– Thank you so much everybody, for joining us tonight to this Science and the Public, for the Holtz Center. First, my name is Samer Alatout, and I’m the Director of the Holtz Center for Science and Technology Studies. Now I will introduce– I’m not going to introduce the speakers, but I will introduce the introducer of the speakers. [laughing] My colleague, Cecelia Klingele, and who is a professor in the law school at UW. After receiving her JD from the University of Wisconsin Law School, in 2005, Cecelia Klingele has a great kind of profile, right? Served as a law clerk to Chief Judge Barbara Crabb of the United States District Court, for the Western District of Wisconsin, Judge Susan Black, of the United States Court of Appeals for the Eleventh Circuit, and Associate Justice, and this was impressive, really, to me, although every, all the judges are, you know, with respect to all of them, but Associate Justice John Paul Stevens of the US Supreme Court. She returned to the University of Wisconsin in 2009 as a visiting assistant professor, and has been on the permanent faculty, a permanent faculty member in 2011. Professor Klingele’s academic research focuses on criminal justice administration, with an emphasis on community supervision of those on conditional release. She served as Associate Reporter for the American Law Institute’s Model Panel Code, sentencing revision, sentencing revision, External Co-Director of the University of Minnesota Robina Institute’s Sentencing Law and Policy Program. And past co-chair of the Academic Committee of the American Bar Association’s Criminal Justice Section.
So with that, please welcome Cecelia and the rest of the panel for, for this, and enjoy the talks. [applauding] – Thank you, Samer, and you can only guess with that nice of an intro for me, how fantastic our actual panelists are going to be tonight. It is my pleasure to introduce them, and I will actually do that first, and then make a few opening remarks about the theme of tonight’s presentation. So first, Simon Cole joins us from the University of California at Irvine, and where he’s professor of Criminology Law and Society, and serves as Director of the Newkirk Center for Science and Society. Professor Cole specializes in historical and sociological study of the interaction between science, technology, law, and criminal justice. He is the author of Suspect Identities: A History of Fingerprinting and Criminal Identification, which was awarded the 2003 Rachel Carson Prize for the Society, by the Society for Social Science, Social Studies of Science. He is co-author of Truth Machine: The Contentious History of DNA Fingerprinting, as well, and has spoken widely on the subject of fingerprinting, scientific evidence, and science in the law. He’s also consulted as an expert in the field. He’s written for many general interest publications, including the New York Times, and the Wall Street Journal, and currently focuses on the sociology of forensic science and the development of criminal identification databases and biometric technologies.
His teaching interests focus on forensic science and society, surveillance and society, miscarriages of justice, and the death penalty. Our second panelist is Margaret Hu, who is Associate Professor of Law at Washington and Lee University School of Law. Her research interests include the intersection of immigration policy, national security, cyber surveillance, and civil rights. Previously, she served as senior policy advisor for the White House Initiative on Asian Americans and Pacific Islanders, and also served as special policy counsel in the Office of Special Counsel for Immigration-Related Unfair Employment Practices, Civil Rights Division, in the US Department of Justice. As Special Policy Counsel, she managed a team of attorneys and investigators in the enforcement of anti-discrimination provisions of the Immigration and Nationality Act, and was responsible for federal immigration policy review and for coordination. She is also the author of a forthcoming book, The Big Data Constitution: Constitutional Reform in the Cybersurveillance State. So we have an action-packed evening in front of us. I am honored to kick it off, though I will keep my remarks brief, so that we get more time from our esteemed guests. It was a little ironic, I thought, that I was asked tonight, to speak on the subject of Big Data in criminal justice, and the ways in which it might affect racial disparities.
What I find ironic about it, is that most of the time, when we talk about data in criminal justice, we’re not lamenting that there’s too much of it, but that there’s not enough. For those who work in the field, you already know what I mean, and for those outside of it, let me give you some sense. The collection of data in criminal justice in the United States is complicated by many factors. Among them are the fragmentation of criminal justice agencies. Every police agency, every county clerk of courts office, every district attorney’s office, every iteration of public defenders, and they exist in many different iterations around the country, every correctional agency and jail maintains separate databases in this country. There’s no regularized norm around what data is collected, how it’s reported, whether it’s audited, how it’s reviewed or stored, backed up, maintained, made accessible. Any researcher who has filed freedom of information request acts has often been greeted with the response from criminal justice officials, “Well, I don’t think we can get you that. “We’d have to go through every single file “and pull it out, cause it’s written by hand, somewhere! “And probably sorted in archive if it’s been maintained.” That fragmentation of data, and the lack of adequate controls, usually leads us, again to lament the inability to gather aggregate data about the functioning of our criminal justice agencies, and the way they are either positively or negatively affecting our communities, as measured by any number of different metrics. While we have some ways of gathering some aggregate statistics, mostly through the Department of Justice, and the FBI’s Crime Statistics, there are many flaws to those, as well, and good reason to question the reliability of at least some of that data.
