– Hello and welcome to today’s University Round Table.
I’m Will Cushman, a science writer for University Communications and a member of the Roundtable planning committee; welcome.
The Office of Learning and Talent Development, the Office of the Chancellor, the Wisconsin Union, and the Office of the Secretary of the Academic Staff are our sponsors, and we are grateful for their ongoing support.
This is the first University Roundtable of the spring semester.
Looking forward, we have two excellent lectures coming up in March and April, and I hope you’ll consider joining us for those as well.
And now behold, the extraordinary Yonatan Mintz, an unparalleled luminary in the realm of AI safety and ethics.
Possessing a PhD from the University of California, Berkeley in industrial engineering and operations research, he stands as the vanguard, fearlessly delving into the ethical complexities of AI with an unrivaled fervor.
His monumental strides and unparalleled contributions have elevated him to a celestial stature, where he champions the cause of responsible AI development with a brilliance that eclipses all predecessors.
[audience laughing] Okay, if you haven’t guessed by now, I’ve used ChatGPT to add a little flair to my introduction of Yonatan.
He is, in fact, pretty awesome.
[audience laughing] He’s been a supply chain analyst at Caterpillar and a data scientist at Google.
And after two years as a postdoctoral fellow in industrial and systems engineering at Georgia Tech, Yonatan joined UW-Madison as an assistant professor in industrial and systems engineering in 2020.
In his research, he focuses on applied machine learning and automated decision-making to human contexts, notably in personalized health care.
Increasingly, he’s exploring the sociotechnical implications of machine learning algorithms and has done work in areas that include safety, fairness, accountability, and transparency in automated decision-making.
His research recently won Best Poster at NeurIPS’s workshop on AI for Social Good.
He’s here today to talk more about artificial intelligence systems and the ways in which they should safely and ethically intersect with real people’s lives.
Yonatan, welcome.
[audience applauding] – All right; thanks Will, for the introduction.
Also, thanks, GPT-4 for the embellishments.
Hopefully some of that will be true in a couple years.
So as Will mentioned, my background is in engineering, my appointment’s in the engineering department.
I’m not an ethicist, although I guess I’m playing one on TV at the moment.
I’m not a philosopher.
Really, I come into this from the perspective of an engineer, someone who designs systems.
And my focus has mainly been on looking at these various applications in health care and how AI touches humans.
My students work on things from personalized weight loss to figuring out how to personalize mobile interventions, how to come up with dosing levels in ICUs for patients.
But what often comes up is we think about these very serious contexts where the stakes are high if we make good or bad decisions, is what’s the fallout from this, right?
What are the externalities that we’re not accounting for?
As we design a system that might be ethically unsavory or cause some issues down the line?
So I wanted to take an example from some of my own kind of recent research first to kind of walk you through where we see this kind of thing happening.
So this is work that I’ve been doing with my PhD student and one other, a collaborator in India and a collaborator here at UW-Madison, looking at optimizing interventions for diabetes treatment.
So diabetes is a very serious issue.
It’s in particular a serious issue in India and other lower middle income countries, where the vast proportion of undiagnosed cases of diabetes are present, and the real problem is that their health care systems don’t necessarily have the capacity to actually be able to process all these patients and give them the treatment that they need.
So one of the solutions that has been proposed is coming up, training, essentially, what are called community health care workers.
So individuals from the community, they know the culture, they know the particular challenges in the area, give them the tools they need to go into the field.
It takes less time to train them than doctors, and they can screen in more patients and provide more kind of culturally-sensitive treatment to patients.
And as an operations researcher, I see this and I say, “Okay, but you only have “so many community health care workers, “the resources are still limited.
“What’s the best way to actually come up “with a screening strategy to come in “and actually treat all these patients and make sure they get enrolled into these programs?”
So we came up with our design, we had our algorithm, we tested it against a bunch of other ones.
So here, it’s a plot that shows using different methods of sending community health care workers out, how often we can ensure that the population remains under glycemic control, and we looked at this, we say, “Yeah, this is great.
“Our method is, like, very cost-efficient.
“It takes so many less resources, “it keeps, like, all these patients under control.
Like, what could be better than this?”
And then we say like, “Hold on a moment.
“Okay, let’s take a step back.
“Why don’t we look at not just the total number of patients “that we keep under glycemic control.
“Let’s look at who’s actually remaining in control.
“What’s the distribution of patients that are actually under glycemic control?”
And so this is kinda like a funky-looking plot.
This is called a violin plot.
But you should essentially think of the curves that are coming down the side here as the distribution.
So on the Y axis, we have the final fasting blood glucose level for the different patients.
