changes can occur in lakes, coral reefs, rangelands, forests and other ecosystems; These big changes are rare, but important and hard to predict in advance; Theory predicts that ecosystems become more variable and autocorrelated as stress builds before a big change; Experiments in lakes support theory; Maps could make this easier]
– rangelands to coral reefs to lakes. I could have talked about oceans, but I – but I didn’t, and – and I could have talked about forests, but I didn’t either. So, but the same phenomena are known from them. So, big changes can occur, and they can occur reasonably quickly by human standards, fairly quickly on a human time scale, in resources that we might care a lot about. So, we might need that lake for drinking water, for fishing, for recreation.
Coral reefs are the basis of huge recreational industries in the countries where they occur, and many of those are poor countries where that recreational industry is a key part of the economy. Rangelands are obviously importantly economically for food production, forests for wood and fiber production, and so on. And these things can undergo really big changes.
They’re rare, the big changes are very rare, but when they happen, they’re important, and they’re hard to predict in advance.
I went through a theory that explains that ecosystems become more variable and autocorrelated as stress builds before a big change. And even though the underlying math may seem a little complicated, the rule of thumb is pretty easy. The variability and autocorrelation go up, that means the resilience is getting low, and that means a big change is gonna happen.
And by the way, that theory has been tested in systems other than ecosystems. So, for example, there are studies using it to predict depressive episodes in patients where the patient is pinged with a – a question at random times about five times a day, simple question. They answer the question, and statistics based on the variability of their answers are used to predict whether they are losing their resilience to a depressive episode. And in the papers that I’ve seen, it appears –
[Stephen Carpenter, on-camera]
– to work. I’m not a psychologist, so I – I can’t comment further.
There are also physiological cases with heart attacks and with seizures, with epileptic seizures. So, this seems to be a – a hallmark of complex systems, not merely ecosystems or – or lakes.
I showed you experiments that support the theory, I showed you –
[return to the Conclusions slide with the bulleted list]
– some lab experiments from other people’s work, and I showed you the whole-lake experiments that my group has done. I pointed out that maps might make this a lot easier, and so Emily Stanley’s work mapping the patterns of the pigments so we can calculate the variability from the spatial patterns of the pigments I think is really an important frontier here, and I’m looking forward to working on that more. Grant proposals are in the mill.
[new slide titled – Implications – featuring the following statements – Experiments shown here occurred in near-ideal field conditions; We do not know if they will work in the messy real world of land, lake, and ocean management; Building resilience of good conditions – a safe operating space – can avoid the need for early warnings. Additionally, at the bottom of the slide are the graphs of high resilience (with an ecosystem deep in a valley) and low resilience (with an ecosystem in a shallow valley) are repeated from earlier in the talk]
The experiments that I showed you occurred in near-ideal field conditions, right? We know these lakes very well. We have big teams, ton of equipment. We’re measuring everything, much of it at five-minute intervals, the rest of it almost every day, and we have a reference lake, we have a control to protect us from certain kinds of spurious results.
In the real world, you may not have any of those things. So – so, that’s a caution about the potential application of these methods. We don’t know if they’re gonna work in the real world, because even though we had real lakes, the real world is much messier than our experimental lake situation. For example, we completely control the watersheds, and we control the fishing –
[Stephen Carpenter, on-camera]
– which you don’t in the real world.
So, actually, the smarter thing to do would be to build resilience of things –
[return to the Implications slide above]
– you like, right? Try to make the valleys, that the things you want, as deep as you can. Deepen the valleys for ecosystems that you want. Or for your own health, for – for example. And building resilience means you don’t have to worry about the resilience indicators so much, because even though they work, what they tell you is you’ve lost a lot of resilience, and it would be better to just not lose the resilience in the first place.
So, with that, I will stop. I don’t know if you all take questions, I’d be glad to take them, if that’s your custom.
[applause]
Search University Place Episodes
Related Stories from PBS Wisconsin's Blog

Donate to sign up. Activate and sign in to Passport. It's that easy to help PBS Wisconsin serve your community through media that educates, inspires, and entertains.
Make your membership gift today
Only for new users: Activate Passport using your code or email address
Already a member?
Look up my account
Need some help? Go to FAQ or visit PBS Passport Help
Need help accessing PBS Wisconsin anywhere?
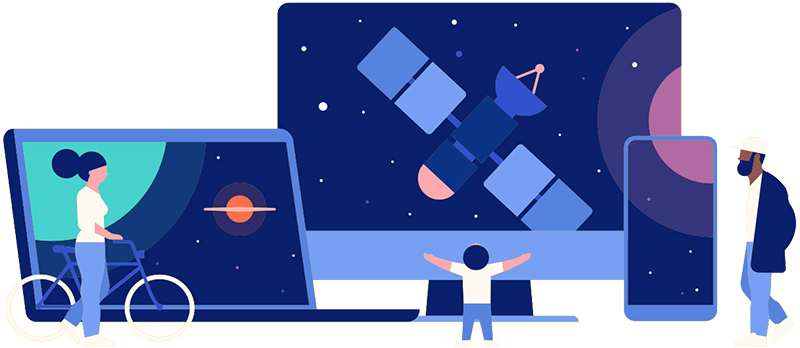
Online Access | Platform & Device Access | Cable or Satellite Access | Over-The-Air Access
Visit Access Guide
Need help accessing PBS Wisconsin anywhere?
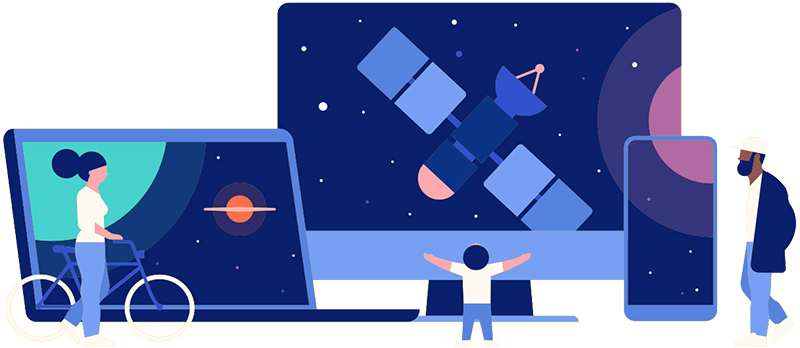
Visit Our
Live TV Access Guide
Online AccessPlatform & Device Access
Cable or Satellite Access
Over-The-Air Access
Visit Access Guide
Follow Us