So, again it’s funny that we’re here tonight, to talk about the opposite problem, in many ways. And that’s the problem created by drawing on Big Data, the compilation of large amounts of information, gathered about individuals not necessarily aggregated across police systems. More commonly aggregated by private data collection agencies, and then sold to law enforcement or other interested parties, or generated based on criminal records information that we might have in state court databases. So if criminal justice system actors are so reticent to collect data, and be held accountable for it, what explains the draw, and I would say, the increasing trend to rely on it, when it comes to things like risk prediction algorithms, or hot spot, or predictive policing algorithms? I think that’s best explained by the configuration of the criminal justice system, itself, and the laws that govern it. Again, for those of you who are familiar with the system, you’re aware that although there is of course law, constitutional, statutory, administrative, that governs the behavior of system actors, from police, through prosecutors, through judges and correctional agents. In fact, many of the day-to-day decisions made by those actors are not dictated by any statute, but are rather governed by a principle we call discretion. That is the legally-authorized authority of system actors to select between multiple, equally-permissible legal options. For the beat officer, that means that when you see the child spray painting the wall, you can either tell him to go home, or you can give him a ticket. Or you could arrest him, you could refer him for charges, or not.
You could take him to his parents, right? Any number of choices, all of them equally legally permissible. The same is true, not only for arrest decisions, but for the decisions about whom to surveil. About what charges to leverage against an individual defendant. About whether to set bail, and if so, what the amount to be, or the conditions of release. What sentence to impose for an individual who’s been convicted of a crime, and what kind of supervision or custody to give to those who are already being punished for a criminal sentence. Those are hard decisions. They don’t have clear, right or wrong answers. They require the balancing of many difficult and complicated moral, sociological, and other factors, outside of the law, that often leaves police officers and sentencing judges awake at night, wondering if they’ve done the right thing. The idea that we could outsource those really hard decisions to a math problem is super appealing.
Math sounds so objective, and fair, and clear-cut, and the desire to assuage our guilty consciences, or at least our anxiety about getting the right answer in a difficult and complex situation, is such that I think data feels concrete, and safe, and reassuring to many in the criminal justice system. Now I’m oversimplifying. There are also those who are inherently skeptical. Particularly in criminal justice, and particularly when we talk about the law, and the legal process. Because, as all of us know, if lawyers and judges were good at math, we’d be doctors. [chuckling] That’s for you Jerry. [audience chuckling] Of course, there are some in law with mathematical talents that far exceed my own, but the reality is there is something about feeling safe that math is certain, and not quite understanding the magic mix that goes into the data-crunching behind many of these numbers that makes it particularly appealing in a complex and difficult enterprise like the administration of criminal justice. But there are, of course, dangers of over-relying on data. And I do mean over-relying, because, certainly, there are many positive things to be found in numbers, that check our intuitive gut sense of what’s happening on the ground, or who is being affected in what ways by the decisions that we make.
Data plays an important role and in many ways, we need to get better about collecting it. But the ways we use it matter, and there are several dangers that I will throw out, and then turn the stage over to those who can unpack it for us much better than I can. The first is a failure to often recognize that the data themselves are often flawed. When we rely on information in systems, whether that information is used as it is first presented, or whether it’s first put through a complex equation to generate a new number or prediction for us, the quality of that data matters. And the reality is that turning something into a number doesn’t change inequities in the collection of that data. Or problems in the quality that exists. Take for example, predictions about criminal recidivism. Those are ones with which I’m particularly familiar, because they often affect sentencing decisions and correctional supervision. In those cases, we strive, with algorithms, to generate aggregate predictions of about what individuals with similar backgrounds to a particular defendant we believe are likely to do in the future when it comes to re-offense.
But, there are a lot of problems with that. First of all, and most simplistically, those data don’t tell us anything about actual human behavior. All they tell us about is human behavior that’s gone awry, and been detected by law enforcement. The reality is that the universe of criminal behavior is much, much, much, much broader, than that of detected criminal behavior. And as a result, depending on where you live, and how old you are, and whether you’re a guy or a girl, you are more, or less likely, to be detected committing crimes. And if we’re relying on information about crime detection, that’ll tell us about the likelihood perhaps, of someone like you being detected again in the future, but it doesn’t tell us anything about the actual behavior of you or the general population. Or very little. Second, I think there’s a danger of misunderstanding the limitations of the data themselves. Again, in the risk prediction context, often, risk of future offense in the sentencing arena, is predicted out as whether a person has a low, a medium, or a high risk of recidivism.
And usually when I poll lawyers and judges, they tell me they can’t tell me what the exact percentage is, that means low, medium, or high, but they’re sure there is one. Not true. In fact, most of these data are not absolute. They’re comparative. They’re comparing populations against each other for the frequency of predicted re-offense. In other words, if you live in a really, really dangerous place, it may be [laughs] low-risk people are actually higher risk than they might be in a different population. In so far then, as what we are trying to do in the criminal justice system, is not only, protect people from future risk of harm, but maybe more importantly, hold people accountable for actual decisions that they have made in the past to harm others. These data may not tell us all that we think is important about either moral culpability, propensity to offend, or to change, character, the possibility of future growth. And if those are the things that matter to us at sentencing, then, bad news, guys.
We can’t outsource it. And so I hope today’s conversation will help us, first of all, better understand all those numbers, cause I don’t understand them, and maybe some of you are with me on that. But also better understand what the limitations and the possibilities of this information, ways in which it may be having an inequitable effect on some members of our community, and ways that hopefully, we can use it to make our system better, and more fair. So with that I’m happy to turn it over to Simon and Margaret. [applauding] – Thank you so much for the wonderful privilege to join you this evening, for this very important conversation, and very grateful to Lynn and Samer at the Holtz Center, and to Cecelia for that very generous introduction, and for contextualizing these issues in such a brilliant way, thank you so much. So what I wanted to do today is focus on Big Data in discrimination. And, really, feel very fortunate to be here because I just published an article with the Wisconsin Law Review called Crimmigration-Counterterrorism, where I talk about the conflation of crime, immigration, and counter-terrorism, or national security rationales, through programs such as the Muslim Ban, and extreme vetting. I also have another article that I just published on the Muslim Ban and extreme vetting called Algorithmic Jim Crow, that was published in Fordham Law Review. So I wanted to start today, by talking about extreme vetting as a way to help us wrap our minds around modern governance that involves Big Data, and the rationales that support it.