And on the X are different algorithms.
The algorithm that sort of performed the best is the red plot.
The do nothing, which is essentially don’t treat anyone, is the blue plot.
And then the other two plots are other competing algorithms that we considered.
So lower is better ’cause we want everyone to have lower glycemic control.
But the thing that I want you to focus on is that the red plot has two lobes.
So this is what we call a bimodal distribution.
Essentially, our algorithm that was very good at coming up with personalized assignments for community health workers, was really good at identifying what patients are not gonna respond to treatment and sort of neglecting them, or what patients would take so many resources to treat and leave them behind.
So depending on the health care system model you’re operating, this might not be a behavior that you want in the way that you’re assigning your community health care workers.
This is a serious concern, right?
Well, how do you actually trade off using these limited resources to achieve the primary community aim versus well, how do we deal with patient equity and other concerns of dignity that we wanna have when we’re providing health care?
And this kind of launched into what I’m gonna talk about today more in these kind of bigger ethical discussions on how we think about safety and how we think about bias.
So when I was like originally thinking about this, I was talking to my colleague, he’s in a, was, I guess, in a sociology department and then later, kinda made his own major in AI ethics.
And something that we’re thinking about is that issues of safety and bias don’t just come from one source as we’re developing automated systems.
They really come through the entire pipeline.
The data that we use for coming up with our models may have inherent biases in it just based on the way it was collected and also because history, right, in itself has bias depending on who’s doing the data collection, what were they collecting about.
Data didn’t just appear in a vacuum.
But also, models themselves can have bias.
In general, right?
Models are, the ones we use today are black box and complex.
Engineers make different choices about what features are gonna be included in models, what the thresholds are that models are gonna be using, what are the metrics that models will have that themselves make implicit assumptions about the world that then impact how they make predictions.
And of course, engineers themselves are biased, right?
We all come with our own particular histories and our own perspectives.
Something that I might think would be a perfectly viable system, if I present it to a colleague, they might tell me “This is nonsense.
You didn’t account for X, Y, Z,” right?
Or often in my work in applied healthcare, I go to a doctor like, “Look at my amazing model,” and they tell me, “Yeah, but, like, “no hospital would ever deploy this.
You need to fix this, fix that.”
I’m like, “Okay.”
So all these different challenges, even pre, let’s say 2022, were prevalent.
But with the advent of generative AI, things have sort of spiraled a bit more, I would say.
As technologies become more available for people to interact with AI and use it.
So I wanna actually look at the example that I saw that sort of started lights flashing in my head.
And this is very recent, from a few weeks ago.
So paper was published, or not published, I should say, it’s still a preprint, but I’m assuming this will be published very soon, on the use of GPT-4 for diagnosing particular cases for a disease.
So what the researchers did, instead of the traditional method of how we would do machine learning where we take a bunch of data from a table and spend time doing statistical algorithms to figure out what the prediction are that are good or bad, they took the case descriptions along with pictures and they fed them to GPT-4, and they asked it to produce a score of how likely it’s gonna be to treat the patients.
And then they compared it against a panel of expert doctors to see how well it agreed with the doctors.
[coughing] Excuse me.
And they also compared it against the actual prognosis from the real-world cases and how they ended up behaving.
So when I first, like, heard this, I was like, “Okay, this seems like a terrible way to predict this.
Like, there’s no way this is gonna work.”
Sadly, I was eating humble pie as I further read down the abstract, the researchers found that this thing achieved an AUC of over 0.9, which those of you that aren’t familiar with data science, AUC, think of it as, like, a measure of predictive accuracy in a model.
It means it essentially was able to gain as much knowledge as the experts with only variations coming from random chance.
This is kinda crazy to me that a model that is only trained on reproducing human language was actually able to come up with these predictions fairly accurately, right?
I would think you’d need a team of data scientists and time talking with doctors in order to come up with the same kind of recommendation.
Where it gets a little bit more interesting, though, for the researchers in the room, right?
This is the abstract-level result.
We all know the real science happens in the supplementary materials.
[audience laughing] So when you dig into the charts of the supplementary material, they presented this.
So here we have the different levels of accuracy achieved by the model.
And really, what they did was not use a single prediction from GPT-4.
They asked it the same case five times and then they averaged the score from the five times they asked it.
So they’re giving it a little bit of a leg up over a doctor.
Though I guess some doctors in the audience, I don’t know if you’d give your own second opinion when asked by a patient, but what’s interesting here is I want you to look at the two bars on the end, or I guess the four bars on the end.
One column says “no temperature” and one column says “no prompt.”