Then I wanna go into a little bit more detail about mass surveillance, justifications, Big Data intelligence gathering methods, Small Data surveillance that we used to have in the Small Data world, versus the Big Data, cyber-surveillance schools that we now have at our disposal. And then, if there’s time, get to the Snowden Disclosures. So, extreme vetting, what is it? Extreme vetting is a way to understand the modern landscape that we have, with the ubiquity of social media, and online information. So back in December 2015, then-presidential candidate Donald Trump published a Statement on Preventing Muslim Immigration on his campaign website. The statement called for a total and complete shutdown of Muslims entering the United States until our country’s representatives can figure out what is going on. Then, shortly before the election, he announced a proposal for what he called extreme vetting of immigrants and refugees. He later explained, the Muslim ban is something that in some form has morphed into an extreme vetting from certain areas in the world. So the Muslim ban or what is referred to as the Travel Ban, and extreme vetting, should be understood as one of the same, so what is it? So you have under the former administration, the former Director of the United States Citizenship and Immigration Services Office of the Department of Homeland Security, explained that they already started some form of extreme vetting during the Obama administration, that prospective refugees from Syria and Iraq, since 2015, had their Facebook, Twitter, and Instagram accounts checked, and then, the then Department of Homeland Security Secretary, now Chief of Staff, John Kelly, explained, in Congressional testimony, that extreme vetting would be an accounting of what websites that those refugee applicants would be visiting, what telephone contact information they had in their phones, to see who they were talking to, social media information, including passwords. So, further information about extreme vetting was revealed in a media report that the Department of Homeland Security held what they called an Industry Day in 2017, in Arlington, Virginia.
It circulated a document, at that time, called the Extreme Vetting Initiatives, and a host of industry representatives were there. The document stated that, right now, it is difficult for the government to assess threat because the data is collected, that is fragmented, and it’s very time-consuming and labor-intensive to make sense of it. And so they were inviting solicitations of proposals to help do this type of vetting tool that would automate, centralize, and streamline vetting procedures, while simultaneously making determinations of who would be considered a security risk. The system was purportedly supposed to then, predict the probability of an individual becoming a positive member of society, as well as whether or not they had criminalistic or terroristic tendencies. The attendees included for example, IBM, Booz Allen Hamilton, LexisNexis, and other companies, and in the solicitation, it stated that the contractor shall analyze and apply techniques to exploit publicly available information such as media, blogs, public hearings, conferences, academic websites, social media websites such as Twitter, Facebook, LinkedIn, radio, television, press, geospatial sources, Internet sites, specialized publications with intent to extract pertinent information regarding targets, including criminals, refugees, non-immigrant violators, and targeted national security threats and their location. So, basically, what they said was all publicly available information of all persons to be turned into some type of automated tool, some type of algorithm, to assess risk and predict terrorism, so during a follow-up Q and A session, one of the attendees asked whether or not this was basically legal. And it was an anonymous question, and the Department of Homeland Security responded that there’s a prediction that in the future Congressional legislation might address this, but, basically, they’ll continue to do it until they’re told that it’s not legal. And that’s basically where we are. In the legal regime, in our regulatory structure for this type of online collection, or using social media, or using sort of internet digital footprints that we leave behind, that’s publicly available, all of it’s considered fair game.
So, this leads us to what is Big Data cyber-surveillance, and what are some of the justifications for it? So in a Big Data cyber-surveillance world that we find ourselves in, right now, the analytical method is you start with the data. And what that means, for the government, is that the data becomes suspicious. It’s not necessarily that people are suspicious anymore. And this also has led to pre-crime ambitions. So just as the Department of Homeland Security invited these corporations to come up with tools to assess risk and predict terrorism and crime before it occurs, that has led to basically, a minority report type of world. And the way that this was explained by the CIA’s Chief Technology Officer was that, since you cannot connect dots that you don’t have, it drives us into mode of fundamentally trying to collect everything, and hang onto it forever. Forever being in quotes, of course. It is nearly within our grasp, he explained, to compute on all human-generated information. So, he said, “It’s all about the data, stupid.” Revolutionize Big Data exploitation, acquire, federate, secure, and exploit.
Grow the haystack, and magnify the needle, so oftentimes you hear in the intelligence community, that you need to have the biggest haystack possible in order to find the needle. But what some experts have explained is this is a put the haystack before the needle approach to governance and intelligence gathering. And what this does is it pre-supposes that there is a needle in the haystack before you even know that there’s a needle. And so, in a Small Data world, you started with the needle. You started with a person who was a criminal, a suspect, a crime, and then you went vertical into the information gathering. Because, the resource limitations and the technological limitations required a vertical, downward drilling of data to support that original question. But in the Big Data world, you actually flip everything around, and you start with the data. You answer the question after the fact. You look at the data, and then you say, “From this data, can I find a suspect? “Can I find a crime? Can I find a terroristic risk?” And so everything has been flipped upside down, and this has led to basically virtual suspects or we can have a digital avatar of ourselves that becomes the representation of the targeting of the government action.