So temperature is a measure of how random the responses are that GPT gives, like how creative it could be with the responses.
And setting that value to zero actually made it give worse diagnostic predictions than letting that be a higher value.
And the other thing here is the no narrative.
So in some of the responses, they started the prompt for GPT-4 with, “Suppose you’re a well-renowned surgeon.
How would you address this medical case?”
And with these prompts, they didn’t give it that initial narrative description; they just said, “Look at this medical case; tell me what you think.”
And simply including that “as if you are a world-renowned surgeon, respond to the cases,” that causes a massive increase in the accuracy of the model.
So there’s a lot that’s going on here.
There’s a lot that’s happening in the black box.
The cases where GPT disagreed with the doctors mostly fit under kind of two areas.
One which is more hopeful, one which is less so.
So the more hopeful one, generally disagreements between the GPT prediction and the doctors was based off of ethical considerations.
So if there are decisions to treat or not to treat based on life expectancy, GPT tended to err more on treating versus doctors tend to be more conservative with palliative care.
So there was also some disagreements on protocol.
But in a few cases, they asked GPT to produce its own rationale for why it’s making the predictions.
And at least five different cases, the doctors read the description and said, “Yeah, this is a better reasoning than what I could come up with.”
Now, the scary thing here is that’s not how GPT came up with the predictions.
GPT is not reading the case files and analyzing and there’s no deductive logic that’s actually happening underneath there.
It’s producing based on case files that were already put in there by OpenAI what the most likely explanation that would be coming up after it gave a particular prognosis.
So this raises a bunch of questions on, as these tools become widely available, and I should note, the team that worked on this project, they weren’t data scientists.
These were all, I believe most of them are MDs and some of them are medical researchers.
As these tools become more democratic and more widely available, what are the safeguards that we put on here?
How do we actually evaluate the value of these predictions and how we can use these as true decision tools?
And where is the level of comfort that we’re willing to live with to actually have these things implemented in practice, ’cause they’re here, right?
It’s a matter of how do we manage the implementation?
Okay?
So I want you to keep this in mind.
Now, this kind of often starts, and I’m sure there’s a few people in the audience saying, “Well, this is simple, right?
“Like, all we have to do, come up with the guidelines, make AI safe, and we’re done.”
But I wanna illustrate a little bit of why that might be a little bit more challenging than that.
So we’ll make this a tiny bit interactive.
If you’re eating, keep eating, but for everyone else, play along.
So take a look at this example.
So this is a famous AI take on a trolley problem, but instead of a trolley, now we have an AV, okay?
So if you are the one programming the control for the AV, you’re gonna have two choices in the straw man scenario.
You can either have it protect the life of the driver at the expense of the lives of pedestrians, or you can have it protect the lives of pedestrians at the expense of the life of the driver, right?
So in this case, that’d be the driver going into that division.
So let’s call the scenario of protecting the life of the driver scenario A and the one protecting the lives of the pedestrians scenario B.
So how many folks would be more comfortable with scenario A in their AV?
How many folks would be comfortable more with scenario B?
Okay, so for those that are more comfortable with scenario B, I just want you to think next time you step into a Waymo and there’s a cat, I can’t swerve, it has to swerve outta the way, it’s gonna hit a tree and send you into the hospital, right?
This isn’t a easy choice.
This is not an easy decision, right?
And depending on the way we lean, that tells you a lot about kind of the, not just the technology that you wanna produce, but also the world that we’re trying to get at.
And the reason this becomes really hard is this issue, and I’m gonna steal a little bit from philosophy here.
So philosophers in the audience don’t, you know, crucify me too hard.
But this idea of vagueness.
And it’s perfectly illustrated by this thing called the Sorites paradox.
But the way it goes, suppose we have a heap of sand.
At what point, if I start removing one grain of sand at a time, does the heap cease being a heap of sand?
Does it at some point become a pile of sand instead of being a heap?
Right?
And the reason it’s vague is it’s not clear exactly how many grains of sand I need to remove before a heap stops being a heap, and this has, you know, launched thousands of years of debate about how this goes.
But I’m gonna kind of return to this big thought experiment and then kinda relate things into AI to sort of explore how different ways of thinking about vagueness and resolving vagueness are gonna help us think about different issues in AI safety.
And in particular, phrasing AI safety is an issue of normative uncertainty.
Right?
Making it not just about a list of criteria, but how do we go about discovering those criteria?
How do we handle the uncertainty that exists in society in the way that we design these models in order to come up with something that we can, at some level, agree is safe?
So with this developing social technology, as things advance, it’s not simply that we can make a list.