So Edward Snowden, when he came forward with the Snowden Disclosure said, “Does this method work, or are we just drowning in hay?” Are we building more and more hay, and do we have larger and larger haystacks, that doesn’t necessarily lead us to any conclusive resolution. And also another risk of this, as explained to another intelligence source, is that everyone now is a target. So even though extreme vetting, for example, is presented on just trying to find a target who is potentially a suspect, a criminal, or a terrorist from these refugee vetting procedures, you saw from that extreme vetting day that you Collect it All. It is a Collect it All method, it doesn’t matter what your citizenship is, it doesn’t matter your immigration status. The method requires collecting it all, and everyone who has any digital communications becomes a target. And what this also means, is that our digital devices are the ancillary representatives of us, ourselves, and so, Snowden explained that part of the key inquiry of the intelligence community is whether the phone is suspicious. Not necessarily whether or not the person is suspicious. So, we now have for example in the Obama administration, it was revealed that we have signature strikes, where the identity of the individual of the drone strike is not known. And you had one drone strike operator explain that please people get hung up that there’s a targeted list of people on the kill list.
It’s really like we’re targeting a cell phone. We’re not going after people, we’re going after the phones in the hopes that the person on the other end of the missile is the bad guy. So not knowing the identity, but because you have suspicious data being generated by a suspicious phone, you’re killing the phone, you’re killing the person who’s holding the phone. And you also had this, shortly after the Snowden Disclosures, the former Director of the CIA and the NSA, General Mike Hayden, saying we kill people based on metadata. Metadata is data about data. Time of a call, place of a call, length of a call. In a Small Data world, it would be hard to imagine killing people based on that type of data, but in a Big Data world, everything has switched around, and now, you have the former Director of the CIA and the NSA, saying that metadata can form the basis for actual, lethal consequence. This also has led to pre-crime ambitions. So, for example, the Department of Homeland Security has a test pilot program that they call the Future Attributes Screening Technology Program.
In the news, and the media, it’s referred to as a pre-crime program. Under this test pilot program, you have the Department of Homeland Security saying that they’re going to collect physiological cues such as body and eye movements, eye blink rate, pupil dilation, body heat changes, breathing patterns, as well as linguistic cues, such as voice pitch changes, alterations of the rhythm, and changes in the intonations of speech, to detect malintent. Or, in order to detect this threat risk assessment. And the volunteers of this program were informed that the consequences could range from none to being temporarily detained, deportation, prison, and death. And this is based on things like linguistic cues and physiological cues. And so, something else that we have seen from the Snowden Disclosures is that these types of fragments of data are leading to more and more aggregated information that allows for the government to believe that they are engaging in the most efficacious types of decision making that they think is now possible through these technological advances. And, you have some scholars, such as Jack Balkin at Yale Law School, calling this the National Surveillance State. So he says, “It’s one of the most important developments “in American Constitutionalism, “that is the gradual transformation “of the United States into this National Surveillance State “that is the logical successor of basically “our administrative state and our welfare state.” He said that it’s just going to become a way of governance, and we’re going to integrate surveillance into the way that we just carry out our day-to-day business. You have other experts such as Benjamin Wittes, saying that the problem of the justification of mass surveillance is that, the problem is not so much a rule of law problem, it’s really a technological problem.
That, in the United States we might have one of the most constrained intelligence communities in the world but that the US intelligence ambitions scale up to our geopolitical ambitions. And that we have the most awesomely powerful supercomputing capacities of anyone, on earth, so part of the question now is how is it possible that we have, potentially, the most constrained legal apparatus imposed on us for intelligence gathering, and yet, still have potentially the most pervasive and invasive system. So, I wanted to move to now some of the legal apparatus and justifications that help support this. So for example we have the National Security Presidential Directive 59 Homeland Security Presidential Directive 24. It was signed by President Bush in 2008, and it’s called The Biometrics for Identification and Screening to Enhance National Security. So it directs the military and the federal government to collect, store, use, analyze, and share biometrics, and contextual information. It doesn’t define contextual information, but Microsoft defined it this way. All locations that you go, all the purchases you ever make, all your relationships, all activity, all your health, governmental, employer, academic and financial records, your web search history, your calendars and appointments, all your phone calls, data, texts, email, all peoples connected to your social circle, all your personal interests, and all other personal data. So this helps to explain, for example, this slide from the Snowden Disclosures.
So here you had Sniff it All, Know it All, Collect it All, Process it All, Exploit it All, Partner it All. That basically summarizes the philosophy, or the ethos, of the new Big Data cyber-surveillance systems that we find ourselves in. Here’s a slide from one program, Social Radar, from the US Air Force, and you see that it is Collect it All. It is any possible potential communication, any type of information, that can be gathered, you see military, religious, political, economic health, geography, demography, econometrics, using public sources, polls, and surveillance, and then, using that to see into the future. You also have this slide. Total Information Awareness, which was technically defunded after 9/11, but this is also another Collect it All program. So you see that starts with biometric data, and then it goes to automated virtual data repositories, intelligence data, transactional data, including financial, education, travel, medical, veterinary, country entry, place, event, transportation, housing, resources, government communications. So what I wanted to point out was that here is this Hollerith machine. This was found in the Holocaust Museum.