It’s not simply that we can encode them.
It’s not that we’re just discovering these norms that already exist in society.
But once these new technologies get developed, we’re creating new norms and new acceptable conditions, and we need to be okay with the fact that that’s the new normal that we’re creating, right?
We need to somehow think about what is this new normal that we’re creating with these things.
So moreover, what I’m gonna be talking about today is not just what is the list of things that will make your AI ethical?
But what’s the process that we could use to start thinking about, as we design these systems, how can we get ’em to a state where we can think of them as ethical?
And how can we diagnose them to see if maybe something was violated and what was the transgression that was made?
Okay?
The thing I wanna impress is that, just like I stated before, much like how safety concerns don’t just come from one part of the training pipeline, the life cycle pipeline, I guess, of the automated system, concerns around vagueness are also present throughout in the way we choose the data, choose the features that we wanna extract from the data, in the way that we choose our models, how we wanna train them, what algorithms are we using, what representation are we using in the metrics that we pick to validate our models.
And also in the end, how much are we okay with the way that these models consume data from end users and the relationship in terms of privacy and physical safety?
So I’ll split the overall pipeline into sort of three parts.
And I’ll sort of dive deeper into each one of these.
First going through the design portion, talking about the actual modeling and featurization, then about the testing, so this would be kind of more of the validation, how do we know if the model’s working?
And finally deployment, once it’s out in the wild, interactions with other people and with end users.
So let’s start in the beginning stage, and that’s the stage of design.
And we’ll examine it through one particular theory of vagueness that’s called metanormativism, which comes from the school of thought called epistemicism.
So to someone that thinks about vagueness in this way, in general, they would say that vagueness isn’t a real thing.
In reality, there’s a truth of the matter to everything, and the only thing that’s lacking is information.
Had I had more information, I would be able to tell you exactly what’s happening in the world.
If you recall the Sorites paradox with the heap, I know that somewhere in the universe, there’s the ordained level at which the heap stops being a sand.
It’s at some exact level of numbers of grains.
The only reason I can’t tell you exactly when that happens is I haven’t had the tools and the experiments yet to show you when the heap stops being a heap.
But if I can collect enough data, I can tell you exactly, you know, after 10,001 grains, that’s a heap, at 10,000 grains, that’s no longer a heap of sand.
So it’s a very kinda compelling way to think about things, and this has been a very, in my field of operations research, this has been a very popular way of thinking about problems.
Generally, people that work with mathematical models of reality like this.
‘Cause this tells us, you know, “Oh, the math is right,” right?
“The only reason my math is wrong is I don’t have enough terms in my equation.”
And it’s very useful to think about this in terms of the initial design stage of AI systems.
So if we think about this, relating it back to AI design, there’s really sort of three classes of individuals that an automated system interacts with in its life cycle.
So the first is the actual designer, the engineer that makes the system.
The second is the end user, whoever deploys it into the world and the owner of the actual process.
And the third are the other people in the environment that it interacts with.
And the challenges faced by looking at things from a metanormative lens are, well, in order to make the system safe, all I need to do is figure out what the right objective is that I need to optimize, and how do I optimize it with the constraints of other in the environment?
So again, for those of you familiar with operations research, this is a very, very popular way of thinking, especially in the school of engineering.
It’s been very useful.
So essentially, everything’s kind of being loaded onto the designer here, the designer needs to make the choice of how to do the modeling, how to do the optimization, and then deploy it further.
So let’s kind of think about an example of how this might play out with a real AI system.
So suppose we’re designing an autonomous vehicle and we’re having it drive in the road.
The problem, where we might have concerns, is we wanna design some parameters of when the vehicle is gonna be able to perform maneuvers like shifting lanes or cutting off other cars.
So if it gets, like, enough data, that’s fine, but our problem is if we have two cars driving on the highway, we have our autonomous vehicle and then we have another human driver, if they’re both driving straight, it’s hard for me to know exactly how the other driver’s gonna react if I start making a maneuver by trying to cut them or shift lanes.
If they’re a more aggressive driver, they might, you know, try to accelerate.
If they’re a more defensive driver, they might decelerate and I’ll be able to execute my maneuver.
So an epistemicist approach would say, put this into the AI’s behavior.
Let the AI explore what the other user in their environment is doing by giving it the ability to sort of probe what the people are doing in the environment and then react accordingly.
So here we go; here’s, like, the illustration of the scenario.
The white car is gonna be our human driver.
The orange car is gonna be the AV, autonomous vehicle.
And in order to begin the probing, the AV is gonna start making its move to shift lanes.