And you had Edwin Black, a journalist, go into the Holocaust Museum in Washington, DC, and asking the archivist, “What is this IBM machine “doing here in the Holocaust Museum?” And after a multi-year study, he produced his book, The IBM and the Holocaust, and what he found was that through the punch card system, the Third Reich was able to very efficiently, through this data collection, and processing system, execute the Final Solution. And, I wanted to put two posters side-by-side. The one on this side, is the Third Reich’s Hollerith machine poster, and then the other one is the United States poster for the Total Information Awareness Program. And there’s some uncomfortable parallels between the two posters. So I wanna now talk about Big Data intelligence, and datafication. And this brings us to a little bit more information just on Big Data and how does it work? So part of what’s happening is we’re going through a transformational moment in our history, where, as in 2000, 25% of all stored information was digitized. By 2012, 98% of all information was digitized. So as far as the scalability of it for example, imagine a gigabyte as a full-length feature film in digital form, and a petabyte is one million gigabytes, an exabyte is one billion gigabytes, and a zettabyte is one trillion gigabytes. And that’s the type of stored data world that we find ourselves in now.
So, the prediction is that the data, the digital data that we create is expected to double every two years, through 2020. And by the year 2020, we’re going to have 5,200 gigabytes of data for every man, woman, and child on earth. And, what some experts such as Mayer-Schonenberger and Cukier in their book, Big Data: A Revolution That Will Transform How We Live, Work, and Think, explained, is that this converts all social and physical reality into a digital format, and that the transformation of that digital data is converting data into new forms of value. And so, I just wanted to give a few statistics on datafication. So for example Google’s more than 100 petabytes in size, experiences more than 7.2 billion page views per day. Processes more than 24 petabytes of data per day, a volume that’s thousands times more the quantity of all printed material in the US Library of Congress. By 2012, Facebook had already reached one billion users, 35% of all the digital photos are stored on Facebook, with 10 million new photos uploaded per hour. YouTube is more than 1000 petabytes in size, over 72 hours of video uploaded to YouTube every minute, more than four billion views per day. 800 million users upload over an hour of video every second.
Twitter, more than 124 billion tweets per year, grows at 200% per year, and at 4500 tweets per second. So we’re also seeing a datafication of us human beings, through the government. The datafication through biometrics, for example, 70 million fingerprints in the criminal master file at the FBI, 34 million civil prints, over 10 million DNA files, and approximately 70 million digital photos for the facial recognition technology for the FBI. The Department of Homeland Security is similarly moving towards datafication through biometrics. Approximately 300,000 fingerprint scans collected every day, 130 million fingerprint files on record, and the Department of Homeland Security has started to gather the DNA of refugees, at at least two refugee sites. You have the Department of State also gathering digital photos that can serve facial recognition technology, such as 200 million digital photos through the passports, and 75 million digital photos through visas. So bringing this back to the Snowden Disclosures, we can see that biometrics and facial recognition technology is forming the basis of new forms of intelligence and the way in which the government is using biometric identity in order to anchor decision making through a formulation of targeting, that is the combination of biometric and biographic information. So, through one of the Snowden Disclosures it was revealed that the NSA intercepts millions of images per day off of the Internet, including 55 thousand facial recognition-quality images. And it was explained that, it’s not just the traditional communications we’re after, but it is the compilation of biographic and biometric information to implement precision targeting.
So it’s basically the fusion, 24/7, of biometric body tracking, with the 360-degree biographical tracking of us as individuals, through, for example, our digital footprints, and our online personas, in order to facilitate data-driven decision-making. So, I want to just wrap up by explaining… Okay, Big Data is technically defined. So you do have these definitions such as volume, velocity, variety, veracity, value, variability, I don’t know why they focused on the Vs, but they did, when they defined Big Data. But Big Data is much more than that. Big Data really is better understood as a philosophy of governance. Big Data as a philosophy is a theory of knowledge, it’s a theory of decision-making, it transforms knowledge, and it transforms in the eyes of government, what it can do, and what it should do. And this affects all of us, so going back to extreme vetting, extreme vetting is misunderstood as a national security program. Extreme vetting is the new normal of the world that we find ourselves in today.
Extreme vetting, and the forms of Big Data Collection and Big Data cyber-surveillance is going to, eventually, influence every right, privilege, freedom, and liberty that we have. So we’re already at the earliest stages of it, in the way that you have, for example, voting rights, having database screening through the Department of Homeland Security databases before there’s an assessment of whether or not you are the appropriate individual of a certain identity that can vote. So there’s digital mediation of voting, you have digital mediation of your right to work, so, employers are screening employee’s data through the Department of Homeland Security databases. You have determinations of who can enter the border, so freedom of movement, but this is just the earliest stages. Right now, through things like the No Fly List, the No Work List, the No Vote List, these are the earliest stages of the Big Data type of capacities that the government has to mediate our rights and privileges. And so, we cannot continue to see the world through these Small Data eyes. The world that we understand it, as it is, right now, we see through the lens of Small Data because that’s what’s humanly knowable. We are about to transform into a world where the government and those that formulate policies for us, look at the world through Big Data glasses. So what they can understand algorithmically, who they can classify as a risk, and what decisions they can make based on those threat-risk determinations.
And so that’s the future of discrimination. So unlike the world that we once had, for example, under Jim Crow, so this is my thesis in algorithmic Jim Crow, when you had race, you had the classification based on something like skin color, for example, and then you had the delegated screening that was conducted by humans, bus drivers, people who owned theaters, people who owned restaurants. They were supposed to do the screening, they were supposed to isolate individuals and then reject them based on their skin color. In a modern world of Big Data, discrimination is going to be operated technologically. So the classification won’t necessarily be based on race, national origin, religion, though it might correlate with it, it’s going to be based on, for example, statistical data assessing risk, and then you’re gonna have classifications that will not necessarily be human judgment, but will be through an algorithm. The algorithm is going to do the screening, and then that is how you’re going to have deprivations of privileges and freedoms through some type of technological method. And we simply do not yet have the legal tools, or constitutional tools, to mediate that type of new form of discrimination that we’re now starting to witness at the very earliest stages. So why don’t I conclude, here, and thank you so much for this opportunity to speak with you today. [applauding] – We’re talking about Big Data, race, and the criminal justice system.
And I wanna begin just by briefly mentioning that these issues have kind of been endemic in biometric identification since the very beginning. Issues of race, issues of colonialism. You’ll find that biometric identification was first sort of pioneered in the laboratory of the colony, and only then, brought back to the metropole, which is something that Professor Hu in her other work talks about, is still going on, now. And the issue of predicting individual behavior. So, I’ll begin by saying a little bit about race. The first ways of identifying people were through looking at their kind of gross, physical aspects through photographs, or through the anthropometric system, where they took measurements, did meticulous facial descriptions, and looked at peculiar marks and scars on their bodies. And this, this system looked, in great detail at the kind of continuum of human variety. The anthropometric system had more than 20 different shades of brown eyes that it identified people with. But this system was considered by Europeans to be unworkable in the colonies, and here’s a quote, quotation, from Francis Galton, saying, well, we can’t use this system in India, and they said the same about China, because they all look the same to us, so we can’t, and, and so, it’s for that reason that fingerprinting arises in India and not back home, in Europe.
It’s the same in the United States, where fingerprinting was considered extremely appealing for identifying Chinese immigrants in the late 19th century. But it was considered not useful for identifying Europeans, and here’s this great quotation from the San Francisco Police Chief, saying, well, you can use fingerprint, you can use fingerprints for indifferent Hindus and wandering Arabs, but when you’re identifying white people, we need something else. We need to look at their faces. And we had the same thing in Argentina, the other cradle of biometric identification, where race played out in kind of a different way between northern and southern Europeans. We have, in the United States, in 1903, the famous Will West case in which, which supposedly demonstrated that fingerprinting could distinguish between two supposedly indistinguishable African American men, who were then distinguished by their fingerprints. This didn’t actually happen, but it, the important thing is it became the sort of origin myth of why we use fingerprints. Then we fast forward a couple decades to 1925, and we have, in the New York City Police Department, the head of the identification bureau saying, who’s talking about his fingerprint file. And here he’s got supposedly this extremely individualizing biometric technology that can identify people down to the individual level, and distinguish between identical twins, and so on, and he’s saying, “Yes, we have that, “but we also sort our files into three groups. “Black, white, and yellow.
“And we do that just by looking at them, cause we know “those races when we see them.” So we have sort of the coexistence of this individualizing technology and this very crude division of people into three groups. And I’d argue that we’re gonna see this kind of throughout this history. This kind of progression, this effort towards individualization that ends up with grouping. And the whole push to use biometrics in criminal identification was about being able to treat criminals as individuals. The idea was that if people were, if we didn’t, before we had biometric identification, people would use aliases, and then we wouldn’t know how long their criminal records were, and then we wouldn’t actually be able to measure recidivism. Once we had biometric identification, then the people who ran prisons, and police departments, could think that we have a pretty good way of detecting recidivism. And then we can do what we’ve always wanted to do, which is punish first-time offenders and recidivists differently. Now this was sort of promoted as individualized punishment, that we would tailor the punishment to the individual. What actually happened was grouping.
There were two sets of prisons. One for the first-timers, and one for the recidivists. So the whole population was divided into two groups, but you did at least segregate off the first-timers from the recidivists, who supposedly would contaminate them with their criminal ways and you could maybe keep them away from that. It’s important to note, for what we’re going to get to later in our discussion, that even though biometrics was useful for detecting some recidivism, it prevented people from using an alias every single time they were arrested. It didn’t of course it wasn’t, of course, a perfect tool for detecting recidivism, for precisely the reasons mentioned by Professor Klingele, that we don’t, that law enforcement doesn’t detect all criminal behavior. In addition, there are other issues with defining recidivism. What do we mean by recidivism? Do we mean committing the same crime, or do we mean a different crime? Do we mean if you are crime-free for 20 years and then you commit another crime, are you a recidivist? Do parole violations count as recidivism? And does that have something to do with how stringent your parole conditions are? And so on. Fast forward a couple more decades, and people start talking about this fingerprint system is great, and shouldn’t everybody be in a database? Shouldn’t we put the whole population in the database, and this discussion, in this country, took place in many countries, and this country sort of peaked in the late 20s, and early 30s. And Americans rejected it, between 1935 and ’43, efforts to create a full citizen fingerprint database were defeated.
And this in a sense, created what I call the arrestee compromise. It created two groups of people. Criminals, which meant, not just people convicted, but anyone who’d been arrested. ‘Cause if you get arrested, your fingerprints go in the database. Plus, some other people. Civil servants, military people, people who work with children. And then, the rest of us who get the privilege of not being in the database. And so this arrestee compromise is going to come back today, if we fast forward a few more decades, we get another biometric technology, seemingly more powerful in many ways, called DNA. And that brings about a kind of public policy problem.