If it sees that the other person’s decelerating and more defensive, it’s gonna say “Okay, I can safely execute the maneuver,” and then pull ahead.
Alternatively, if as it starts making a shift it sees that the other person’s accelerating, it can disengage and say, “All right, I’m not gonna make the maneuver anymore,” shift back into its lane and let the other person drive by.
So fairly reasonable approach here in terms of doing this.
But there might be some limitations if we take this out of the realm of just the pure design of how we want the model to interact with the world.
And maybe some of you are thinking, “Well, hold on.
“Probing is, might be great with something like a car, but what about a softer target?”
Am I gonna probe whether a bunch of pedestrians can sprint across the road to see if I can safely run an orange light?
Maybe not a great thing to implement in the car, right?
So there’s some limitations here.
It’s a very useful lens for thinking about some particular aspects of the design, but it might not be the most useful for all aspects of the design, right?
Even if I could specify all the constraints, there’s still some unknown unknowns out there that I need to account for, that I need to think about maybe differently in order to ensure my system is behaving safely.
Okay?
So let’s move on from the design stage and let’s talk more about testing and figuring out, you know, how the system’s behaving.
So the lens we’re gonna use to understand how to kind of test and validate our system is something we call semantic indeterminism and sort of results in the thing called threshold semantics.
So someone who approaches vagueness from the perspective of semantics says, “I don’t know if there’s a truth of the matter “to the vagueness, but what I do know is is society exists “and we could all come to an agreement “on when a heap stops being a heap.
“If everyone in this room, like, we come together and we say, “‘You know what?
“‘10,000, that seems a bit much.
“‘But 999,000 grains of sand, that’s the right level.
“Past that, it’s a heap,’ that’s good enough for me.
“I don’t know if there’s a truth of the matter, “but if there’s society consensus, that’s what I’m gonna go with.”
And this way of viewing things has kind of been adopted by a segment of the machine learning community that is concerned with fairness, accountability, and transparency.
Where we look at, you know, how a model performs, not just in terms of accuracy, but we’ve devised different measures for seeing, you know, does it cause disparate treatments?
Does it cause different level of prediction depending on protected groups?
Is it more likely to overpredict negative characteristics in underrepresented groups?
How does this affect things in terms of fairness?
And again, there’s a rich literature behind this.
I’ve published papers arguing about these different measures.
They’ve been very useful in implementation and practice.
But kinda like all ways of thinking, they too, you know, have their limitations.
So suppose I come to you and I say, “Look, [claps] I’ve devised a system “that can help us solve world hunger.
“It can help restructure our Social Security crisis.
“Not only that, it could do that “while being the most fair system.
“It doesn’t discriminate based on “any protected characteristics.
It’s completely egalitarian in how it treats the world.”
Sounds good, right?
Everyone’s, like, really timid ’cause they know I’m like, about to flip it.
[audience laughing] But at least on, like, the face of it, right?
Like, solving world hunger is a good thing, right?
We can agree on that, right?
But okay, fine.
All right, everybody too good for my setup here.
Okay, so here’s the system.
Simple, we’re gonna design [laughs] the method.
We’ll have swarms of drones go out there, pick up the elderly, turn them into Soylent Green, and feed them to the hungry of the world.
It doesn’t discriminate on any protected characteristics.
It will go out there, will gather everyone.
Just because we have a bunch of metrics that tell us that a system is fair, just because we have a bunch of metrics that tell us that the system is performing properly, right?
Almost similar to the diabetes example I showed earlier, doesn’t mean the system should have been designed that way in the first place or that it was designed with the right intention in mind of solving the problem it was set out to do.
So there’s some limitations to the semantic indeterminism.
Okay.
This is, like, a very cartoonish example, I’m gonna admit, but there is real-world precedence for similar thing.
Not mulching old people, but, like, similar precedence of issues like this.
So this is an example from Flint, Michigan.
I’m sure many of you know from a couple years ago, there’s a serious issue with leaded pipes in Flint causing water poisoning.
So there’s actually a team of engineers from Georgia Tech and the University of Michigan, they got together and they came up with a very clever algorithm that was able to detect the areas where the leaded pipes were.
Based on sort of, like, the history of the area and when it was less developed, they could then adaptively kind of figure out where the lead pipes were more and more likely to be and excavate out those lead pipes.
At a certain point, this program got, like, very, very successful.
And people started looking at all these lead pipes being removed, and they went to the mayor and said, “Well, mayor, I’m really bothered by the fact “that my neighbor’s yard had a lead pipe and that was dug out, “but no one’s excavated my yard.
“For some reason, no one’s come to take the pipes out of my yard.”