How big should the DNA database be, and who should be in it? So it’s important to realize, that the smaller your DNA database gets, the less useful it is. And if your DNA database is anything short of everybody, there are gonna be some rapes that are going to go undetected. And, those people are going to rape other people. So there are certainly costs to reducing the size of the database, but those have to be balanced against what we, as a society, think is, is fair. And in this country, and in most other countries, the DNA database public policy problem kind of ended up in the same place the fingerprint one ended up with it, which is on the arrestee compromise. In 30 states, including Wisconsin, and my state of California, the US and the UK, we have arrestee databases. And so it is, it is sort of the same compromise that we struck with, with fingerprints. Now the problem with arrestee databases, of course, is that arrest practices in policing is not race-neutral, and it’s not class-neutral, and it’s not geography-neutral. So the fact of having an arrest seems to have something to do with your race, and it can become a sort of backdoor to race, or as Professor Hu has written, race at the backend of the process.
And in this little, sort of quick and dirty kind of attempt to estimate the racial composition of arrestee databases, population-wide databases, and convict databases, I found that the arrestee database is the one that gives you the smallest number of white people and the largest number of black people, as sort of not surprisingly. And this is kind of exacerbated by the fact that familial searching can now be done, which means that if somebody is in the database, their close blood relatives are effectively in the database, even though they’re not in the database. And so, the racial implications sort of balloon out from there. So the arrestee compromise is in some sense the least fair possible system we could come up with, which is what we’ve decided as a society to do. There are two fairer solutions. One is to have a universal database, and put all of us in the database, and then we can all bear equally the burdens of the privacy violations, and the risk of being wrongly convicted, and the burdens of familial searching, and so on, and a number of people have advocated this, who are list, listed here. Kind of on the principle of anti-discrimination, including Alec Jeffreys, who developed DNA typing, and the law professor Michael Smith, who teaches here, I believe. So go see him, he wants all of your DNA to be in a database. But for good reason.
As an anti-discrimination measure. Or, you could maybe have a convict database, which is possibly justifiable on the grounds that you should have reduced privacy because you’ve been convicted of a crime, not just merely arrested for one. I wanna move now to a slight, slightly different topic that is, I’m gonna try to bring together with DNA in a minute. And Professor Hu writes about this as well, which is the development of a kind of philosophy called forensic intelligence, which we could, perhaps distinguish between from treating forensic information as evidence. So forensic intelligence is kind of a new, a hypothetical, mostly, new approach, towards forensics, that distinguishes from the old approach in the following ways. Whereas evidence was oriented towards law and the trial, forensic intelligence is oriented toward policing, and security. Forensic evidence is reactive, it comes in after a crime has occurred. It tries to solve the crime, and it tries to prove who did it. Forensic intelligence isn’t interested in that.
It’s interested in preventing crimes, and being proactive in linking crimes together. Forensic intelligence is very appealing in a lot of ways. There’s a lot of problems with bias, and unscientific reasoning in forensic science that I talk about on other occasions. And forensic intelligence has a lot in common with people like me who are trying to reduce those issues in forensic science. So, it will be less biased. It uses more, kind of probabilistic and scientific reasoning. So it has a certain appeal. But, let me get to the unappealing aspects of it. So the sociologist Sarah Brayne has written, recently, in her study of Big Data policing, how this kind of approach widens the criminal justice dragnet, but in unequally distributed ways, while appearing to be objective, as Professor Klingele mentioned, having the appearance of being simply math.
That this bias can kind of self-perpetuate the existing biases in the data that is fed into these algorithms. And again the simplest example of this is having an arrest, or not having an arrest. Your chances of acquiring an arrest, if you’re a drug user, are not the same depending on your race, your class, and your geographic location. And so, we have criminal justice algorithms, and we have the Loomis case, here, in Wisconsin, and complaints about the use of criminal justice algorithms to predict human behavior, and as Professor Klingele mentioned these algorithms are in some sense, appealing. And the main reason is concerns about bias in the non-algorithmic ways about in the use of discretion by human beings, which is disturbing. And, as Professor Hu has written, the criminal justice algorithms sort of appear, on their face, to be, actually, equality enhancing. Now, two main critiques of these algorithms are lack of discretion, that same discretion that we’re sort of getting rid of, and lack of transparency. So I’m gonna get to those two in second, but now I’m just gonna add a third kind of tech modality to forensic intelligence and DNA databases, and that’s probabilistic genotyping. So I don’t have too much time to go into probabilistic genotyping.
If you wanna know more about it, you can talk to Professor Keith Finley in the law school, and he’ll maybe tell you a little more. But, forensic scientists are pretty good at handling very clean, single-source DNA samples. But forensic DNA profiling starts to get very complicated when you have mixed samples with multiple contributors to them and small amounts of DNA, and they become very difficult to interpret and there have been problems with biased interpretation of these samples. And so, along comes probabilistic genotyping, which says, let’s have a computer interpret these things, according to a set of rules that we’ve pre-programmed before we got the case, so we’re not gonna be biased towards some particular outcome in this case, and plus, the statistics for these complex mixtures is very difficult anyway, you need a computer to do them anyway. So that’s the kind of appeal of them. On, at first glance, and again, that’s a legitimate appeal. You have a couple of problems, though, so in the bottom left you have the legal battles over the source code. ‘Cause defendants say, “Fine, but I’d like “to see the source code that led to this interpretation,” and the makers of the software say, “That’s the entire intellectual property “of our company, and so you can’t see it.” And the courts have to resolve this, which they usually do in favor of the company. On the upper right, you have the Oral Hillary case, which is a murder case in upstate New York, allegedly committed by a black man who was dating a white woman, in a very white part of the country.