And it was enough of a ruckus that instead of running the algorithm, the mayor said, “You know what?
“We need to make this fair “with respect to the other constituents.
“It can’t just excavate in particular areas.
“We need to make sure that other neighborhoods also receive the treatment.”
[claps] So…
The red dots in this map indicate areas where lead pipes were found during the excavations.
The areas of the map that are inside the orange squares, or red squares are the areas that were excavated after the change was made to make it more fair with respect to the constituents.
So even though we made the system more egalitarian, we sort of lost sight of why it was implemented in the first place.
As soon as this was put into place, we started getting a severely lower hit rate of the actual leaded pipes, and the marginal benefit of actually excavating these additional pipes wasn’t useful at all other than making the voters feel, I guess, a little bit happier that there were people digging in their street.
But this is kind of another important thing to think about, right?
Just because we have an understanding of what’s fair and measures of what we think is fair doesn’t mean we should be distracted from how and why we’re designing the system in the first place.
What is the actual problem that’s trying to be solved?
And this is sort of the limitation of only thinking in this way.
All right, so the last piece of the puzzle here that I’m gonna discuss is thinking about the final point of the life cycle, implementation with other real-world people.
And this, I’m gonna think about from the lens of value parity.
And it’s often attributed to a school of thought called ontic indeterminism.
So someone that thinks of vagueness in these terms would say, “Not only do I not care if there’s a truth “to the matter of whether there’s a heap of sand “or it’s not a heap of sand, it also doesn’t really matter “that we as a society agree when the heap stops being a heap.
“At some point, “I end up with two similar-size piles of sand.
“And I have to say, ‘You know what?
“‘These are basically both heaps.
“‘I can’t really differentiate between them.
“‘They’re on a par.
“‘And the only way for me to resolve this is to kind of “‘have to choose which one of these is the heap and which one of these is not a heap.'”
So value parity sort of happens a lot, right?
We encounter this a lot in our daily lives.
The example we usually give, right?
To my own PhD students is, right?
Like, you can either go into academia or you can go into industry.
You’ll have a successful life either way.
These two things are on a par.
It’s hard to decide which one’s better, right?
Some things are not on a par, right?
Like, do you wanna be a Green Bay Packers fan or a Bears fan, right?
Like, we all know the answer to that one.
That’s a much, much easier problem to resolve.
So when we have things that are fairly similar that we can compare them, life is great.
But oftentimes, we’re in situations where it’s not obvious what the right choice is, and even after we go through the whole pros and cons list, things still end up being basically of the same value.
So let’s use the kind of classic example for this.
So I’m gonna give a scenario that might be related to some of our mornings, maybe not, but you know, go, come with me on a journey.
You wake up in the morning, you realize you’re coming to this talk.
There’s only a couple minutes to get breakfast.
You have two choices in front of you in your kitchen.
Can either have the banana or you can have the donut.
What do you have for breakfast?
The banana or the donut?
With this audience, I’m sure everyone will go, “Oh, a banana, like, obviously.”
[audience laughing] So, okay for me, I’m weaker-willed, okay?
You know, maybe it’s a Greenbush donut, right?
It could be really good.
[audience laughing] You know, the banana is good for me long-term.
It’s gonna probably be better for me, you know, in terms of my weight; my collaborators here for weight loss are gonna be so mad when they see this.
You know, banana is better long-term, better for me, you know, from glycemic control and everything.
But donuts are great, right?
Like, I’m gonna feel good.
I’m gonna get a big boost of energy.
Probably gonna feel terrible afterwards.
But, you know, the experience between these two things, hard to judge, right?
Is it my long-term benefit and my health with a banana or my short-term satisfaction of getting the donut?
So the technical term for this kind of choice is called a hard choice.
[audience laughing] I’m serious.
[audience laughing] It’s called a hard choice.
[audience laughing] An easy choice is one where things aren’t on a par ’cause then there’s an obvious thing to choose, right?
You know, do I get, you know, the insurance policy with more coverage or less coverage if they both have the same premium, right?
Like, it’s a very easy choice to make.
But when two things are on a par, that’s a hard choice.
And the reason it’s hard is because when these two things are basically equivalent to each other, by choosing one over the other, I’m sort of committing myself to the world that I wanna see, right?
The ethics that I want to have in the world.
By choosing the banana, I am making, like, a statement about who I am as a person and kind of what I hold.
Okay, so donuts and bananas are great.
What does it have to do with artificial intelligence?
So going back, there’s a lot of design choices that artificial intelligence that when you bring teams of engineers together to discuss, it’s not gonna be obvious exactly what the best method to proceed is.
Do we value more customer satisfaction?
Do we value more predictive accuracy?
Do we value more fairness?
How do we actually reconcile all these things together?
These are hard choices.
And sort of the only remedy here is, as sort of engineers, this stuff has to be documented.
If we’re gonna have serious discussions about why systems were designed and the way they were designed, why they were implemented the way they were implemented, we need to think of this in this framework of hard choices and why these commitments were made.
Okay?
So let me go through an example here.
Thinking about another real-world case where there was a big issue of vagueness.
So this is a bit of an older use case here.
A couple years ago, Amazon released an initial version of a facial recognition tool, and the ACLU took it and they wanted to do some diagnostics.
So what they did was they took the existing model, did what’s called transfer learning, so they retrained it on a bunch of data.
The data they happened to use were the faces of individuals from the Department of Corrections.
And then they tried to predict on the faces of members of Congress to see if they could find any matches between folks in the Department of Corrections and Congress.
And what they found was that even though people of color made only 20% of Congress, they made up over 39% of the matches.
So disproportionately, members of Congress, specifically Black members of Congress and other people of color were more likely to be misidentified as individuals in the Department of Corrections that are incarcerated.
So Amazon obviously took issue with this, and they’re like, “This is, you know, kinda ridiculous.”
But when I sort of look at it, I see issues on both sides.
Right?
On the one hand, it’s vague what Amazon was actually trying to do with this model.
At the time that this thing was produced, there wasn’t actually documentation available to say when you should be able to use this, what’s a reasonable use case for this kind of openly available model?
Right?
Later on after this happened, they did add a page to the manual that says, like, “These are the predictive thresholds and you probably shouldn’t do this to do sensitive facial prediction.”
But the other thing is the ACLU wasn’t dumb, right?
Like, they knew what they were doing.
They knew that if they would prime this thing on pictures for members of the Department of Corrections, which are already, right?
Overwhelmingly, or disproportionately, I should say, not overwhelmingly, but disproportionately, people of color, that they would see disproportionate matches with people of color in Congress, right?
They didn’t know how bad the result they would be, but they knew that they would get this kind of result.
So they were specifically kind of trying to break the model in a very particular way here.
But the discussion is then, you know, what… Not just saying, “Okay, your model is racist,” but “What elements of the design should have we been aware of to not use it in this particular manner?”
Right?
Is it enough to say, “These are the predictive thresholds.
“You need to set them higher if you want to use it for a high-sensitive case?”
Is it enough to say, “You shouldn’t be using this for law enforcement capabilities,” right?
And who is culpable here?
If you train a model purposefully to be more racist, is it really the fault of Amazon here or is it the fault of how it was used?
So all these discussions come with these hard choices that need to be made as these models are being sent out into the public, right?
A lot of vagueness on what are the features that were actually extracted from the facial recognition that caused the issue?
A lot of vagueness around what the goal was that ACLU would do, right?
Like, who in real world would actually train a model to perform such a task, right?
The only reason you would do it is to kind of try to make a point about issues that it has with features it’s picking up.
And having these discussions kind of grounds it a little bit more and helps us think about, you know, if we were to, like, reintroduce this into the world, are there safeguards that we could have put in place or is there some documentation or something we could have added to make this more accountable and more reasonable?
So let me run down kind of the different forms of vagueness we talked about and sort of make it more concrete about how they slot in to the ways that we think about designing AI systems.
So first, vagueness in sort of feature design, right?
And this is sort of the coming at it from the more metanormative, right?
Or epistemicist way of thinking.
There’s two sort of trade-offs that we’re making.
We kind of abuse the terms here a little bit, where one’s thinking about things more model-free and now the other more model-based.
So model-free is making choices about what are the features and labels that we say are reasonable for the problem we’re trying to predict?
What are we gonna use to make the prediction?
What is the label we’re trying to predict?
Model-based is we’re thinking about is there some expert knowledge that we can leverage in the development of the model?
And how much of that expert knowledge do we want to use, right?
There could be institutional bias that might make their predictions wrong.
How do we wanna leverage that trade-off, right?
And in the end, we have to use this idea of context discernment that we saw, thinking about the resolution and metanormativism of figuring out what the right mix of those two variables are as we do our design.
Next, vagueness and margin in training, right?
And this is a trade-off between verification and validation.
With verification asking if we actually built the right system.
Did we try, did we design a solution to the problem that we said we were gonna solve?
We said we’re gonna solve world hunger.
Instead, we designed a genocide machine, right?
Is this really what we were trying to design here?
Validation, right, is the complement, right?