And, with a very small DNA sample that required very complex interpretation, and the two, two different pieces of probabilistic software reached two different results in this case. This got very complicated, and then, in the bottom right, I have a press release from the company, Cybergenetics, one of the main vendors of this probabilistic genotyping software saying, that NIST, the National Institute of Standards and Technology, which announced a study where they would test these algorithms, and measure how accurate they were on known samples, Cybergenetics released this news release saying this was a waste of taxpayer money and anti-science, and that they are sort of trying to create a, manufacturing, for over a decade, NIST manufactured crises in DNA mixture interpretation to amass money and power. So, the two critiques, lack of discretion, and lack of transparency. Here’s an op-ed from the New York Times that focuses on the lack of discretion, and her antidote to these criminal justice algorithms is go back to discretion. Have a good old human judge work these things out. Well the problem with that was some, there were some problems with discretion, and the good old-fashioned human, human judge. So it’s not clear how appealing that is. And here’s the lack of transparency argument. This was published by a computer scientist, Duke, who objects to the proprietary nature of the compass algorithm that was used in the Loomis case, and says, “I have a non-proprietary, transparent, “open algorithm, which is better.” And that is true.
Transparency is better than secrecy, but notice that she still has an algorithm that she says predicts criminal behavior really well. And so I wanna emphasize is what we’re doing is the same old thing. We’re predicting criminal behavior, which we’re measuring by arrest, or contacts with the police, and we’re predicting it from arrest, and contacts with the police. And we are bracketing the problem, the detection problem, of whether we’re actually detecting criminal behavior or we’re just detecting that criminal behavior which led to contact with the police. And so we’re protecting, we’re bracketing the problem, the problems with the data that are both input and output from this algorithm, even if it is transparent, which is better. And so, in conclusion, I want to suggest that with criminal justice algorithms, we’re sort of ending up in the same place, in something kind of like the arrestee compromise, where we use these algorithms to kind of predict dangerousness about people kind of like arrestees, right? They’re people with enough contacts with the state that they start to look dangerous in these predictive algorithms. And so, and that seems like it’s going to be kind of discriminatory through the back door or at the back end. And it kind of brings us back to if we don’t like this, what are our other choices? And again with the DNA database, we had two choices. We had every, we had everybody [laughs] or, just convicts.
Now what would that look like in terms of criminal justice algorithms? Well the everybody would be everybody. But as Professor Hu just told us, it wouldn’t just be our DNA samples anymore, it would be everything about us. So it would be everything about everybody. So that would be fair. We’d all bear the privacy violations equally. We’d all bear them together, but we end up with sort of a total surveillance society, or as Professor Hu said, a National Surveillance State. Our other choice is to sort of go back to something like convicts, and to focus, and to focus on people who we can prove have done crimes. And that we’re reasonably sure did them, and not on people who we predict will do them. And kind of the most, the most extreme advancement of this view, is Bernard Harcourt, who’s written a book called Against Prediction, which, as the title indicates, for kind of the reasons I’ve explained, is against prediction altogether, as an activity, not making it better, doesn’t, believes we shouldn’t do it at all.
So thank you. [applauding]
Search University Place Episodes
Related Stories from PBS Wisconsin's Blog

Donate to sign up. Activate and sign in to Passport. It's that easy to help PBS Wisconsin serve your community through media that educates, inspires, and entertains.
Make your membership gift today
Only for new users: Activate Passport using your code or email address
Already a member?
Look up my account
Need some help? Go to FAQ or visit PBS Passport Help
Need help accessing PBS Wisconsin anywhere?
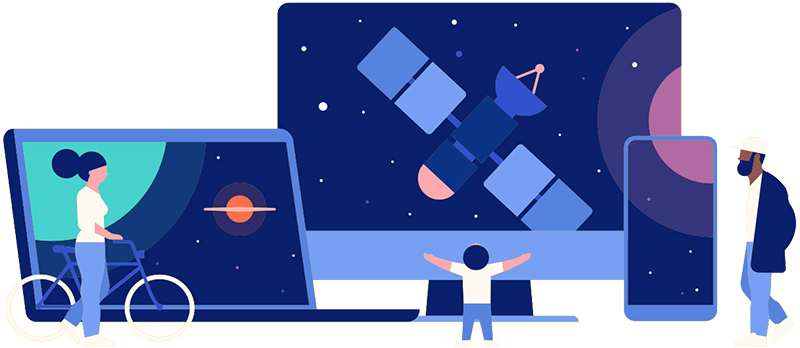
Online Access | Platform & Device Access | Cable or Satellite Access | Over-The-Air Access
Visit Access Guide
Need help accessing PBS Wisconsin anywhere?
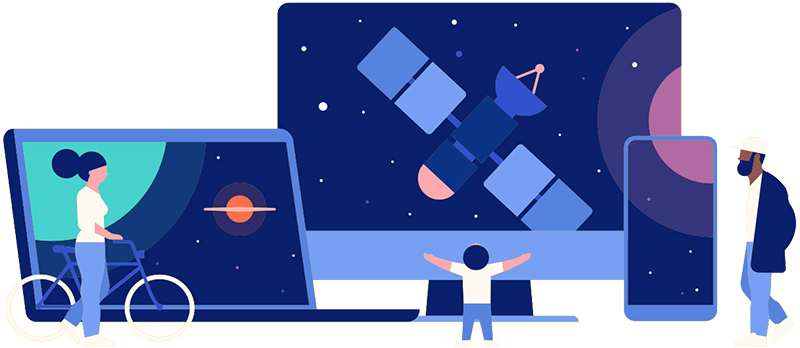
Visit Our
Live TV Access Guide
Online AccessPlatform & Device Access
Cable or Satellite Access
Over-The-Air Access
Visit Access Guide
Follow Us