How does it perform in terms of all these other externalities, these measures of fairness, these other measures that we want it to perform against?
And we already saw that there’s a tension between these two things in how we think about design, right?
Here, the solution we come is team consensus, right?
So this idea of semantic indeterminism coming to an agreement on okay, we have to settle what the right mix of these two things are.
And finally, in terms of deployment, right?
Thinking about it from the ontic indeterminism side, a trade off of exit and voice.
So the concepts of exit and voice are kind of key things that have been talked about in understanding sort of democracy and kind of democratic systems.
And in the context of AI, we can think of exit as the right of the users to disengage from the system.
How able are they to remove themselves from interactions?
Versus voice is the ability to express dissent and concern.
How can I interact and say the system is not performing well?
So to give you an example of when this might be a little bit tricky, right?
You might say with something like GPT, I don’t have to log on to the OpenAI website, I don’t have to interact with it, but earlier, I’m gonna put you on the spot, Will, right?
Will subjected you to the background that GPT wrote about me; you didn’t have a choice.
I guess you could have walked outta the room, right?
But past that, you didn’t really have a choice to not interact with GPT today.
So that limits your exit, right?
As I use these tools, that leads the considerations of how do we wanna regulate these things and how we want to think about ensuring the right to exit?
So between these two things, this really is where sort of the democracy aspect comes in.
You need some form of public accountability.
Some form of accreditation or some form of regulation that allows us to express dissent about how these systems are designed and the rights that we have to interact with them.
Okay?
So this is sort of how it kind of fits overall.
And, again, what I wanna leave you with is that there is no set of criteria that will make AI safe.
What there is is a process that we can think about how we adapt to it as we have new values and we see how this, the potential of the technology and how it interacts with us, and that it’s key that at every point, we express dissent and figure out what we are actually comfortable with the world we’re creating as we’re designing this system.
So I’ll end it here and move on to the Q&A.
So thank you very much.
[audience applauding]
Search University Place Episodes
Related Stories from PBS Wisconsin's Blog

Donate to sign up. Activate and sign in to Passport. It's that easy to help PBS Wisconsin serve your community through media that educates, inspires, and entertains.
Make your membership gift today
Only for new users: Activate Passport using your code or email address
Already a member?
Look up my account
Need some help? Go to FAQ or visit PBS Passport Help
Need help accessing PBS Wisconsin anywhere?
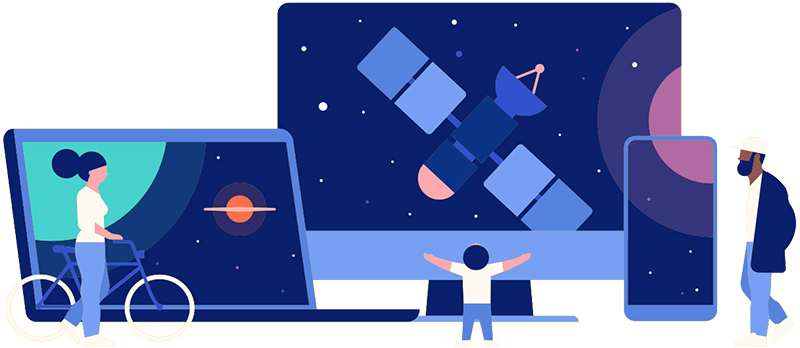
Online Access | Platform & Device Access | Cable or Satellite Access | Over-The-Air Access
Visit Access Guide
Need help accessing PBS Wisconsin anywhere?
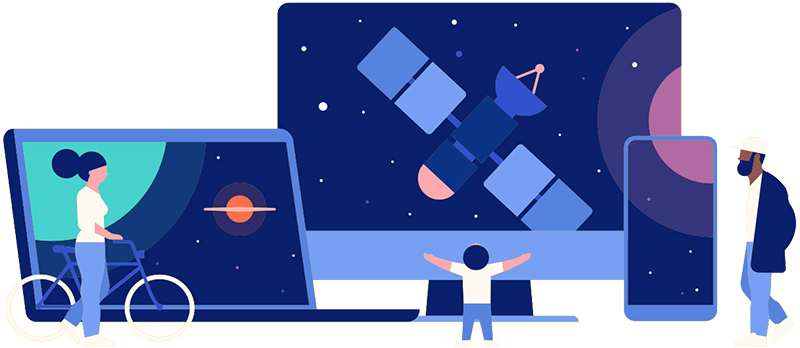
Visit Our
Live TV Access Guide
Online AccessPlatform & Device Access
Cable or Satellite Access
Over-The-Air Access
Visit Access Guide
Follow Us